Data Classification and Prevention for Secure Data Management
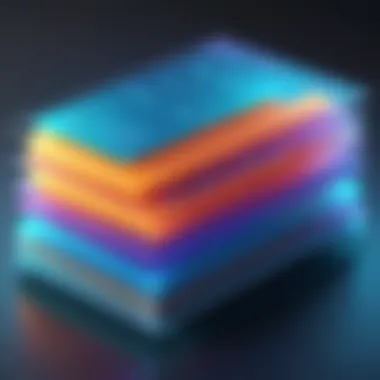
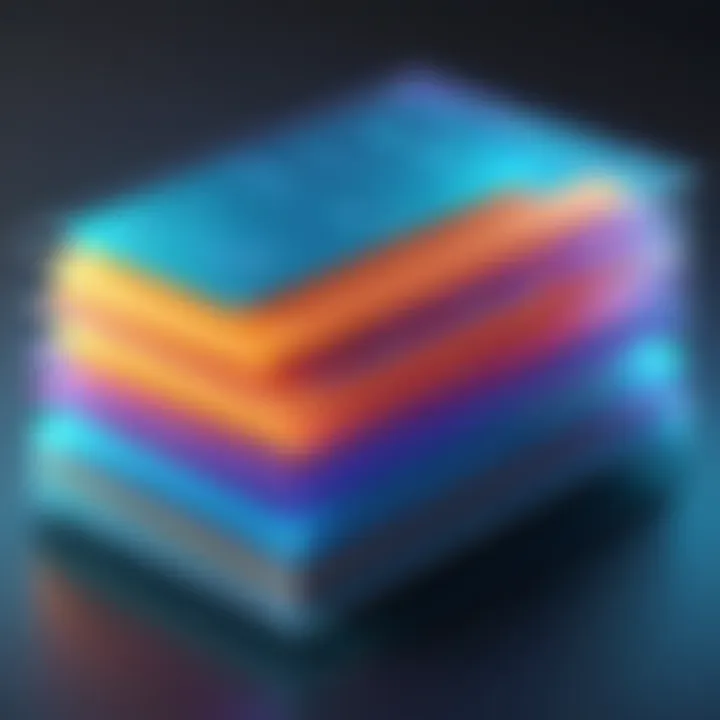
Intro
In an era where data has become a crucial asset, understanding its importance cannot be overstated. Businesses, regardless of size, are increasingly dependent on data to drive decisions and foster innovation. Yet, with this reliance comes the inherent risk of data breaches and loss. Protecting sensitive information is not merely a defensive strategy; it's a necessity to secure trust and maintain operational integrity. This is where the dual concepts of data classification and data loss prevention come into play.
By implementing robust data classification strategies, organizations can better categorize their information according to sensitivity and regulatory requirements. This fosters a structured approach, making it easier to understand which data requires enhanced protection and monitoring. Coupled with effective data loss prevention technologies, businesses can not only identify potential risks but also mitigate them before they escalate.
Thus, recognizing the interplay between these two strategies is essential for developing a comprehensive security posture that addresses contemporary challenges and threats.
Overview of Software
As data breaches are increasingly prevalent, software designed for data classification and data loss prevention has become indispensable. These tools help manage and secure sensitive information, ensuring compliance with regulations and protection against unauthorized access. Understanding the scope of this software and its key characteristics can aid IT and software professionals in selecting the solution that best meets their needs.
Description of Software
Typically, data classification and loss prevention software encompasses a range of functionalities. At its core, it enables organizations to categorize data based on various attributes, such as sensitivity, compliance requirements, and usage. This classification informs the subsequent data loss prevention strategies, establishing a multi-layered security approach.
For instance, the software might categorize data into groups like "Confidential," "Internal Use Only," or "Public Access." By enforcing policies associated with these classifications, the software helps to ensure that data is accessed and shared appropriately, minimizing the risk of exposure.
Key Features
When evaluating data classification and loss prevention software, several key features stand out:
- Automated Classification: Uses machine learning algorithms to automatically classify data based on predefined rules, reducing manual oversight.
- Policy Enforcement: Implements policies for data handling based on classifications, helping to ensure compliance with legal and corporate standards.
- Monitoring and Alerts: Provides real-time monitoring and alerts for unauthorized data access or transfers, allowing for swift action.
- Reporting and Auditing: Generates detailed reports that facilitate audits and provide insights into data handling practices.
