Exploring Data and AI Consulting: Trends and Insights
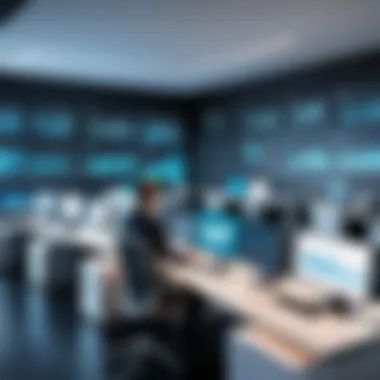
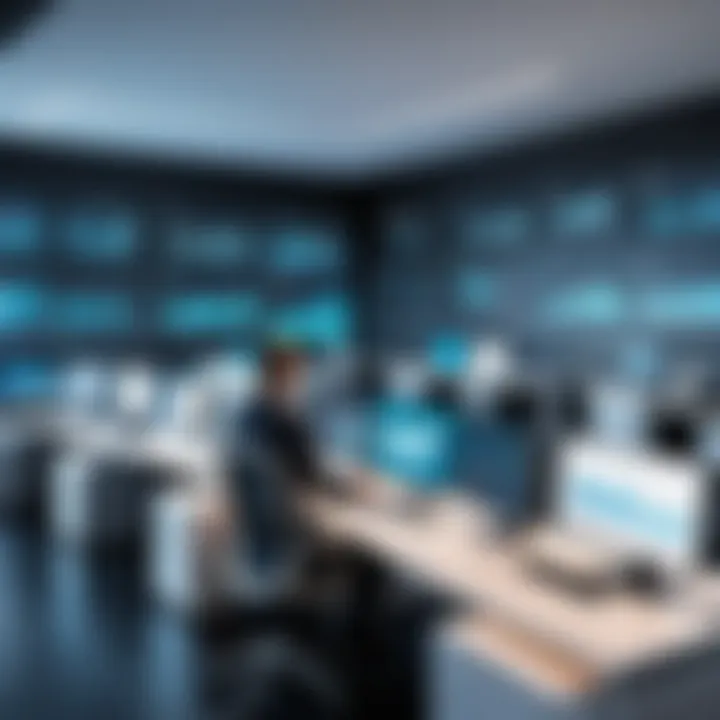
Intro
The consulting field is rapidly transforming, particularly in areas involving data and artificial intelligence. These two components are no longer niche subjects limited to specialized professionals; they have become critical assets for organizations of all sizes. The emergence of data-driven decision-making signifies a shift in traditional consulting methods toward a more analytical approach. It is essential for professionals to keep abreast of ongoing changes and understand how these elements interact to drive business success.
In this article, we will explore the various dimensions of data and AI consulting, focusing on the importance of software solutions. We will dissect methodologies, tools, challenges, and future trends that are relevant in the current landscape. By understanding these components, businesses can effectively leverage data strategies to enhance decision-making.
"Data is often called the new oil, but data is only valuable when refined."
The decision to implement AI and data analysis solutions can be daunting. Hence, comprehending the implications and evaluating the options available becomes crucial. Moreover, authentic peer reviews play a significant role in this landscape, influencing the selection process of the right tools and software solutions.
Understanding Data and AI Consulting
Data and AI consulting represent a critical intersection of technology and business. This domain addresses how organizations can leverage their data and artificial intelligence capabilities to drive decisions that enhance efficiency and promote growth. A nuanced understanding of this field helps professionals navigate the complex landscape of information and technology, shaping organizational strategies effectively.
Definition and Scope
Data consulting typically involves the analysis, management, and strategy for data utilization within an organization. It encompasses the methods through which data is collected, processed, and transformed into actionable insights. AI consulting, on the other hand, focuses on integrating artificial intelligence technologies to automate tasks, deepen insights, and refine processes. Both areas are interrelated and crucial in establishing data-driven cultures in businesses.
The scope of data and AI consulting encompasses various industries including finance, healthcare, retail, and manufacturing. It extends from performance optimization to customer relationship management, ensuring organizations effectively use their data assets. Specialists in this field utilize various methodologies, frameworks, and tools to navigate challenges and identify opportunities.
Historical Context
Over the past few decades, the advent of technology has dramatically changed how businesses operate. The rise of the internet and digital communication provided vast amounts of data, necessitating strategies for effective management. The early 2000s marked the beginning of serious investment in data analytics as companies sought competitive advantages through informed decision-making.
