Data Blending Tools: An In-Depth Exploration
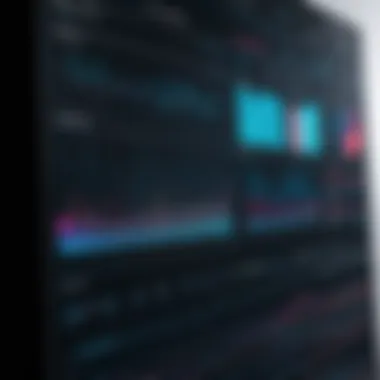
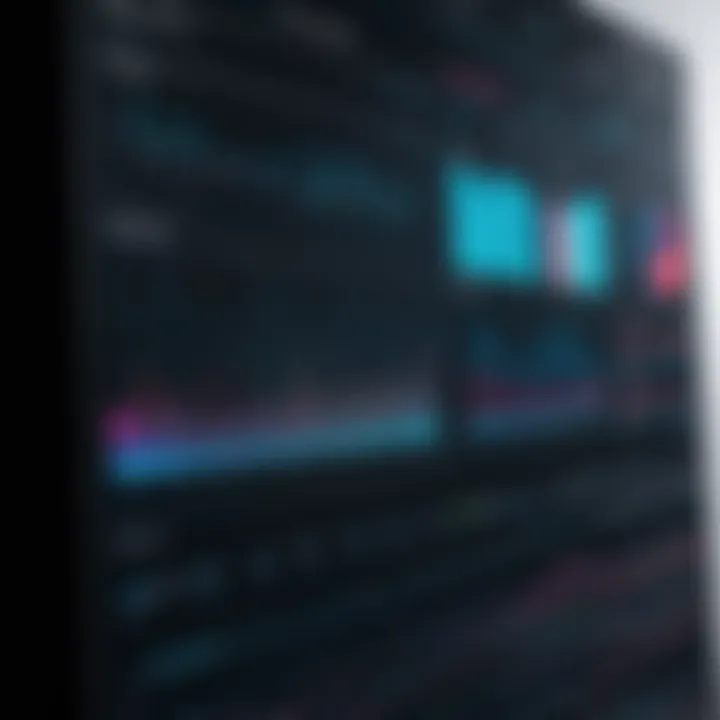
Intro
In todayβs data-centric world, organizations are inundated with vast amounts of information across various platforms. Analyzing this data effectively can offer critical insights. This necessity gives rise to data blending tools, which play a vital role in accumulating and integrating data from multiple sources. Understanding their functions, advantages, and implementations is crucial for IT and software professionals.
Data blending allows users to unite information from different origins, whether they are structured data sets or unstructured data flows. This means users can conduct more comprehensive analyses without needing complex data warehousing solutions or IT interventions. This article aims to illuminate the significance of data blending tools and provide an informed perspective for organizations considering these solutions.
This discussion will cover various aspects of data blending, including an overview of notable software options and an analysis of their strengths and weaknesses. Such insights will empower organizations to make well-informed decisions tailored to their specific needs.
Prologue to Data Blending Tools
Data blending tools play an essential role in the realm of data analytics. They offer a unique capability to combine data from various sources, creating a more comprehensive view that enhances decision-making processes. Understanding these tools is key for any IT or software professional looking to leverage data for strategic advantage. The importance of these tools extends beyond mere functionality; they empower organizations to harness diverse datasets, driving insights that are otherwise obscured when data is analyzed in isolation.
Definition of Data Blending
Data blending refers to the process of integrating data from multiple sources into a unified view. This operation goes beyond simple data aggregation, as it involves aligning and transforming different data types and structures. Commonly, organizations utilize data blending tools to merge internal data from databases with external data sources like social media, web analytics, or third-party APIs. Such processes allow for enriched analysis and provide deeper insights into business operations and consumer behavior.
Importance in Data Analytics
The significance of data blending in data analytics cannot be overstated. Firstly, it enables a holistic approach to data analysis, allowing analysts to see patterns that would not be visible when working with singular datasets. Organizations can identify trends across varying data points, which assists in forecasting and strategic planning.