Software Comparison
In selecting the right software for an organization, itโs crucial to compare available options effectively. Different products can vary significantly in their approach and features, making careful evaluation essential.
Comparison with Similar Software
For example, tools like Symantec Data Loss Prevention and McAfee Total Protection for Data Loss Prevention are often cited in industry discussions. Symantec focuses heavily on data discovery, whereas McAfee offers integrated solutions combining endpoint security with DLP features.
| Feature | Symantec | McAfee |
| Automated Classification | Yes | Yes |
| Centralized Management | Yes | Yes |
| Endpoint Integration | Yes | Yes |
Advantages and Disadvantages
With most software, advantages and disadvantages abound.
- Symantec Advantages: Strong data discovery capabilities, comprehensive reporting.
- Symantec Disadvantages: May have a steeper learning curve for new users.
- McAfee Advantages: Integrated protection across multiple attack vectors, user-friendly interface.
- McAfee Disadvantages: Resource-intensive on older systems.
"Having the right classification and prevention software can make all the difference in safeguarding sensitive information."
As organizations navigate the complexities of data security, understanding these tools' key features and comparing them effectively will enable informed decisions tailored to their specific needs.
Understanding Data Classification
Data classification serves as a crucial first step in the broader spectrum of data protection. Much like organizing a filing cabinet, it ensures that information is sorted into categories that make it easier to locate and manage. This not only boosts efficiency but also allows organizations to deploy targeted data security measures.
When businesses approach data classification correctly, it provides a framework that emphasizes valuable data as a priority. Consider it akin to having a well-organized toolbox where each tool is stored in its appropriate section, making it easier for employees to find what they need while minimizing the chance of sensitive information ending up in the wrong hands.
Moreover, the benefits of classifying data extend beyond mere organization. Companies can tailor their responses to potential threats and incidents based on the classification levels that correspond to the sensitivity of the data. In an age where data breaches seem to be a common occurrence, implementing a robust classification system is essential to maintaining integrity and compliance across various regulatory frameworks.
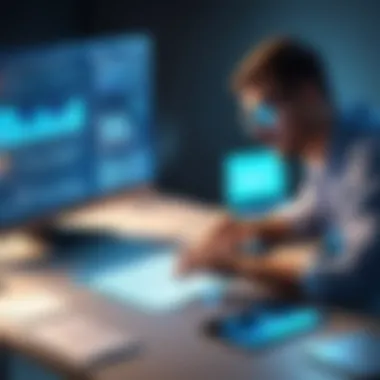
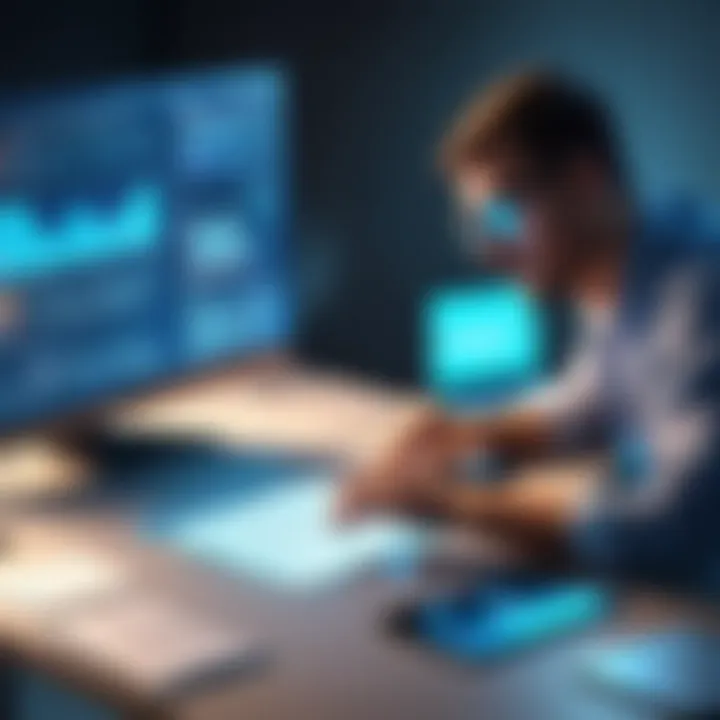
Defining Data Classification
Data classification involves categorizing information based on its type and sensitivity level. It forms the foundation of data governance and dictates how data should be handled, stored, and protected. Simply put, classification helps discern which data is routine and which is sensitive or critical to operations.
