Harnessing Data Analytics with Splunk for Efficiency
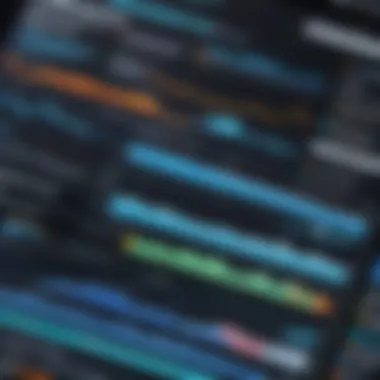
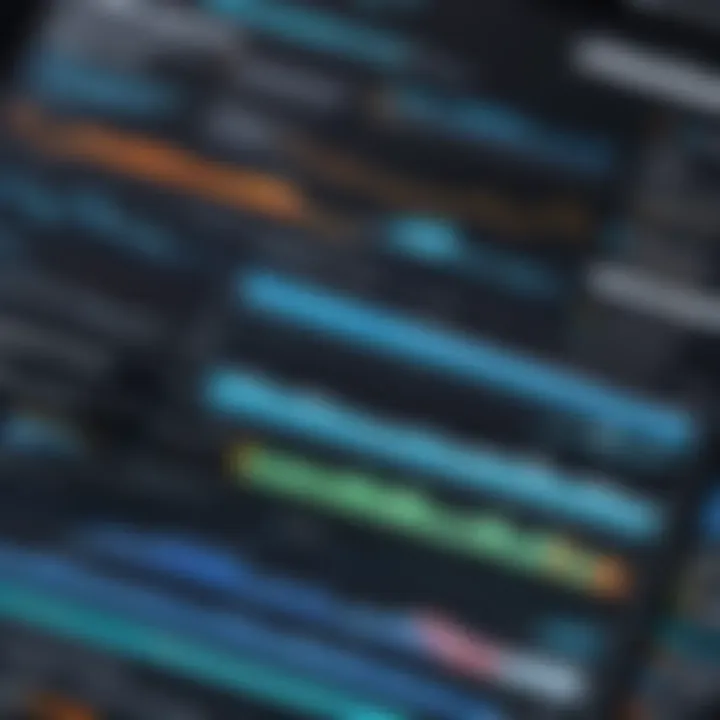
Intro
In today's fast-paced digital arena, enterprises are drowning in a sea of data. From customer interactions to operational metrics, the sheer volume is staggering. Enter Splunk, a tool that claims to harness this chaos into structured insights. The question floatin' around is: how exactly does data analytics play a role in maximizing the capabilities of Splunk? This exploration dives into its inner workings, revealing techniques, applications, and even a peek into its advantages compared to other software in the market.
Overview of Software
Description of Software
Splunk is a powerful platform designed for operational intelligence, collecting and indexing machine-generated data across an organization's infrastructure. Its charm lies in its ability to transform complex datasets into actionable insights. Think of it as a magnifying glass for your data, enabling businesses to see patterns, trends, and anomalies that might otherwise slip through the cracks.
Key Features
- Data Ingestion: Splunk accepts data from various sources—be it logs, configurations, or transactions—turning them into a searchable knowledge base.
- Powerful Search Capability: With its search processing language (SPL), users can delve deep into their data, crafting queries that yield relevant results.
- Real-Time Monitoring: Businesses can monitor their operations in real-time, ensuring that any unusual activity is spotted before it spirals.
- Analytics and Dashboards: It offers robust visualization tools to build custom dashboards, allowing users to see data at a glance.
- Alerts and Reports: Users can set thresholds that trigger alerts, alongside generating reports that summarize key insights.
Software Comparison
Comparison with Similar Software
When stackin' Splunk next to other analytics platforms like Elastic Stack or IBM QRadar, a few distinctions stand out. Elastic Stack offers a more open-source approach, while QRadar focuses heavily on security intelligence, primarily favored in cybersecurity realms. However, Splunk’s versatility makes it a broader choice for operational insights across various sectors.
Advantages and Disadvantages
- Advantages:
- Disadvantages:
- User-Friendly Interface: Even for those who aren’t data scientists, Splunk brings complexity down to an approachable level.
- Wide Range of Apps: The Splunkbase features numerous apps enhancing functionality tailored to specific needs.
- Scalability: Accommodates growing amounts of data without breaking a sweat.
- Cost Factor: It can be expensive, especially for smaller businesses looking to scale.
- Learning Curve: While its usability is commendable, mastering SPL can be daunting for new users.
"Data is like a well-structured narrative; each piece contributes to the bigger picture. Splunk just helps you tell that story more effectively."