Moreover, by using data blending tools, businesses can enhance data quality. Blending supports the reconciliation of data discrepancies that arise from using multiple sources, thus improving overall accuracy in reporting. With precise analytics, companies can optimize their operations and make informed decisions.
Additionally, the agility offered by data blending tools is vital. In a fast-paced business environment, having the ability to quickly integrate and analyze data from multiple sources provides a competitive edge. Organizations can adapt strategies based on real-time insights, leading to improved performance.
Key Features of Data Blending Tools
Understanding the key features of data blending tools is essential for IT professionals and businesses seeking to enhance their data analytics capabilities. These tools enable the integration of data from multiple sources, transforming it into a format that is easy to analyze. Not only do they save time, but they also enable deeper insights by combining disparate data sets. The following sections will delve into three critical features: integration capabilities, user interface and usability, and data transformation functions.
Integration Capabilities
Integration capabilities are a cornerstone of effective data blending tools. The ability to connect to various data sources is vital. For example, organizations often use databases like MySQL, cloud services like Google Drive, or applications like Salesforce. A powerful data blending tool must support diverse sources, allowing users to gather, merge, and analyze data seamlessly.
Furthermore, integration involves more than just connecting sources. It requires compatibility with various data formats, including structured and unstructured data. This is particularly important to ensure that all relevant data can be harnessed for analysis. Smooth integration minimizes the time spent on data preparation, enabling faster and more efficient insights. Ultimately, strong integration capabilities lead to better business decisions, driven by a comprehensive view of the data landscape.
User Interface and Usability
A user-friendly interface is crucial for maximizing the effectiveness of data blending tools. The design should enable users, regardless of their technical skill level, to navigate and utilize the tool successfully. An intuitive layout with easily accessible features encourages users to engage fully with the software.
Several aspects contribute to usability:
- Drag-and-drop functionality: Users should be able to easily manipulate datasets without extensive training.
- Visualization options: Good tools often offer built-in visualizations to help users quickly understand the data context.
- Guided workflows: Step-by-step processes can simplify tasks and reduce the learning curve.
In addition, support resources can significantly enhance usability. Providing tutorials and responsive customer support can help users overcome challenges quickly. Overall, a well-designed user interface contributes to faster adoption and better outcomes in data analysis.

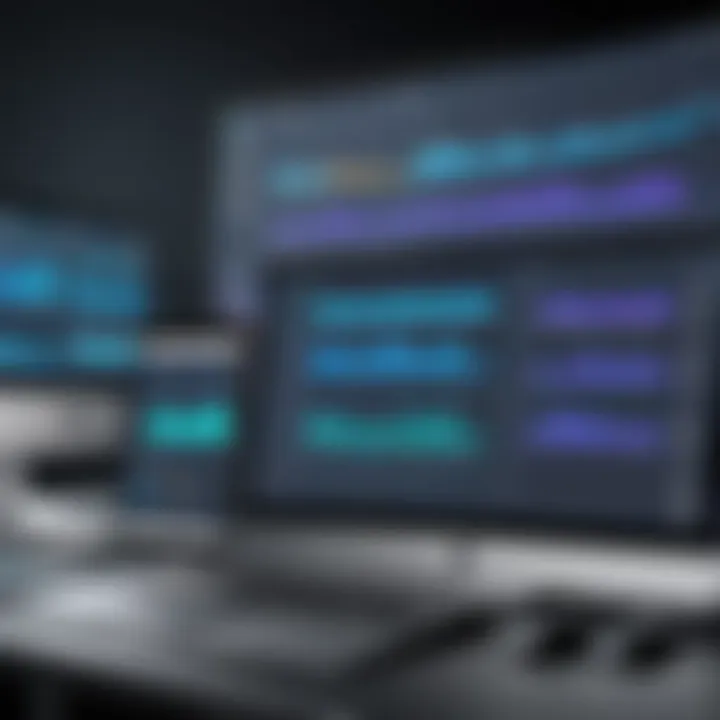
Data Transformation Functions
Data transformation functions are integral to the utility of data blending tools. Once data is collected, it often requires manipulation to ensure it fits analytical needs. Data transformation functions allow users to refine their data through various processes, such as:
- Filtering: Removing irrelevant data to focus on what truly matters.