Types of Data Classification
- Content-based Classification
Content-based classification focuses purely on the data itself. This method assesses the information within a dataset to determine its sensitivity. For example, documents with personal identifiable information (PII) or trade secrets are classified as high sensitivity, while general notes or public documents are classified as low sensitivity.
Its key characteristic is the direct analysis of content, making it popular among organizations looking to safeguard personal data and proprietary information. It offers a straightforward and objective approach. However, one challenge lies in differentiating similar content types, which can lead to misclassification if not monitored closely. - User-based Classification
In contrast, user-based classification assigns data sensitivity according to who is accessing it. It acknowledges that certain users handle sensitive information differently based on their role within an organization. Thus, an HR manager might have access to personal data that a marketing executive does not.
This approach ensures data remains protected while allowing necessary access to individuals depending on their responsibilities. However, a drawback may arise if users misuse their privileges, necessitating vigilant oversight to prevent potential data leaks. - Context-based Classification
Context-based classification combines aspects of content and user classification to define data sensitivity based on the context. For example, a file marked for marketing might contain sensitive financial projections, and its classification might shift based on who is viewing it and what their intent is.
This type of classification is beneficial in dynamic environments where data context can change rapidly. Yet, it can lead to ambiguity during classification, requiring solid policies and oversight to maintain clarity.
Importance of Data Classification
Understanding data classification is pivotal for several reasons. It is foundational to regulatory compliance, risk management, and data governance. By grasping its significance, organizations can turn potential pitfalls into their strengths.
- Regulatory Compliance
Regulatory compliance necessitates that businesses adhere to specific legal requirements concerning data protection. Knowing exactly how to classify data helps entities abide by laws such as the General Data Protection Regulation (GDPR) or Health Insurance Portability and Accountability Act (HIPAA). This proactive measure can mitigate the risk of costly fines. As such, clear classification systems align with compliance efforts, safeguarding organizations against potential violations. - Risk Management
In today's landscape, risk management goes beyond preventing data breaches. Itโs about understanding the potential impacts and tailoring responses accordingly. Effective classification allows organizations to identify high-risk areas, thus prioritizing security efforts on the most sensitive data. The unique advantage lies in creating an adaptable, risk-aware culture that promotes vigilance and preparedness. - Data Governance
Lastly, the essence of data governance lies in establishing policies and procedures that dictate how data should be handled. By classifying data effectively, organizations can ensure accountability and streamline decision-making processes. It provides clarity in roles and responsibilities while outlining how data relates within the organization. This structured approach ensures data remains a valuable asset rather than a liability.
Data classification is not merely an option but a necessity in todayโs data-driven world. It provides the blueprint for effective data management and security.
Data Loss Prevention Explained
In an age where information is the new gold, keeping data safe holds paramount importance. Data loss prevention (DLP) serves as the first line of defense against potential threats that could compromise sensitive information. The core idea of DLP is to protect data from unauthorized access, misuse, or inadvertent loss, which can lead to financial instability and reputational damage. Essentially, it focuses on identifying, monitoring, and protecting data wherever it resides or travels. This holistic approach is essential for any modern organization aiming to maintain integrity and trust.
What is Data Loss Prevention?
Data loss prevention refers to strategies and tools that help safeguard sensitive data from theft, loss, or inadvertent sharing. Organizations implement DLP solutions to ensure compliance with regulations, maintain privacy of customer and employee information, and safeguard intellectual property. Without effective DLP measures in place, companies are at risk of data leaks, which could have dire consequences. A robust DLP framework not only shields valuable data assets but also provides peace of mind for stakeholders.
Core Components of Data Loss Prevention
Data Identification
The essential aspect of data identification lies in recognizing what constitutes sensitive data within an organization. It involves tagging and categorizing data based on its confidentiality and regulatory requirements. This process allows businesses to prioritize security efforts toward data that is most at risk. The key characteristic here is the ability to classify data accurately. This is a beneficial strategy, as it ensures that the most sensitive information receives the tightest security controls. A unique feature of data identification processes is the use of automated tools that can sift through vast amounts of information, identifying and tagging sensitive data efficiently. However, automated systems can sometimes misclassify data, leading to potential oversights.