As we unravel these details, one thing remains clear—data analytics in Splunk is not just about crunching numbers, it's about essential insights that businesses can wield to enhance performance and strategy. Whether you're a tech-savvy analyst or a business professional, understanding this dynamic relationship between Splunk and data analytics can be a game-changer in driving your organization forward.
Prolusion to Data Analytics
In an age where information is abundant, understanding the framework of data analytics serves as the linchpin for modern enterprises. The intersection of data analytics and business strategy is where significant insights emerge. This section aims to elucidate how data analytics not only aids in strategic decision-making but also enhances operational efficiency in various sectors.
When organizations harness the potential of data analytics, they open doors to vast reservoirs of insights. From improving customer experience through targeted marketing to streamlining operations, the benefits are manifold. With effective analysis, businesses can pivot based on data rather than gut feelings. This could mean the difference between staying afloat or sinking amid competition.
Definition and Importance of Data Analytics
Data analytics involves the systematic computational analysis of data, which transforms raw numbers into meaningful information. It makes sense of the chaos—turning pressure into precision. The importance of data analytics cannot be overstated; it brings clarity and focus to organizations, driving them toward data-informed decisions. Companies like Amazon and Netflix thrive because they leverage vast data sets to personalize user experiences. This not only attracts customers but also enhances retention rates.
Every facet of an organization can benefit from data analytics, be it marketing, finance, operations, or supply chain management. The ability to discern patterns and trends from collected data is invaluable. An organization that makes decisions backed by data stands a much higher chance of achieving its objectives.
Overview of Data Analytics Tools
The market is saturated with tools designed to aide in data analytics, each catering to various needs and preferences. Tools range from user-friendly platforms to sophisticated analytical software that requires a certain level of expertise. Here are some noteworthy tools:
- Tableau: Known for its powerful visual capabilities, Tableau allows users to create interactive dashboards that can transform complex data sets into digestible visual narratives.
- R and Python: These programming languages are heavyweights in the analytics domain. R is particularly useful for statistical analysis, while Python offers versatility, with libraries tailored for data manipulation.
- Google Analytics: For businesses focused on online presence, Google Analytics provides invaluable insights regarding web traffic, user behavior, and conversion rates.
- Microsoft Power BI: This tool allows businesses to visualize their data and share insights across the organization, all while embedding analytics in their apps.
These tools highlight the wide-ranging possibilities in the realm of data analytics. Choosing the right tool often hinges on specific organizational needs, resources, and the proficiency level of the potential users.
"Data is the new oil. It's valuable, but if unrefined, it cannot really be used. It has to be processed and analyzed to extract the value." - Clive Humby
In summary, the journey of data analytics is integral for businesses aiming for sustainable growth and innovation.
Understanding Splunk
When diving into the realm of data analytics within Splunk, grasping the essence of Splunk itself is paramount. Understanding Splunk isn't just a technical necessity; it forms the bedrock of leveraging the platform’s capabilities to unlock invaluable insights. Splunk serves as not merely a tool, but a comprehensive solution that addresses various aspects of data management, from collection and parsing to analysis and visualization. The benefits of using Splunk are numerous, making it a choice for organizations aiming to derive maximum value from their data.
What is Splunk?
Splunk is a powerful software platform meticulously designed to search, monitor, and analyze machine-generated big data in real-time. In simpler terms, think of it as a sophisticated librarian for data – it takes a mountain of information and organizes it so that businesses can make sense of the chaos. Have you ever tried to find a specific book in a library that contains thousands of volumes? That’s how many organizations feel when staring down massive troves of data. Splunk brings clarity, enabling businesses to transform raw data into actionable insights effortlessly.
"In the world of data, Splunk acts as a lighthouse guiding ships through fog, ensuring they reach their destination safely."