Then, with the introduction of advanced technologies such as machine learning and big data, organizations recognized the potential of data to not just support decisions, but to drive innovation. AI consulting emerged as a separate discipline as businesses anticipated the impact of intelligent systems on operations and customer engagement. This evolution continues today, with a focus on ethical considerations and data governance, ensuring that these powerful tools are used responsibly.
The historical context sets the foundation for understanding why data and AI consulting are now indispensable elements within business strategies. As companies continue to embrace digital transformation, the demand for effective consulting in these areas will only increase.
The Importance of Data in Decision-Making
Data has become a cornerstone of modern business practices. In an age where information flows freely and rapidly, organizations that harness the power of data can gain significant advantages over their competition. Understanding how data influences decision-making is crucial to fostering innovation and ensuring operational efficiency. This section highlights the value of treating data as a vital asset and discusses how to transform it into meaningful insights.
Data as an Asset
In today's information-driven landscape, data is not merely a byproduct of business operations; it is an asset that can drive strategy and growth. Organizations recognize that effective data management can yield better outcomes. Unlike traditional resources, data can be continuously accumulated and analyzed, leading to enhanced forecasting and improved service delivery.
Having quality data at oneโs disposal allows businesses to make informed decisions that have far-reaching implications. For instance, retail companies utilize customer purchase data to tailor marketing strategies. This enables them to target specific demographics, thus maximizing their return on investment. While the infrastructure to manage large datasets may require investment, the potential returns make it worthwhile.
An effective data strategy requires a change in mindset across all levels of an organization. Employees must understand why data is essential and how it can be leveraged in their respective roles.
Transforming Data into Insights
Simply collecting data is not enough; the critical challenge lies in converting this data into actionable insights. To do this, organizations must employ various analytical methodologies and technologies. The utilization of robust tools for data analytics allows businesses to identify patterns, relationships, and trends that inform decision-making.
Key steps include:
- Data Cleaning: Raw data often contains errors and inconsistencies. Cleaning processes are crucial to ensure that the information used is reliable.
- Data Analysis: By employing techniques such as statistical analysis and machine learning, organizations discern underlying patterns in data. This can illuminate factors contributing to success or identify potential pitfalls.
- Data Visualization: Presenting data visually enables teams to interpret complex information more easily. Tools like Tableau and Power BI create intuitive dashboards that summarize key metrics, thereby facilitating quick insights.
Transforming data into insights isn't a one-off process but should occur continuously as more data is collected and analyzed. It is about creating a feedback loop where insights feed into strategic planning and operational adjustments.
"In the era of information overload, the ability to extract relevant insights from data determines an organization's ability to thrive." - Data Analyst Overview
AI: A Catalyst for Innovation
Artificial Intelligence (AI) has emerged as a crucial element in the landscape of data consulting. Its integration into business processes transforms traditional methods of analysis and decision-making. The ability to process vast amounts of data efficiently has made AI indispensable. By analyzing patterns and trends more swiftly than any human analyst, AI can provide insights that drive strategic decisions.