Monitoring
Monitoring is crucial for tracking data movements and usage within an organization. It provides visibility into who accesses data, when, and how they engage with it. This characteristic is vital as it enables organizations to detect any unauthorized attempts to access or mishandle sensitive data. One significant feature of monitoring systems is the real-time alerts they generate, informing security teams of suspicious activity immediately. Such features, however, can lead to information overload if proper thresholds for alerts are not set, possibly desensitizing security personnel to legitimate threats.
Protection Mechanisms
Protection mechanisms are the tactics and tools employed to physically secure data from threats, breaches, or losses. These mechanisms could include encryption, tokenization, and data masking. Their key characteristic is variability; organizations can deploy varying levels of protection based on data sensitivity levels. This flexibility helps businesses manage costs and resource allocation effectively. A notable feature of these mechanisms is their adaptability to hybrid environments, ensuring data remains secure across on-premises and cloud storage. However, organizations may find implementing these protections cumbersome, especially if they have legacy systems in place that do not integrate well with modern solutions.
The Role of Policies in Data Loss Prevention
Policies serve as the backbone of any data loss prevention strategy. They provide guidelines that dictate how data should be handled, processed, and shared across the enterprise. Establishing clear DLP policies empowers employees to understand their roles in protecting sensitive information, thereby fostering an organizational culture of compliance and security. The key characteristic of effective policies is their adaptability; they need to evolve in response to emerging threats and changes in regulations. Uniquely, well-constructed policies can also aid in regular audits, serving as a benchmarking tool for evaluating organizational performance in data protection. Nonetheless, overly complex policies might lead to confusion among staff, hindering compliance and effectiveness.
Policy Frameworks
A policy framework outlines the structure and guidelines related to data management and protection. It helps in establishing a blueprint for DLP implementation across the organization. An important characteristic of such frameworks is clarityโclear frameworks enhance understanding among employees about how to manage sensitive data responsibly. The unique advantage of policy frameworks is their ability to integrate with various business processes. The downside is the potential rigidity, as too strict a framework can create friction and resistance among staff members accustomed to certain workflows.
Enforcement Strategies
Enforcement strategies are vital in ensuring that DLP policies are not merely words on a page but are actively integrated into the daily operations of an organization. These strategies rely on a blend of technology and manual oversight to ensure compliance with established policies. A standout characteristic of enforcement strategies is their proactive nature, as they monitor adherence to data protection measures continually. Unique aspects of successful enforcement strategies can include a focus on immediate feedback loops for employees who might inadvertently breach protocols. However, if enforcement measures are perceived as punitive rather than corrective, it might create an atmosphere of apprehension rather than one of education and improvement.
"In todayโs data-driven world, understanding and implementing robust data loss prevention strategies is not just a good practiceโit's paramount for the survival of an organization."
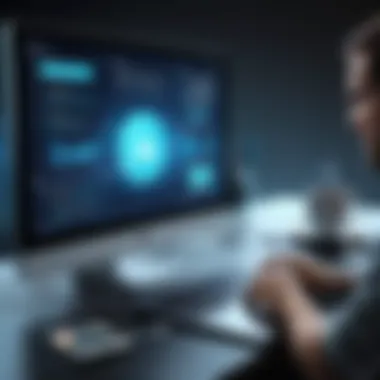
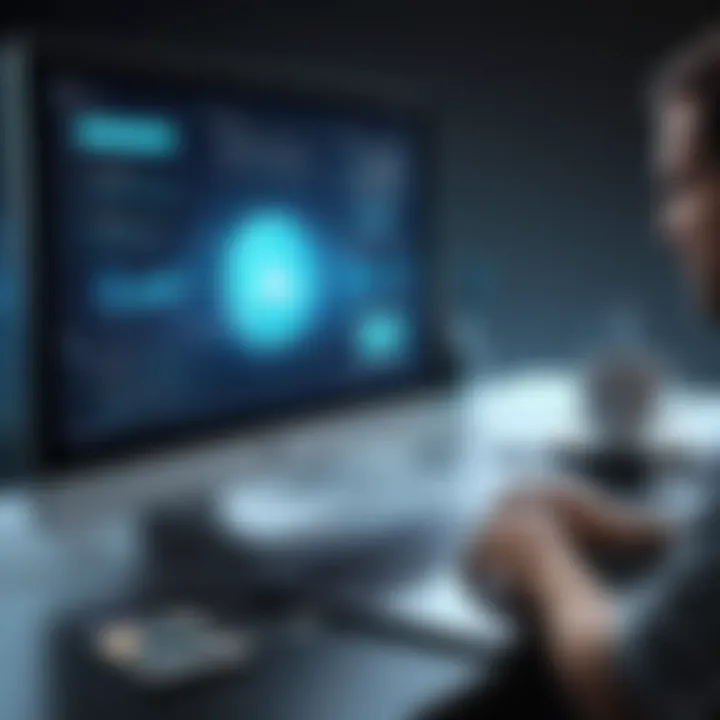
Integrating Data Classification and Data Loss Prevention
As organizations grapple with the complexities of safeguarding sensitive information, the integration of data classification and data loss prevention becomes imperative. Why is this merging of practices so essential? The crux lies in understanding that both components serve as foundational pillars in a comprehensive data security strategy. When classification methods are aligned with loss prevention techniques, organizations can more effectively manage risks, ensuring that critical data remains shielded from both internal and external threats.