Core Features of Splunk
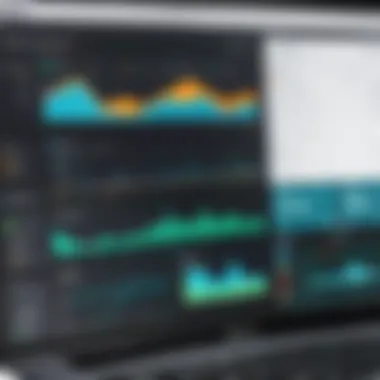
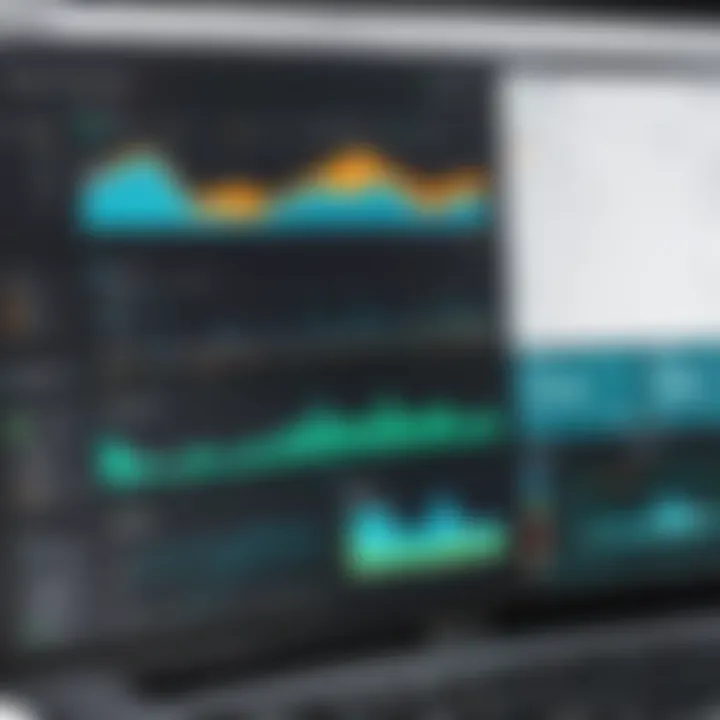
One cannot discuss Splunk without highlighting its robust core features. These tools form an essential part of its framework:
- Data Ingestion: Splunk can ingest diverse data formats seamlessly. Whether it’s logs from servers or metrics from your devices, it can bring in data from a myriad of sources.
- Search & Investigate: Its powerful search capabilities allow users to hunt through data using simple keywords, making complex queries intuitive.
- Real-Time Monitoring: Splunk offers live monitoring, enabling organizations to stay ahead of issues before they escalate. Consider it a proactive guardian of your systems.
- Alerts & Reports: Users can set up alerts that trigger when specific conditions are met, and generating reports is straightforward, providing management with ongoing insights.
- Custom Dashboards: The ability to craft personalized dashboards enables teams to visualize their data in the ways most relevant to them, adding an extra layer of comprehensibility.
These characteristics, when combined, turn Splunk into a robust data analytics tool, making it indispensable in today’s data-centric world.
Splunk's Ecosystem
Understanding Splunk also requires some awareness of its broader ecosystem. Splunk thrives not just as a standalone platform, but within an expansive framework that includes various integrations and applications that expand its capabilities.
- Add-ons and Apps: Splunk offers a wide array of apps and add-ons that extend its functionality. For instance, the Splunk Machine Learning Toolkit offers enhanced capabilities for predictive analytics.
- Community Contributions: The Splunkbase community actively supports the platform with user-generated apps and add-ons, promoting a culture of collaboration and shared learning.
- Integrations with Other Tools: Splunk can easily integrate with tools like AWS, Microsoft Azure, or even various SIEM solutions to deliver a cohesive data analytics experience.
The ecosystem around Splunk enhances its versatility, allowing organizations to tailor solutions to their unique needs. Without a clear understanding of this entire landscape, users might miss out on capabilities that can propel their analytics strategy forward.
Data Analytics Capabilities in Splunk
Data analytics capabilities in Splunk are crucial for modern organizations, especially when it comes to harnessing data for smarter decision-making. By leveraging analytics, companies can turn everyday data into actionable insights, improving efficiency and enhancing strategic planning. Splunk serves as a powerhouse in this regard, offering a spectrum of functionalities that cater to both real-time analysis and long-term strategic insights.
Data analytics in Splunk doesn't just stop at collecting data; it intelligently processes and analyzes that data to reveal patterns, trends, and correlations. This capability is vitally important because many businesses generate terabytes of data daily. Without robust analytics tools, making sense of this data can be akin to trying to find a needle in a haystack.
Real-Time Data Analysis
Real-time data analysis in Splunk is arguably one of its standout features. Organizations can monitor their operations continuously, allowing them to detect anomalies or issues as they happen. This immediate feedback loop is essential for enterprise operations where downtime can lead to significant losses, notably in sectors like finance or manufacturing.
For instance, consider a scenario where a retail business utilizes Splunk to keep an eye on their sales transactions. If there’s a sudden spike in trends indicating fraudulent activity, the system can alert the staff right away. This fast action can save the company from potentially severe financial repercussions.