- Aggregating: Summarizing data to create higher-level insights.
- Joining: Merging different datasets based on common fields.
These functions enable users to prepare data for detailed analysis. Furthermore, well-developed transformation features automate repetitive tasks, reducing human error and saving valuable time. The efficiency gained from utilizing robust transformation capabilities enhances the overall productivity of data analysis teams.
In summary, the key features of integration capabilities, user interface and usability, and data transformation functions are essential for effective data blending tools. They empower organizations to make better data-driven decisions and maintain a competitive edge in their industries.
Popular Data Blending Tools in the Market
The realm of data blending tools is vast and diverse. It is essential for organizations to identify suitable tools that best fit their analytical needs. With many options available, understanding what each tool offers can dramatically impact the efficiency and effectiveness of data analysis processes. Popular data blending tools provide unique advantages that cater to varying business requirements.
In this section, we explore some of the leading options available today. By evaluating their features and functionalities, users can make sound decisions that align with their data strategies.
Overview of Leading Tools
Several data blending tools have established themselves as frontrunners in the market. Among these, tools like Tableau, Alteryx, and Microsoft Power BI stand out due to their robust capabilities and user-friendly interfaces.
- Tableau: This tool is known for its excellent visualization capabilities. Users can effortlessly blend data from different sources and create visually appealing dashboards. Its intuitive drag-and-drop interface allows users to focus on analyzing data rather than wrestling with complex coding.
- Alteryx: Alteryx is favored for its data preparation and blending abilities. It supports a variety of data types, enabling users to blend structured and unstructured data efficiently. Moreover, it offers advanced analytics and predictive modeling features, making it suitable for users looking to delve deeper into their data.
- Microsoft Power BI: Power BI integrates well with various Microsoft products and is designed for ease of use. Its ability to connect to numerous data sources makes it a valuable asset for businesses already using Microsoft tools. Additionally, its real-time data blending capabilities enhance decision-making processes.
These tools have gained traction not only for their functionalities but also for the communities built around them. Dedicated user forums and extensive documentation often accompany these products, assisting users in resolving challenges and maximizing their use of the tools.
Comparison of Top Data Blending Solutions
When choosing a data blending tool, users must consider various factors that differentiate one solution from another. Evaluating features such as integration capabilities, supported data types, and analytics functionalities is crucial.
Hereβs a brief comparison to highlight key aspects:
| Tool | Integration | Data Types Supported | Advanced Analytics | User Experience | | Tableau | Excellent | Structured, Unstructured | Limited | High | | Alteryx | Good | Structured, Unstructured | Strong | Medium | | Microsoft Power BI | Excellent | Structured | Limited | High |
Integration: Tableau and Power BI have exceptional integration capabilities with various third-party applications. Alteryx also integrates well but shines more in data preparation rather than visualization.
Data Types Supported: While Tableau and Power BI focus primarily on structured data, Alteryx does a commendable job of handling both structured and unstructured data, giving it an edge for diverse datasets.
Advanced Analytics: Alteryx stands out in this category with its robust predictive modeling features. Tableau and Power BI, while effective for visualization, are more limited when it comes to advanced analytics.
User Experience: Both Tableau and Microsoft Power BI are celebrated for their intuitive user interfaces. Alteryx is also user-friendly but may require more time to master due to its advanced functionalities.
Key Consideration: Different tools may suit different types of organizations based on their analytic needs, existing setups, and team expertise. It is crucial to assess which tool aligns best with specific requirements.