Data classification lays the groundwork for data loss prevention by providing a framework that defines what data needs protection and to what degree, facilitating targeted loss prevention initiatives. This alignment not only enhances security postures but also streamlines regulatory compliance efforts. Essentially, merging these strategies yields a holistic view, allowing businesses to prioritize their protective measures based on data sensitivity, and thus makes operations far more efficient.
"Integrating these two approaches can yield a synergy, where the whole becomes significantly greater than the sum of its parts."
How Classification Enhances Data Loss Prevention
Classification greatly uplifts the efficacy of data loss prevention strategies. By categorizing data into various levels of sensitivity, organizations can apply different security measures tailored to the specific needs of each category. For instance, highly sensitive financial data might require stronger encryption and access controls than less critical data, such as marketing content. This targeted approach not only helps in allocating resources judiciously but also simplifies compliance with regulations such as GDPR and HIPAA.
Moreover, when data is classified correctly, organizations can respond more promptly to potential data breaches. For example, if an incident occurs involving confidential personnel files, swift containment can be activated based on prior classification, without wasting time on less sensitive data.
Technological Solutions for Integration
Unified Security Platforms
Unified security platforms serve as the backbone of this integration between data classification and data loss prevention. These platforms consolidate various security functions into a single interface, making it easier for IT teams to monitor and manage security threats effectively. One key characteristic of unified security platforms is their capability to seamlessly integrate classification tags into loss prevention policies. This allows data to be protected according to its sensitivity level, employing tailored preventive measures specific to each classification type.
A unique feature of these platforms is their ability to automate data visibility, allowing organizations to see where sensitive information resides across their networks. While the primary advantage is streamlined protection, the downside can sometimes be the initial learning curve required for IT staff to adapt to such comprehensive systems. Yet, once the system is in place, the operational benefits often outweigh these initial challenges.
Automated Classification Tools
Automated classification tools represent another cutting-edge technology in this integration effort. They utilize machine learning algorithms to analyze data and apply classification labels based on established criteria, saving organizations substantial manual effort and reducing human error. One of the significant benefits of these tools is their ability to scale as data volumes increase, ensuring that classification remains accurate and up-to-date.
However, these tools do come with their unique challenges. As sophisticated as they are, they can only operate within the boundaries of their programming and may not always interpret nuanced context correctly. Therefore, a robust human oversight mechanism is vital to complement automated classification, ensuring that critical data is correctly identified and secured.
Case Studies of Successful Integration
To illustrate the tangible benefits of integrating data classification with data loss prevention, one can look into case studies of organizations that have adeptly combined these strategies. For example, a fintech company implemented a unified security platform alongside automated classification tools, resulting in a measurable decrease in unauthorized data access incidents. The proactive monitoring and quick response capabilities afforded by their integrated approach not only protected sensitive customer information but also strengthened their compliance posture significantly.
With another organization, a large multinational had previously struggled with regulatory audits. By adopting a cohesive strategy to classify and protect their data effectively, they found themselves better equipped to manage compliance, resulting in fewer fines and improved stakeholder trust.
The journey toward successfully integrating data classification and data loss prevention isn't without its challenges. However, the payoff can be substantial, underscoring the importance of this combined approach in today's data-driven world.
Challenges in Data Classification and Data Loss Prevention
Understanding the difficulties that arise in data classification and data loss prevention is crucial for organizations striving to safeguard sensitive information. Tackling these challenges can not only enhance an organizationโs security posture but also foster a culture of compliance and awareness among employees. Without addressing these obstacles, even the best-designed strategies may fail to protect against data breaches or inadvertent data loss.