Moreover, real-time analysis fosters a data-driven culture, enabling employees to rely on current data, not outdated reports, facilitating better decisions. Just like a captain needs to know the weather conditions for successful sailing, companies need real-time insights to steer their operations effectively.
Predictive Analytics Features
The predictive analytics features within Splunk can turn data from a rearview mirror into a compass guiding enterprises toward future outcomes. Through sophisticated algorithms and machine learning models, Splunk provides predictive insights that help businesses foresee potential challenges and opportunities.
Imagine a logistics company applying Splunk's predictive capabilities. By analyzing historical traffic data and delivery times, the system can forecast delays in shipments and suggest optimal delivery routes. This foresight not only enhances customer satisfaction but also reduces operational costs.
Additionally, these capabilities can be adapted to various industries. For healthcare sectors, for example, predictive analytics can help anticipate patient influx in hospitals based on past records, allowing administrators to allocate resources effectively. In a world where a stitch in time saves nine, predictive analytics is the thread that connects past behavior to future expectations.
Visualizations and Dashboards
Visualizations and dashboards play an essential role in enabling stakeholders to grasp data outcomes quickly and effortlessly. Splunk excels in providing rich visualizations that convert complex data sets into easily digestible visual formats, such as graphs and charts. This becomes especially beneficial for busy executives, who often need to make decisions at a moment's notice but may not have the bandwidth to parse through intricate data sets.
By having customizable dashboards, companies can tailor their views to extract relevant information pertinent to their specific needs. For example, a marketing team may want to track campaign performance in real-time, adjustments made by sales teams, or website traffic indicators all in one view.
Visual representations of data can often highlight trends that are not immediately apparent in raw numbers. The right dashboard can transform a mountain of data into a clear, actionable trail for decision-makers.
"The effectiveness of any data strategy lies not just in what you measure, but how effectively you visualize it."
In summary, Splunk's data analytics capabilities transform how organizations analyze and leverage their data. From real-time insights to predictive capabilities and compelling visualizations, these features are integral for guiding enterprises toward success in increasingly competitive markets.
Implementing Data Analytics with Splunk
Implementing data analytics within Splunk is not just a technical procedure; it is a crucial step in converting a wealth of raw data into actionable insights. Organizations today generate an enormous volume of data from multiple sources—from server logs to user activity across applications. Being able to harness this data effectively can lead to smarter business decisions, enhanced security measures, and improved operational efficiency.
The integral aspect of implementing data analytics with Splunk involves understanding how to configure the platform properly and utilize its advanced capabilities to maximize the value of your data. This section will break down essential components such as setting up Splunk for analytics, exploring data ingestion methods, and formulating effective queries. The goal here is not merely to understand what tools are available, but to appreciate how they interact synergistically to transform data into clear and strategic insights for your organization.
Setting Up Splunk for Analytics
Setting up Splunk for analytics requires a systematic approach to configuration and user access. The initial setup isn't just about installing the software but also about creating a conducive environment for data exploration and analysis.
- Install Splunk: Start with downloading the version fit for your system—be it on-premises or cloud-based. Splunk provides guides that outline the installation steps clearly.
- User Configuration: After installation, set up user roles and permissions. This step is critical because it ensures that sensitive data is protected while allowing analysts to access necessary information for their work.
- Connect to Data Sources: It’s essential to connect Splunk to the relevant data sources. This could involve integrating with databases, cloud services, or third-party applications where your data resides.
Remember, the easier it is to retrieve data from various sources, the better the analytics outcomes will be.
Data Ingestion Methods
Data ingestion is the lifeblood of any analysis platform—without effective data ingestion methods, all tools and queries are rendered pointless. Splunk supports several ingestion methods that provide flexibility and efficiency in bringing data into the platform.
- File Upload: You can manually upload files such as CSVs or log files directly into Splunk. This is straightforward for small sets of data but not scalable solutions for bigger datasets.
- Data Forwarders: Deploying Splunk Forwarders is one of the more robust approaches. These lightweight agents collect log and event data from servers to send to the Splunk indexers. They can operate in both real-time and batched modes. Using forwarders can significantly reduce the load on your network during peak times.
- REST API: For applications, Splunk provides REST APIs, allowing data ingestion directly from web applications. This method is vital for real-time streaming data where timeliness is crucial.