User Experiences with Data Blending Tools
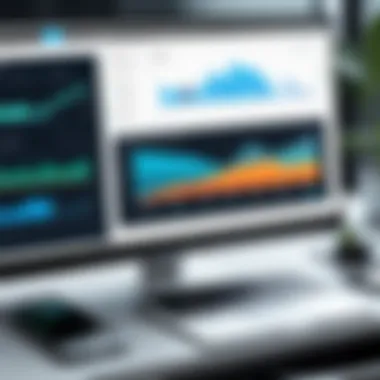
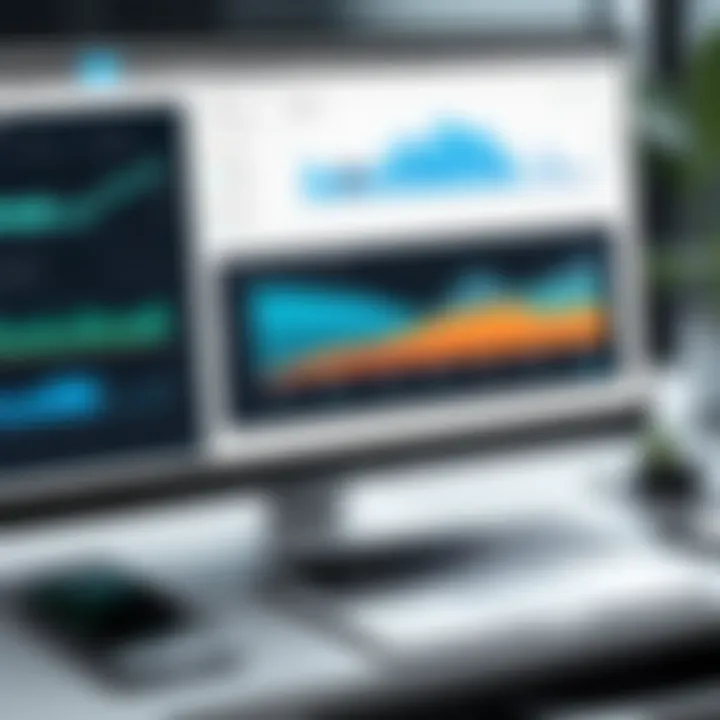
User experiences with data blending tools are crucial for understanding how these systems perform in real-world applications. Insights gathered from various users can direct IT and software professionals toward making informed choices. Evaluating experiences helps businesses uncover the practical benefits and challenges associated with different tools. User feedback often reveals the impact on productivity, data integration, and overall workflow. This section will examine case studies of successful implementation as well as common user challenges.
Case Studies of Successful Implementation
Analyzing case studies offers valuable lessons on how data blending tools can be integrated into business processes. Many organizations have successfully utilized tools like Tableau, Alteryx, and Microsoft Power BI to enhance their decision-making processes. For instance, a retail chain implemented Tableau to visualize sales data from various sources, resulting in increased revenue by 15% within six months. The visual insights allowed the management team to spot trends in inventory that they had previously overlooked.
Another compelling example includes a healthcare provider leveraging Alteryx to combine patient records from multiple databases. This integration led to improved patient care and streamlined administrative processes, as healthcare professionals could access consolidated data quickly.
These examples illustrate not only the effectiveness of these tools but also the transformational impact they can have on business operations. However, the success of implementation largely depend on proper training and ensuring that users are fully equipped to utilize the toolβs capabilities.
Common User Challenges
While there are many positive experiences associated with data blending tools, there are also common challenges that users face. Understanding these issues can help organizations prepare better for implementation.
A frequent concern among users is the complexity of initial setup. Many tools require significant configuration to blend data sources accurately. Training staff to utilize these features can be time-consuming and often leads to frustration.
- Data Quality: Another major roadblock is ensuring high-quality data flows into the blended environment. Poor data quality can lead to misleading insights.
- Integration Difficulties: Users may encounter difficulties when integrating tools with existing systems. Compatibility issues can result in data silos that hinder analytics efforts.
- Cost: Some data blending tools come with high costs which can be a burden for small and medium-sized enterprises. Ensuring return on investment through effective use becomes a major concern.
Organizations need to understand that choosing a data blending tool is not solely about features. It should also focus on user experience and support during implementation.
Addressing these challenges early can significantly improve the chances of successful data blending adoption. By focusing on user feedback and experiences, organizations can avoid common pitfalls and maximize the benefits of these powerful tools.
Best Practices for Data Blending
Data blending is a crucial practice in making data analytics more effective and insightful. Implementing the best practices in data blending ensures that quality insights are derived from which informed business decisions can be made. The following sub-sections detail effective strategies, including management of data sources, ensuring data quality, and the importance of regular monitoring and maintenance.