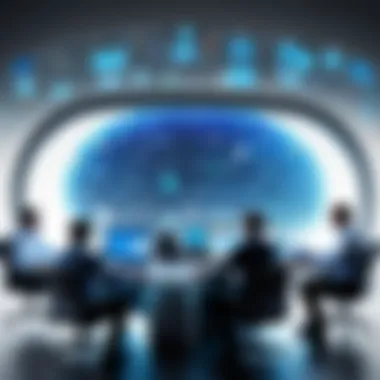
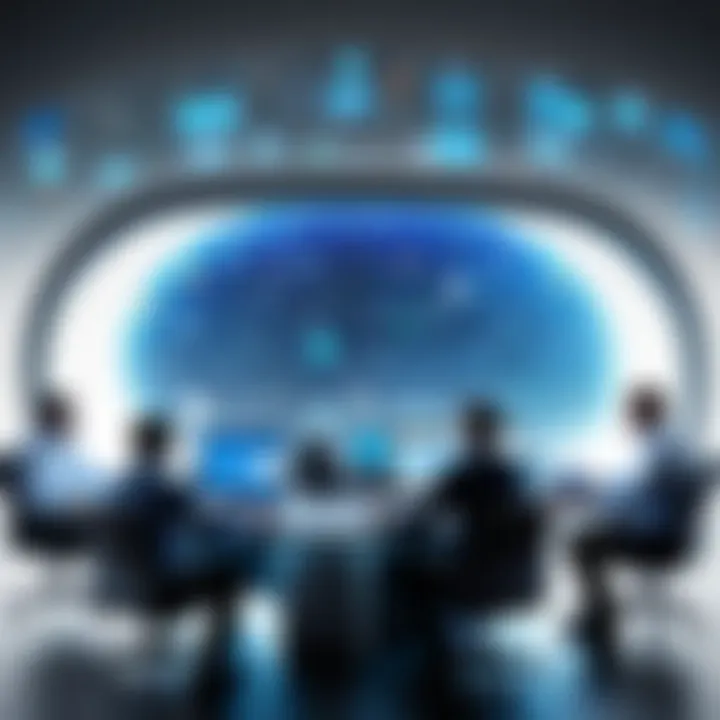
Machine Learning and Its Applications
Machine Learning is a prominent subset of AI that focuses on algorithms and statistical models that enable computers to perform tasks without explicit instructions. This technology learns from data, improving its performance over time through experience. In data consulting, machine learning finds applications in numerous areas, including but not limited to:
- Predictive Analytics: This involves the use of historical data to predict future outcomes. Businesses use predictive models to enhance areas like sales forecasting, risk management, and customer behavior analysis. For instance, an e-commerce company may use machine learning to predict which products are likely to sell during a specific season based on historical trends.
- Anomaly Detection: In sectors such as finance and cybersecurity, detecting anomalies is crucial. Machine learning algorithms can identify patterns of normalcy within a dataset and flag unusual occurrences, thus preventing fraud or data breaches. Organizations like PayPal leverage this technology to monitor transactions in real-time, enhancing security.
- Recommendation Systems: Services like Netflix and Amazon use machine learning for recommendation engines. These systems analyze user behavior and preferences to suggest content or products, thereby improving user satisfaction and engagement.
Natural Language Processing
Natural Language Processing (NLP) is another vital component of AI, enabling machines to understand and interpret human language. This capability broadens the scope of data consulting in ways that were previously unimagined. AI-driven NLP tools can analyze customer feedback, social media interactions, and various text-based data to gather insights about public sentiment and market trends.
- Sentiment Analysis: Machine learning techniques structured through NLP allow businesses to gauge public opinion regarding their products or brand. This analysis informs marketing strategies and product development. Understanding consumer sentiment can significantly alter how a company positions itself in the market.
- Chatbots: Many companies deploy chatbots powered by NLP to enhance customer service. These bots can respond to inquiries, assist with problem-solving, and streamline communication processes, thereby improving overall operational efficiency.
"AI helps us go beyond traditional data analysis, informing decisions with unprecedented accuracy and speed."
The implications of adopting AI are profound, affecting everything from operational efficiency to customer engagement.
Consulting Methodologies in Data and AI
Consulting methodologies in data and AI play a crucial role in shaping the effectiveness of projects in this fast-evolving landscape. These methodologies detail the approaches and processes that consultants employ to tackle complex data and AI challenges facing organizations. Each methodology brings unique strengths, guiding businesses to make informed decisions. Understanding these methodologies can support IT professionals and business leaders in selecting suitable strategies that align with their specific needs.
Agile vs. Traditional Approaches
Both Agile and traditional methodologies offer distinct advantages in data and AI consulting.
Agile is characterized by flexibility and iterative progress. The approach allows teams to adapt to changes and incorporate feedback quickly. It emphasizes collaboration and continuous improvement, making it well-suited to projects in dynamic environments like AI development. Teams can respond to new insights about data and adjust their strategies accordingly. This leads to faster results and aligns closely with the rapid pace of technological advancements.
On the other hand, traditional methodologies typically follow a linear process, often divided into sequential phases: requirements gathering, design, implementation, verification, and maintenance. This approach might be more familiar to some organizations, providing a clear framework that can enhance accountability. However, it may not accommodate changes as fluidly as Agile methodologies, potentially leading to missed opportunities for optimization.
Understanding when to deploy Agile versus a traditional methodology requires careful consideration of project specifics. Before engaging, organizations should assess their capacity for change, urgency of outcomes, and the volatility of data.