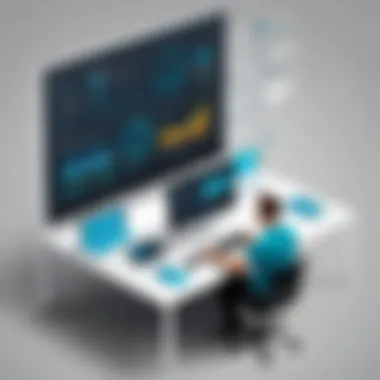
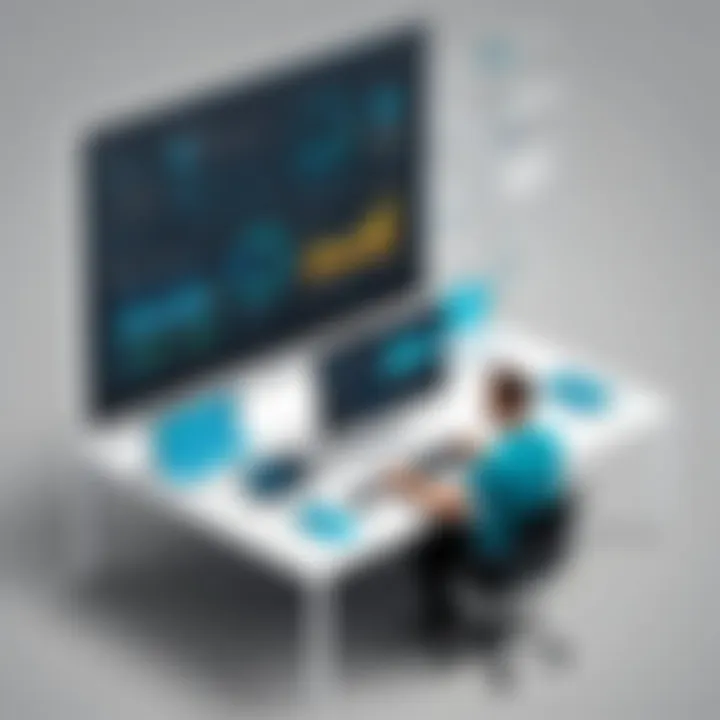
Choosing the appropriate method is often based on specific use cases and the organization’s existing infrastructure.
Creating Effective Queries
Once your data is ingested into Splunk, the next critical phase is formulating queries that extract relevant insights. Here, the Search Processing Language (SPL) becomes your best friend, providing a powerful means to sift through vast datasets.
- Understand SPL Basics: Having a solid grasp of SPL syntax is paramount. It allows you to craft queries that can find elements within datasets quickly.
- Leverage Field Extraction: Take advantage of Splunk’s ability to extract fields during index time. Things like IP addresses, user IDs, or timestamps can be vital for effective analysis.
- Utilize Search Commands Wisely: Splunk offers a range of search commands, such as for summarizing data or for calculating new fields. Using them correctly can turn a pile of raw data into insightful information in just a few clicks.
"The ability to ask the right question is more than half the battle in deriving insights from data."
The effectiveness of your queries will significantly depend on the clarity of the questions you are trying to answer, and thus, understanding the data structure plays a vital role in this process.
In summary, implementing data analytics with Splunk involves a multifaceted approach that encompasses proper setup, effective ingestion methods, and tailored queries. Each of these elements contributes to not only understanding the data being analyzed but also enhancing the decision-making process. As you build familiarity with the system and its capabilities, the results will speak for themselves.
Challenges in Data Analytics with Splunk
Navigating the world of data analytics within the Splunk platform comes with its unique set of challenges. Acknowledging these challenges doesn't merely cast a shadow on Splunk's capabilities. Rather, it sheds light on critical aspects that organizations must consider when leveraging data analytics. For IT professionals and businesses alike, understanding these hurdles is essential to optimizing their analysis efforts and fully tapping into Splunk's potential.
Common Pitfalls in Data Analysis
Data analysis, even with robust tools like Splunk, can often lead analysts down a rabbit hole of misunderstandings and inefficiencies. Some common pitfalls include:
- Over-looking Data Context: Analysts may focus on raw figures while ignoring the story behind the data. Data without context is like a map without markers; it can lead you anywhere but not necessarily in the right direction.
- Neglecting User Training: Users must grasp Splunk’s features. If they don't, insights can become half-baked, making it more of a chore than a tool.
- Assuming All Data is Relevant: Not every data point has the same level of importance. Analysts must prioritize and filter what data is vital for their objectives to avoid analysis paralysis.
By recognizing these pitfalls early on, organizations can create a culture of data literacy that fosters accurate analysis and insightful findings.