Effective Data Source Management
Effective data source management involves organizing and categorizing different data inputs to optimize their blending and overall analysis. It is important to know the origin, structure, and the intended use of each data source.
- Data Inventory: Keep a detailed inventory of all data sources being blended. This includes clearly labeling data sets and understanding their formats and update cycles.
- Categorization: Group data sources based on their relevance or similarity. For example, financial data can be kept separate from operational data. This helps avoid confusion when analyzing results.
- Access Control: Managing who has access to what data is vital. Ensure sensitive data is protected and only accessible to authorized personnel, reducing the risk of data leaks or misuse.
By following these steps, organizations can streamline their data blending process, which leads to more reliable insights.
Ensuring Data Quality
Data quality is foundational to effective data blending. Poor data quality can result in flawed analyses, leading to incorrect conclusions. Strategies to ensure data quality include:
- Consistency Checks: Regularly verify data consistency across different sources. Discrepancies can arise from differing data formats or entry errors.
- Validation Rules: Implement validation rules during the data entry or import stages. This may involve checks on data types, ranges, or mandatory fields.
- Data Cleaning: Routinely clean data to remove duplicates, correct inaccuracies, and handle missing values. Automated tools can assist in speeding up this process.
Ensuring high data quality will significantly enhance the reliability of blended results, thus supporting more accurate business strategies.
Regular Monitoring and Maintenance
Monitoring and maintaining data blending processes is essential to adapt to evolving data environments. Organizations must prioritize ongoing assessments of their systems to ensure efficiency, including:
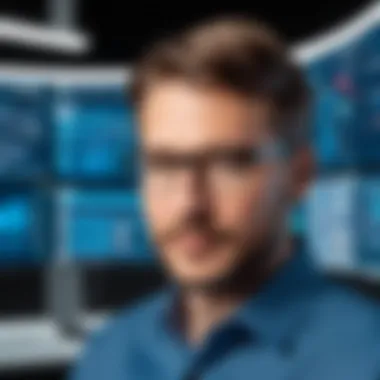
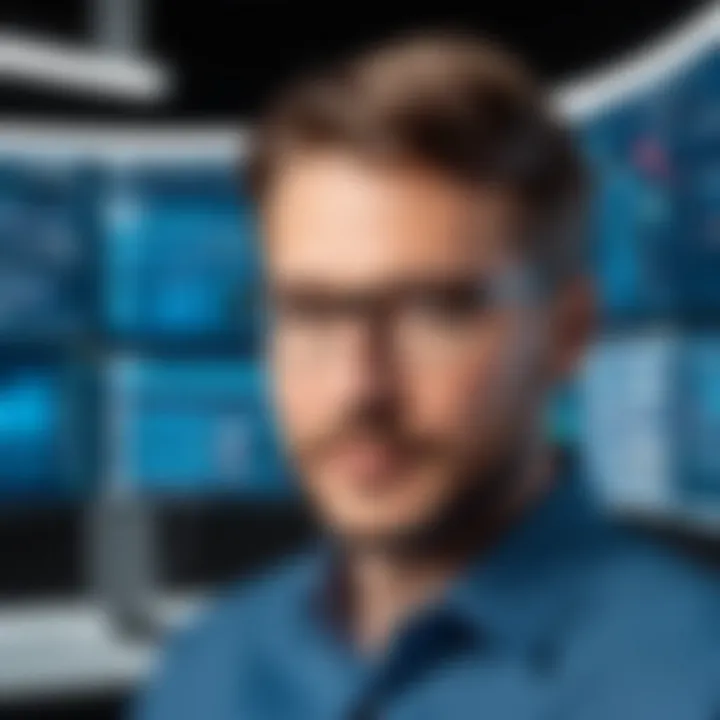
- Performance Metrics: Establish metrics to assess the efficiency of data blending activities. Monitor these metrics to identify performance trends or potential issues.
- Scheduled Audits: Conduct regular audits of data sources and blending processes. This helps to catch inconsistencies or quality declines early, preventing larger issues later on.