Data Governance Frameworks
Data governance frameworks form a foundational aspect of data and AI consulting. These frameworks establish the policies, procedures, and standards for managing and using data within an organization. Implementing sound data governance is essential for ensuring data quality, compliance, and security.
A robust data governance framework includes several key elements:
- Data Stewardship: Designating individuals responsible for managing data assets and ensuring compliance with established standards.
- Data Quality Management: Setting criteria for data accuracy and consistency, which is fundamental to effective decision-making.
- Compliance Monitoring: Ensuring adherence to regulations like GDPR or HIPAA, safeguarding against legal repercussions.
- User Access and Security Policies: Defining who can access data and under what circumstances to maintain confidentiality and integrity.
Establishing a strong governance framework contributes to building trust in data-driven initiatives. Organizations that prioritize governance often see improved outcomes, as they can make data-driven choices backed by accurate and reliable information. Overall, the implications of data governance extend beyond regulatory compliance, impacting business strategy and growth.
"Effective data governance minimizes risks and maximizes value from data assets."
Tools and Technologies in Data Consulting
In the realm of data and AI consulting, the tools and technologies employed are pivotal. They serve as the backbone for analysis, visualization, and predictive modeling. By leveraging advanced software, consultants can derive actionable insights from complex datasets. The integration of appropriate tools not only enhances productivity but also ensures accuracy in decision-making processes. Furthermore, these technologies help in navigating the expansive landscape of data, optimizing its utility for various industries.
Data Visualization Softwares
Data visualization softwares play an essential role in transforming numerical data into comprehensible visual formats. This is crucial because often, raw data appears confusing or overwhelming. With tools like Tableau, Microsoft Power BI, and QlikView, professionals can create interactive dashboards and reports that present trends and patterns clearly.
Benefits of Data Visualization Softwares:
- Enhanced Comprehension: Visuals make it simpler to grasp complex information.
- Informed Decision-Making: Stakeholders can make faster decisions with clear visual data representation.
- Collaboration: These tools often support sharing capabilities, fostering teamwork.
However, it's essential to consider the learning curve associated with each tool. Some softwares require significant training to maximize their potential. Therefore, organizations should assess their team's current skill levels before committing to a specific solution.

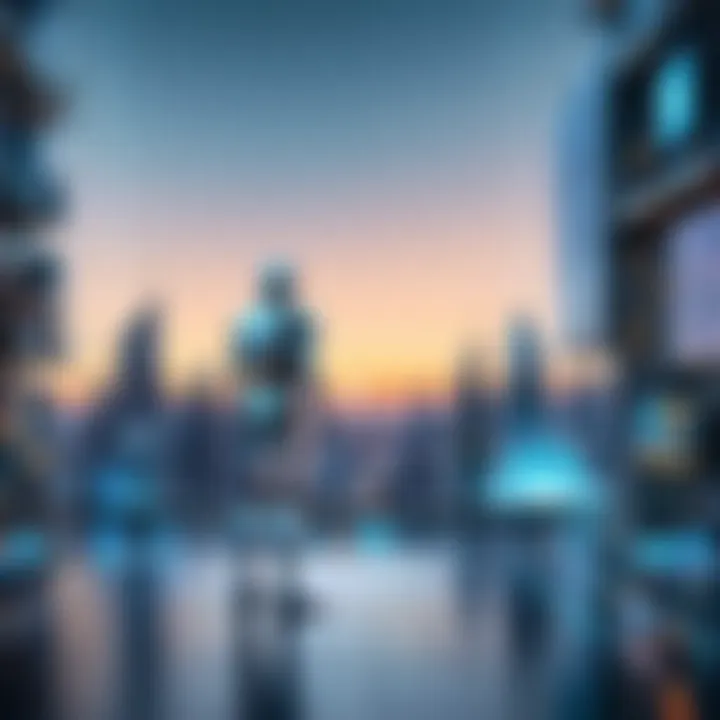
Predictive Analytics Tools
Predictive analytics tools are at the forefront of data consulting, enabling organizations to foresee trends and make preemptive decisions. Using algorithms and statistical techniques, these tools analyze historical data to predict future outcomes. Popular options include SAS, IBM SPSS, and RapidMiner.
Advantages of Using Predictive Analytics Tools:
- Data-Driven Decisions: Organizations rely on solid data insights rather than assumptions.