Data Quality Issues
Quality remains the bedrock on which solid analysis stands. Flawed data leads to conclusions that could misguide decision-making. With Splunk, the following data quality issues often emerge:
- Inconsistent Data Sources: Different sources often use varying formats. This inconsistency can confuse analysts, who may end up aggregating data that should not be combined.
- Incomplete Entries: Missing data points can skew results. Thus, ensuring complete and comprehensive datasets is vital for accurate analytics.
- Data Duplication: Duplicate data can inflate metrics, leading to distorted insights. Frequent checks ought to be in place to clean up any redundancies before analysis.
Addressing data quality issues like these means establishing rigorous data governance practices, which helps ensure that the analysis conducted yields relevant results.
Handling Large Datasets
Working with extensive datasets can feel akin to trying to drink from a fire hydrant—impressive in volume, yet overwhelming. Splunk can manage large datasets effectively, but certain considerations must be in mind:
- Performance Optimization: Large volumes of data can slow down queries. Strategic data indexing and partitioning enhance performance, allowing for faster queries and quicker insights.
- Resource Allocation: When dealing with vast datasets, ensuring that your hardware is scaled appropriately becomes critical. Underprovisioned systems can lead to frustrating delays and missed opportunities for real-time analysis.
- User Segmentation: Different teams may require different datasets. Segmenting data access helps users focus only on relevant information, ensuring analysis remains efficient and effective.
"Understanding the challenges that come with data analytics can transform obstacles into opportunities for improved strategies and insights."
In summary, while challenges in data analytics with Splunk are real, they can be managed through well-considered practices and strategies. By recognizing common pitfalls, prioritizing data quality, and effectively handling large datasets, organizations can unlock deeper insights and better utilize Splunk’s robust capabilities.
Case Studies of Data Analytics in Action
Exploring the case studies of data analytics in Splunk reveals not only the capabilities of the platform but also its tangible impact across various industries. It serves as a powerful testament to how organizations can leverage data to thrive in today’s data-saturated world. By understanding real-world implementations, businesses can derive critical insights that facilitate informed decision-making and improve overall operational efficiency.
Business Intelligence Applications
In the sphere of business intelligence, Splunk emerges as a vital tool for interpreting vast datasets to unearth trends and insights. For instance, several retail companies have utilized Splunk to analyze customer behavior through transaction logs and online activities. By sifting through this data, organizations can identify purchasing patterns that guide inventory management and marketing strategies.
- Targeted Marketing: By understanding customer preferences, businesses can tailor marketing efforts to suit specific demographics, ultimately maximizing conversion rates.
- Supply Chain Optimization: Data analysis helps expose bottlenecks in supply chains, allowing for timely interventions that enhance operational performance.
The impact is often visible in increased sales and enhanced customer satisfaction, making a strong case for embedding analytics into core business strategies.
Security Information and Event Management (SIEM)
Security remains a top priority as organizations increasingly face cyber threats. Splunk aids in the real-time analysis of security events, enabling IT teams to quickly address vulnerabilities before they spiral into larger issues.
An example can be drawn from a financial institution that deployed Splunk for SIEM. They monitored logs across their entire infrastructure to detect anomalies indicative of potential security breaches. Results were impressive:
- Immediate Threat Identification: Detection of unusual login attempts led to swift action, preventing unauthorized access.
- Regulatory Compliance: Maintaining a holistic view of activities helps in adhering to compliance mandates as auditors can easily verify logging practices.
Thus, employing Splunk for SIEM not only fortifies security but also fosters a culture of vigilance and preparedness in handling potential threats.