Technical Challenges
Data Complexity
Data complexity refers to the vast array of formats, types, sources, and repositories that data can originate from. As organizations grow, so does their data, leading to an intricate web of information that can vary greatly in structure and meaning. The fundamental characteristic of data complexity is its heterogeneity; think spreadsheets, databases, emails, and cloud-based documents all coexisting. This variety presents a unique challenge: how to effectively classify and manage such disparate data types.
Managing data complexity is preferred in our discussion because it highlights the potential pitfalls one might encounter when trying to implement a cohesive data strategy. For instance, a lack of standardized categorization could lead to misplaced information, making it difficult to apply appropriate data loss prevention measures effectively.
The unique feature here is that complex data environments often require advanced technologies like machine learning and automated classification tools to intelligently process and sort information. The advantage of this approach is that it can enhance accuracy, but the disadvantage lies in the time and resources needed to set up and maintain these systems.
Scalability Issues
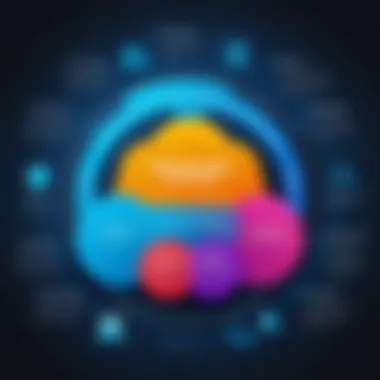
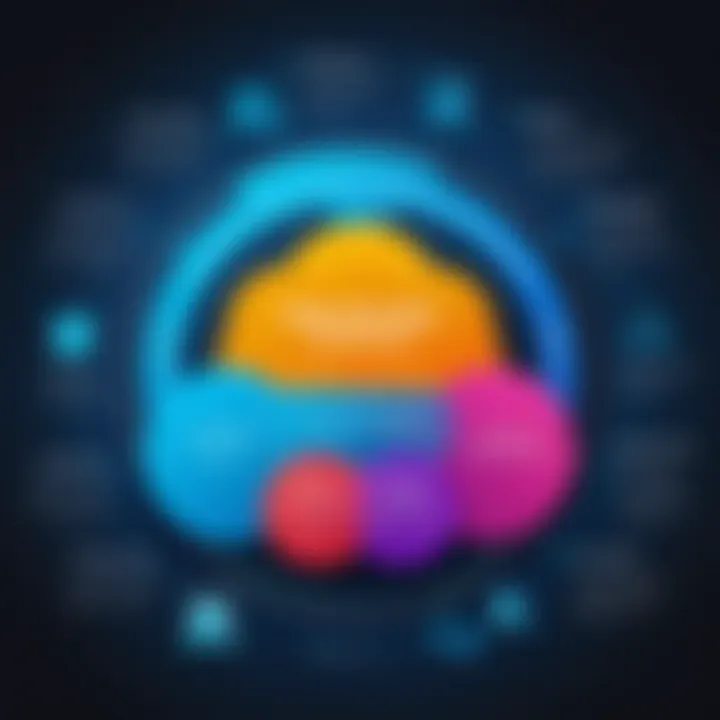
Scalability issues emerge when organizations attempt to grow their data classification and loss prevention systems in lockstep with their business expansion. While a small business might manage data effectively with a rudimentary approach, that strategy can become cumbersome as data volumes increase.
The key characteristic of scalability issues is that they demand solutions that can evolve without compromising performance or security. When organizations try to force existing frameworks to accommodate increased loads, they often run into performance bottlenecks. This aspect is vital for our article since the ability to scale successfully sets apart resilient organizations from those that struggle under the weight of growing data requirements.
A unique feature of scalability challenges is their demand for dynamic adjustments; systems should adapt to handle larger volumes of data or more complex regulations over time. The advantage of anticipating scaling needs is that it allows for smoother transitions during growth phases, but the disadvantage is that it typically requires foresight and investment in advanced technologies, which may not be feasible for all.
Human Factors and User Compliance
Training and Awareness
One of the main components in ensuring data classification and loss prevention efforts succeed is human involvement. In this vein, training and awareness serve as the backbone of a strategy that relies heavily on user compliance. Well-trained employees understand the importance of data security and recognize their role in protecting sensitive information, which contributes to the overall goal of the organization.