- Update Protocols: Keep protocols in place for updating data sources and tools. As technology evolves, so too should the methods used for data blending.
Ongoing monitoring not only enhances the integrity of the data blending process but also boosts user trust in the results generated.
In summation, these best practices provide a structured approach to data blending. By focusing on effective source management, ensuring data quality, and committing to regular maintenance, organizations can enhance their analytical capabilities and support data-driven decision making.
Future Trends in Data Blending Tools
Understanding future trends in data blending tools is vital for IT professionals and businesses striving to maintain a competitive edge in data analytics. As the field evolves, staying informed about emerging technologies and methodologies is essential. This section explores advancements that are shaping the future of data blending tools, focusing on AI, machine learning, and automation.
Advancements in AI and Machine Learning
Artificial Intelligence (AI) and machine learning are rapidly transforming how data blending tools function. These technologies enhance data blending processes, enabling tools to analyze large datasets with greater efficiency. With AI algorithms, systems can detect patterns, trends, and correlations in the data that may not be obvious to human analysts.
Key benefits of integrating AI in data blending tools include:
- Enhanced Accuracy: AI reduces human error by automating data validation processes.
- Real-Time Analytics: Businesses can access insights much quicker, allowing for timely decision-making.
- Predictive Capabilities: Machine learning allows tools to make educated predictions based on previous data trends, helping organizations to strategize efficiently.
The integration of these technologies requires careful consideration of current infrastructures. Organizations must assess whether their existing systems can implement AI functionalities. Moreover, training data must be robust to ensure the AI models learn accurately and provide reliable results.
"The integration of AI in data blending tools promises not only improved accuracy but also operational efficiency."
Emergence of Automation in Data Blending
Automation is becoming increasingly prominent in the realm of data blending. This trend leads to streamlined workflows, allowing teams to dedicate more time to strategic analysis rather than manual data preparation tasks. The benefits of automation in data blending tools include:
- Time Savings: Automating routine tasks frees up valuable resources for more complex analytics and business intelligence work.
- Consistency and Reliability: Automated systems ensure consistent data handling, which enhances reliability during analysis.
- Scalability: As business data grows, automated processes can easily scale, accommodating larger datasets without significant increases in effort or time.
Businesses considering automation must evaluate their specific needs. It is crucial to choose tools that not only integrate well with existing systems but also align with long-term data strategies. Automation should not merely replace manual tasks; it should enhance the overall effectiveness of data blending efforts.
The End
Data blending tools are pivotal in modern analytics frameworks, offering essential capabilities for aggregating and analyzing diverse data sources. This section emphasizes the significance of concluding insights derived from data blending processes and the broader implications for decision-making and strategy. Their role is not merely functional; they enhance the quality and depth of analyses, making them indispensable for both small and large businesses.
Summation of Key Insights
In the realm of data blending, several notable insights emerge:
- Cross-Source Integration: One of the primary advantages is the ability to seamlessly combine data from various origins, leading to more comprehensive insights.
- User-Friendly Interfaces: The advancement in user interface designs ensures that even users with minimal technical background can effectively harness tools without steep learning curves.
- Enhanced Data Quality and Accuracy: The blending processes involve transformation functions that ensure better data quality, leading to more reliable outcomes.
- Real-World Applications: Case studies have shown that organizations using data blending tools have marked improvements in their analytics capabilities, revealing deeper insights that drive business growth.
These aspects underscore the vital role that data blending avenues play in orchestrating a symphony of information that drives strategic decisions.
Final Recommendations
In light of the discussed insights, the following recommendations can guide organizations in optimizing their use of data blending tools:
- Evaluate Integration Needs: Before selecting a tool, evaluate the specific integration capabilities required for your data environment.
- Invest in Training: Ensure appropriate training for users to maximize the utility of these tools, bridging the gap between technological ability and business needs.
- Monitor Performance Regularly: Establish regular monitoring systems to assess the performance and impact of data blending solutions.
- Prioritize Data Security and Governance: With blending bringing together multiple data sources, it's crucial to maintain stringent security standards and data governance practices.
By focusing on these recommendations, businesses can significantly enhance their data analytics processes, leading to informed and strategic decision-making.