- Risk Reduction: Anticipating changes can help mitigate risks before they escalate.
- Resource Optimization: Predictive analytics can reveal areas to allocate resources more effectively.
While these tools offer significant advantages, it is crucial that users understand the foundations of the models they utilize. Blindly trusting results without interpreting the underlying data can lead to misinformed strategies.
"Data is the new oil. Itโs valuable, but if unrefined, it cannot really be used."
Challenges in Data and AI Consulting
Data and AI consulting is replete with challenges that must be addressed for successful implementation. In the current landscape, organizations are increasingly reliant on data-driven strategies. However, without overcoming these challenges, the effectiveness of such strategies is compromised. This section delves into key hurdles such as data quality management and ethical concerns, both of which are crucial for IT professionals and businesses.
Managing Data Quality
Managing data quality stands at the forefront of challenges in data and AI consulting. Poor data quality can severely impact decision-making and strategic outcomes. Several factors contribute to data quality issues, including outdated information, inconsistent formats, and inaccuracies in data entry.
To effectively manage data quality, organizations should adopt the following practices:
- Regular Audits: Conduct audits to identify discrepancies and rectify errors. This helps maintain an accurate and up-to-date data repository.
- Standardization Protocols: Implementing standard data formats ensures consistency across various systems, reducing confusion and improving integration capabilities.
- Data Governance Frameworks: Establishing a solid governance framework allows organizations to oversee data management processes and ensure accountability.
By prioritizing data quality, firms can not only improve efficiency but also enhance the reliability of insights drawn from AI applications. Raising data quality is not merely a technical undertaking, but a strategic one that involves cultural shifts within the organization.
"High data quality is essential for effective Artificial Intelligence applications; without it, results can be misleading."
Ethical Considerations
Ethical considerations are an equally important aspect of data and AI consulting. As the use of AI expands, so do the concerns about privacy, bias, and the moral implications of automated decision-making. Businesses must be proactive in establishing ethical frameworks to navigate these challenges.
Some pressing ethical considerations include:
- Data Privacy: Respecting user privacy and adhering to regulations such as GDPR are paramount. Organizations must ensure that data collection practices do not compromise individual rights.
- Bias in AI Algorithms: AI systems can develop biases based on the data fed into them. This can result in unfair treatment of certain groups. Regular audits to evaluate biases and taking corrective actions can mitigate this risk.
- Transparency: Providing transparency in AI processes helps build trust. Stakeholders should understand how decisions are made, ensuring accountability in AI deployments.
In addressing these ethical challenges, companies not only reinforce their brand reputation but also contribute positively to society at large.
Evaluating Software Solutions for Consulting
Evaluating software solutions for consulting is a critical phase in maximizing the effectiveness of data and AI strategies. In an environment characterized by rapid technological advancement, the challenge lies not only in selecting tools but also in ensuring they align with business needs and objectives. The right software can enhance data processing capabilities, improve insights, and streamline workflows. Therefore, taking a structured approach to evaluation helps in identifying solutions that genuinely meet organizational demands.
Criteria for Evaluation
When assessing software solutions, several criteria come into play. These elements include:
- Functionality: Does the software provide the necessary features? It is important that it supports specific analytical tools, visualization techniques, and integration capabilities relevant to ongoing projects.
- Usability: A user-friendly interface increases productivity. Testing the ease of use can uncover how quickly the team can adopt the new tool, ultimately affecting overall satisfaction.
- Scalability: The software should adapt to varying data loads and team sizes. Evaluate if the solution can grow alongside the organization, especially as data volumes increase over time.
- Security: In a landscape marked by data breaches, ensuring sufficient security measures are in place is non-negotiable. Check how data is stored, accessed, and protected.
- Support and Updates: Quality customer support and regular updates from the vendor signify a commitment to customer satisfaction and product improvement. An ongoing relationship with the vendor enhances long-term usability and reliability of the software.
- Cost: Assessing the total cost of ownership is essential. This includes licensing fees, training costs, and potential upgrade expenses. A solution should demonstrate a clear return on investment through improved efficiency and productivity.
By carefully considering these factors, organizations can create a solid foundation for selecting the right software solutions for their consulting needs.