The central aspect of training and awareness is that it promotes a culture of security mindfulness among staff. When organizations invest in regular training, employees are less likely to fall victim to phishing attacks or mishandle sensitive data. This level of engagement is beneficial because informed teams will proactively seek to identify and mitigate risks.
A unique feature of effective training programs is their adaptability; they can be tailored to address specific roles and data types. The advantage lies in enhanced adherence to security protocols, while the disadvantage is the potential for knowledge decay if training is not updated or reinforced regularly.
Resistance to Change
Resistance to change is another significant hurdle that organizations face when implementing new data classification and loss prevention controls. Employees may feel threatened by new technologies or policies, leading to pushback that can derail otherwise well-planned initiatives. This aspect is particularly relevant, as it impacts the organization's effectiveness in safeguarding its data.
The primary characteristic of resistance to change is an emotional reaction from users who are comfortable with existing workflows. This can often create silos within departments that undermine overall compliance efforts. It is crucial to highlight this topic because understanding employee sentiment can inform better change management practices.
One unique feature here is the ability to integrate feedback loops within change processes. By fostering open communication and addressing concerns, organizations can ease transitions. The advantage of this approach is increased buy-in from users, while the disadvantage is the additional time and resources needed to manage this dialogue effectively.
The success of data classification and loss prevention is not solely reliant on technology but also heavily influenced by human factors. A well-informed and willing workforce can be your first line of defense against data threats.
Future Trends in Data Classification and Protection
Future trends in data classification and protection are shaping how businesses, regardless of size, approach data security. As organizations increasingly rely on data for decision-making, understanding these trends becomes critical for ensuring the integrity and confidentiality of sensitive information. This section highlights the evolving landscape of data management, focusing on innovations driven by technology and the pressing need for adaptable strategies in a dynamic environment.
AI and Machine Learning in Data Management
The integration of artificial intelligence (AI) and machine learning (ML) into data management is not just a fad; it signifies a leap towards smarter, more efficient processes. These technologies enable organizations to learn from data patterns and apply insights to improve classification accuracy and data loss prevention efforts. One prominent benefit is the automation of data tagging and classification, which can drastically reduce human error and save time.
For instance, using AI algorithms, enterprises can analyze vast datasets to identify sensitive information quickly and classify it based on predefined parameters. This capability not only optimizes data handling but also enhances ongoing monitoring and compliance efforts. As ML models continue to evolve, they will become adept at recognizing evolving threats and anomalies in data behavior, allowing organizations to stay one step ahead in data protection.
"AI and machine learning arenโt just tools; theyโre strategies for future-proofing your data management processes against emergent threats."
Cloud Technologies and Data Security
The rise of cloud technologies has fundamentally changed the way businesses approach data storage and management. As more organizations migrate to the cloud, data security strategies must adapt accordingly. Cloud service providers often implement robust security measures, but ultimately, responsibility lies with the businesses leveraging these platforms.
With cloud computing, data classification becomes a multi-faceted challenge. Organizations must ensure that the classification aligns not only with internal policies but also with the compliance requirements dictated by service agreements. Additionally, continuously assessing the cloud security landscape becomes necessary to mitigate risks such as data breaches or unauthorized access.
Key considerations for leveraging cloud technologies include:
- Data Encryption: Always ensure data is encrypted both in transit and at rest.
- Access Control: Implement strict permissions and access controls to safeguard data.
- Regular Audits: Conduct regular audits of your cloud service providerโs data security practices.
Innovations and Emerging Tools
In the realm of data classification and protection, continuous innovation offers fresh solutions to persistent challenges. Emerging tools designed for both large corporations and small businesses focus on enhancing efficiency and security. For instance, tools that integrate real-time analytics with data classification systems allow organizations to react swiftly to threats as they arise.
Moreover, blockchain technology is beginning to find its way into the data protection landscape. By providing a decentralized and tamper-proof method for verifying data integrity, blockchain presents a promising avenue for securing classification and distribution processes.
Some notable advancements include:
- Automated Data Classification Platforms: Leverage intelligent software that can adapt to new types of data.
- Unified Data Protection Solutions: Centralized systems that streamline security measures across various platforms.
Embracing these innovations not only fortifies a companyโs defenses but also cultivates a culture of proactive data management that can adjust to future demands.