Operational Monitoring and Insights
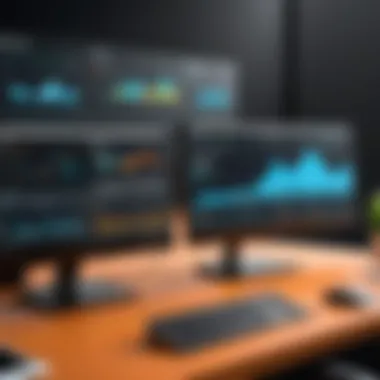
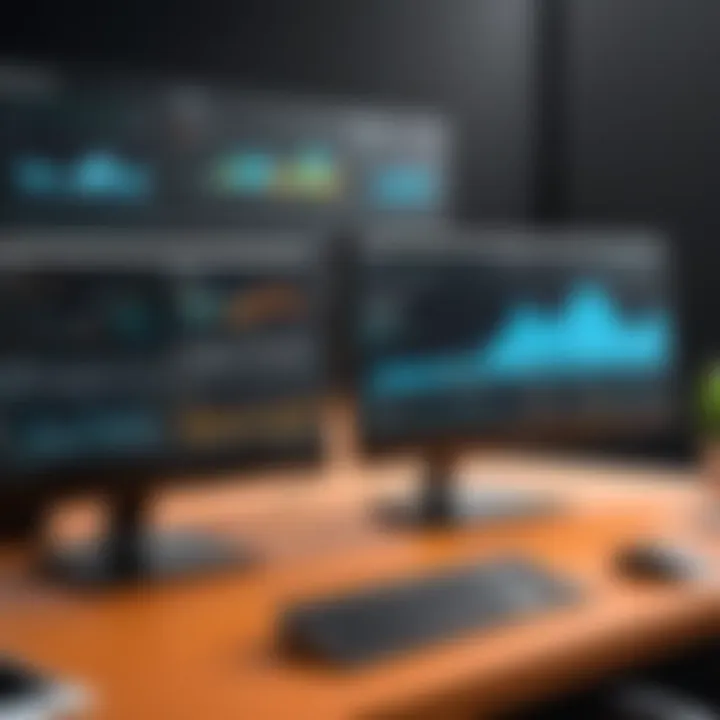
Integrating data analytics into operational monitoring creates a robust feedback loop that drives continuous improvements. Companies have successfully harnessed Splunk’s analytical capabilities to monitor system performance, leading to faster incident resolution and reduced downtime.
For example, an online service provider used Splunk for monitoring server performance. The system analyzed logs in real-time to pinpoint discrepancies in server health, which led to:
- Proactive Maintenance: Early detection of server errors resulted in scheduled maintenance that greatly reduced system outages.
- Enhanced User Experience: Improved performance metrics directly translated into a smoother user experience, leading to increased customer retention rates.
Best Practices for Data Analytics in Splunk
When it comes down to data analytics within Splunk, employing best practices can significantly elevate the effectiveness of your analysis. The sheer volume of data enterprises deal with today is overwhelming, and without a structured approach, insights can easily get lost in the noise. Best practices help streamline processes, ensure accuracy, and enhance the overall utility of the analytics performed. This isn't just about making sense of the data; it’s about optimizing workflows and gaining the sharpest insights possible.
By following these best practices, businesses can empower their teams to derive meaningful information, drive strategic decisions, and maintain a competitive edge in their respective markets.
Optimizing Queries for Efficiency
Crafting efficient queries in Splunk is akin to sharpening a knife before slicing through a roast—without it, progress will be sluggish and results might be underwhelming. Efficiency in query optimization can save time and resources, leading to quicker insights. Here are some methods for optimizing queries:
- Use of Wildcards: Be judicious with wildcards; they can either expedite your search or drag it down, depending on how they’re applied. A well-placed wildcard can save time in searching across multiple fields.
- Limit Data Retrieval: Instead of searching through all available data, it’s advisable to specify only the necessary fields. This cuts down on search time and improves performance, like riding a bike downhill instead of uphill.
- Leverage Summary Indexes: Utilize summary indexing for repetitive searches, storing the results of intensive queries so they don’t have to be recalculated. This is particularly helpful for generating reports.
- Regular Expressions: Get familiar with regex; it is a powerful tool for filtering data. A well-crafted regex can do what could take multiple lines of conditions in half the time.
By mastering these approaches, IT specialists can ensure that their querying technique mirrors a well-oiled machine: smooth, efficient, and effective.
Utilizing Machine Learning Toolkit
The Machine Learning Toolkit in Splunk is like adding a turbocharger to your car; it supercharges your analytics efforts by enabling predictive insights and identifying anomalies. This capability is vital in the modern landscape, where businesses need to stay ahead of the curve.
- Supervised Learning Models: For analyzing historical data, supervised learning can highlight trends and predict future outcomes. Businesses can anticipate customer behaviors, inventory needs, and security event occurrences more accurately.
- Unsupervised Learning: When data comes in without labels, unsupervised learning helps in clustering similar data points. This is particularly beneficial for threat detection where unknown patterns may indicate a security breach.
- Anomaly Detection: Using machine learning, teams can quickly identify outliers that signify issues. A sudden dip in metrics could suggest a potential system fault or malicious activity.
Incorporating machine learning into Splunk analytics not only amplifies data handling but also contributes to smarter decision-making by leveraging advanced algorithms to process large datasets efficiently.
Establishing Data Governance
Data governance can seem like a buzzword to some, yet, without a solid framework, organizations risk making decisions that are based on unclear or unreliable data. Governance sets the boundaries for data usage, ensuring security and compliance, much like the rules of the road keep traffic flowing smoothly.