The Role of Peer Reviews
Peer reviews are invaluable in the software evaluation process. These reviews provide insights that can be equally important as the technical specifications displayed by vendors. Relying solely on marketing materials from a vendor can present a biased view, so looking at independent sources becomes critical.
Through peer reviews:
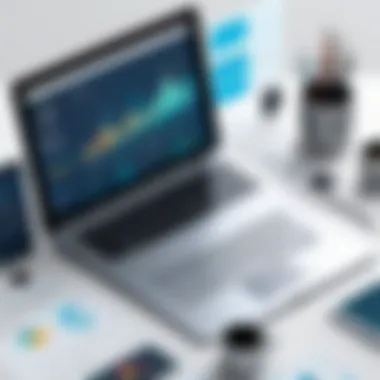
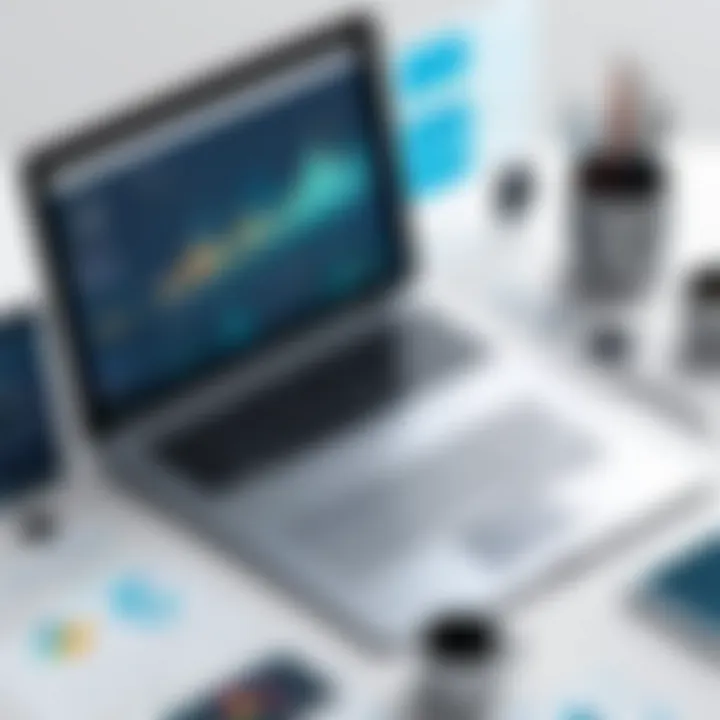
- Real Use Cases: Existing users share their experiences with the software, including both strengths and limitations. Having insights from actual users can clarify how well a solution might fit an organizationโs specific circumstances.
- Concept Validation: Peer reviews often touch upon not just whether the software performs, but how effective it is in real-world applications. Validation from other professionals can confirm whether product claims hold true in practical scenarios.
- Community Insights: Engaging with online platforms like Reddit or specialized forums can yield in-depth discussions on software capabilities and performance. Users can ask questions and receive feedback from a diverse audience, providing a broader understanding.
Incorporating peer reviews into the evaluation process complements more traditional methods, fostering a well-rounded assessment of potential software solutions.
Future Trends in Data and AI Consulting
In the rapidly evolving field of data and AI consulting, understanding upcoming trends is crucial for professionals aiming to maintain their competitive edge. Organizations that leverage the latest advancements can optimize their operations, enhance customer experiences, and make more informed decisions. Staying abreast of these trends allows businesses to align their strategies with technological innovations, ensuring they remain relevant and effective in a data-driven world. Here, we explore two key trends that are shaping the future of data and AI consulting: automation and cross-industry collaborations.
Emphasis on Automation
Automation stands at the forefront of the future of data and AI consulting. With the increasing demand for efficiency in business processes, automation technologies are becoming indispensable. By automating routine tasks, companies not only save time but also reduce the risk of human error. For instance, robotic process automation (RPA) can streamline data entry, data processing, and report generation, allowing human resources to focus on high-level strategic tasks.
The benefits of automation extend beyond mere efficiency. Organizations that adopt AI-driven automation can gain deeper insights through real-time data analysis. This capability allows businesses to identify trends and patterns quickly, facilitating proactive decision-making.
Furthermore, automation contributes to scalability. As businesses grow, manually managing immense volumes of data becomes increasingly impractical. Tools that automate data management can ensure that as the data landscape evolves, the organization can adapt without significant resource strain. However, companies must also consider the implications of automation on their workforce. Training and upskilling employees in collaboration with automated solutions is essential to maximize benefits while minimizing disruptions.
Cross-Industry Collaborations
In addition to automation, cross-industry collaborations are emerging as a vital trend in data and AI consulting. Sharing expertise across different sectors allows organizations to pool knowledge and resources, leading to innovative solutions that might not have been possible in isolation. For instance, a partnership between healthcare and tech firms could lead to the development of advanced predictive analytics for patient care, combining medical insights with cutting-edge technology.
The diversity of perspectives contributes to more robust problem-solving capabilities. Each industry brings unique challenges and approaches, fostering a multidisciplinary environment that drives creativity and innovation. Moreover, cross-industry collaborations can also enhance data sharing initiatives. When organizations collaborate, they can create a broader dataset for analysis, thereby improving the quality and relevance of insights derived from the data.
However, successful collaboration requires careful planning and clearly defined objectives. Organizations must ensure they establish trust and share compatible goals to maximize the benefits of working together. This careful approach is increasingly important in a world where data privacy and security are paramount.
"The future of data and AI consulting lies in embracing automation and fostering collaborations that transcend traditional industry boundaries."
To summarize, the emphasis on automation and the rise of cross-industry collaborations are pivotal to the future landscape of data and AI consulting. By actively engaging with these trends, professionals and businesses can retain their relevance and thrive amid the complexities of the modern data environment.
Case Studies in Data and AI Consulting
Case studies serve as a powerful tool in the domain of data and AI consulting. They provide real-world examples of how businesses have leveraged data-driven strategies and artificial intelligence to solve complex problems. By analyzing successful implementations and their impact, IT professionals, software experts, and business leaders can gain valuable insights into best practices and potential pitfalls.
Success Stories
Success stories illustrate how organizations have effectively utilized data and AI solutions. These case studies often highlight innovative approaches, showcasing the tangible benefits that arise from integrating these technologies into business operations. For example, a retail company might have implemented predictive analytics to improve inventory management, resulting in reduced costs and enhanced customer satisfaction.
Key elements of success stories include:
- Specific Outcomes: Clear metrics are often shared, showing improved revenue, operational efficiency, or customer engagement.
- Implementation Methods: Detailing the strategic processes followed during integration helps others assess applicability to their contexts.
- Challenges Faced: Acknowledging the hurdles faced during the implementation process offers a realistic view of what to expect.
Lessons Learned
Lessons learned from case studies emphasize the importance of reflection and adaptation in data and AI consulting. After a projectโs completion, the review process helps identify what strategies worked and what did not. This knowledge enables organizations to refine their approach in future endeavors.
Examples of important lessons include:
- Data Governance: Establishing robust data governance frameworks is crucial for maintaining quality and compliance.
- Stakeholder Engagement: Engaging key stakeholders throughout the project leads to greater alignment and better outcomes.
- Continuous Monitoring: Ongoing evaluation of implemented solutions ensures that they remain effective over time.
Lessons learned should guide future initiatives, making every case a stepping stone to further innovation.
Closure
In today's fast-paced business world, the realm of data and AI consulting has become integral for organizations aiming to thrive. This article has explored the numerous aspects of this specialized domain, highlighting its significance and multifaceted nature. By effectively leveraging data, companies can enhance their decision-making processes, drive innovation, and ultimately achieve a competitive edge.
Recapitulating Key Insights
The discussion covered various topics, from the importance of data as an asset to the role of artificial intelligence in promoting operational efficiency. One significant insight is the importance of data quality. Without ensuring accurate and reliable data, the effectiveness of any AI model or data-driven strategy falls short. Moreover, we examined the need for robust methodologies, balancing both traditional and agile approaches in consulting projects.
Several tools and technologies came under scrutiny as crucial enablers of effective data management and analytics. Software like Tableau for visualization and predictive analytics tools can substantially aid in translating raw data into actionable insights. The case studies illustrated these concepts, demonstrating real-world applications and their impact on business successes.
Call to Action for Professionals
For professionals in the field, the implications of the discussed insights are clear. Embracing a data-driven mentality is no longer optional; it is essential. Practitioners should prioritize continuous learning and improvement of their data analysis and AI capabilities. Engage in networking and share experiences with peers. This collaboration can lead to discovering innovative solutions and tools beneficial in various contexts.