- Data Stewardship: Assign roles that are responsible for data quality and accountability. Designating data stewards helps keep data consistent and trustworthy across different departments.
- Policy Development: Establish clear policies about data access, usage, and sharing. This prevents misuse and keeps sensitive information secure, mitigating risks associated with data breaches.
- Regular Audits: Implement periodic reviews of data integrity and compliance with established policies. This allows organizations to identify gaps proactively, correcting issues before they spiral.
In essence, solid data governance ensures that the data used in Splunk analytics holds up under scrutiny, enabling better decisions based on reliable insights.
The Future of Data Analytics within Splunk
Looking ahead, understanding the future of data analytics within Splunk is crucial for anyone aiming to leverage the platform's capabilities. It’s not just about keeping pace with technology but actually getting ahead of the curve in a world where data drives decisions. As organizations increasingly rely on their data for insights and strategies, knowing what’s on the horizon for Splunk and analytics can shape how businesses position themselves moving forward.
Emerging Trends in Data Analysis
Data analytics continues to evolve at an astonishing pace. Within Splunk, several significant trends are currently emerging, which are set to redefine how users interact with their data:
- Increased Automation: Automation tools in Splunk will likely see further development. By automating repetitive data tasks, teams can focus on deeper analytics rather than just data preparation.
- Advanced Machine Learning Applications: More predictive analytics features will emerge, allowing users to forecast trends with greater accuracy. Splunk’s integration with machine learning libraries can empower users to conjure advanced models tailored to their unique datasets.
- Enhanced Real-Time Analytics: As data generation rates soar, the need for real-time analytics becomes vital. This will demand more refined algorithms that can process data instantaneously.
- Collaboration Across Platforms: Splunk users can anticipate better integration with other platforms and tools. Seamless data flow between systems will enable versatile analytics and foster teamwork.
"In a world where knowledge is power, staying abreast of emerging trends is no longer optional, it’s essential."
Technologies such as edge computing and IoT devices are gaining strength, meaning data sources are diversifying. Splunk is adjusting to accommodate these shifts, which will create new data analytics solutions tailored to the evolving landscape and varied datasets.
Innovations on the Horizon
The path ahead for Splunk signals not just improvements but also groundbreaking innovations:
- Natural Language Processing (NLP): Future iterations of Splunk might incorporate NLP, allowing users to query their data using everyday language. Imagine transforming complex query syntax into something as simple as "Show me trends in user logins this week."
- Citizen Data Scientist: The shift toward empowering users without extensive technical backgrounds means more intuitive analytics tools utilizable by those with less experience. This democratization of data analysis could unleash significant innovative solutions across organizations.
- Augmented Reality (AR) and Virtual Reality (VR) Data Visualization: As technology progresses, so too will our methods of visualization. Incorporating AR and VR in data analysis allows for transformative insights, shifting visual data into an interactive experience.
- Decentralized Data Storage: New methods of storing and accessing data can emerge, with blockchain technology piquing interest. This could enhance data security and integrity, which is essential as businesses expand their digital footprint.
As these innovations come to fruition, they promise to revolutionize how organizations approach data analytics within Splunk. The end goal remains straightforward: enabling informed decision-making and operational excellence.
In summation, the future of data analytics within Splunk is bright and filled with potential. Those who can harness these trends and innovations will not only improve their analytics processes but also gain a significant competitive edge in their respective markets.
Epilogue
Wrapping up the discussion on data analytics within Splunk, it's clear that this platform plays a pivotal role in modern data management and analysis environments. The final insights into this topic are not merely academic; they resonate with real-world applications and practical benefits that organizations can leverage.
Recap of Key Insights
First and foremost, this article highlighted how Splunk serves as a robust tool for tackling the complexities of data analysis. The discussions spanned across several core areas:
- Real-Time Analysis: Splunk’s capability to sift through massive datasets in real-time provides organizations immediate insight and allows for quick decision-making.
- Predictive Analytics: This feature enables enterprises to anticipate future trends, enhancing strategic planning and resource allocation.
- Visualization Tools: The dashboards and visual tools that Splunk offers are crucial for simplifying complex data and making it accessible at various organizational levels, fostering a data-driven culture.
- Challenges in Implementation: While the benefits are compelling, the article did not shy away from discussing common pitfalls and data quality issues, reminding organizations to be vigilant and strategic about their analytics approaches.
Final Thoughts on Splunk and Data Analytics
By harnessing the power of data analytics through Splunk, businesses are not just reacting to data—they are learning from it, creating a cycle of continuous improvement and innovation in their operations.