Exploring the Depths of Snowplow Analytics for Insights
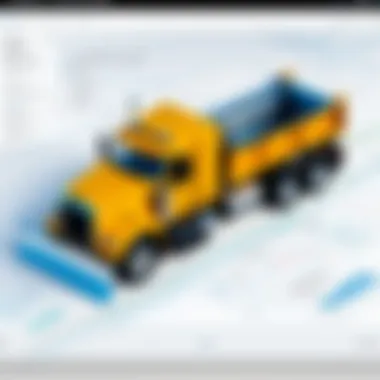
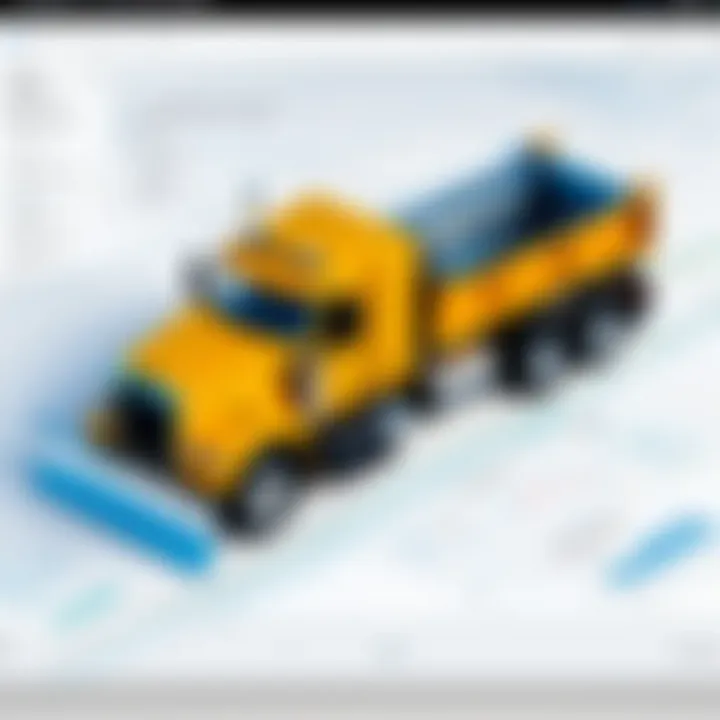
Intro
In the evolving world of data, businesses are constantly on the lookout for tools that offer deeper insights and streamline their analytical processes. Amidst this sea of options, Snowplow Analytics stands out as a compelling choice for companies looking to harness the power of their user interactions. This platform is not just another analytics tool; it provides a robust framework for collecting, processing, and analyzing data in real-time, making it an essential companion for organizations aiming to make data-driven decisions swiftly.
Overview of Software
Description of Software
Snowplow Analytics is an open-source platform specifically designed to help businesses capture and scrutinize their user data effectively. Starting from basic user interactions like clicks and views, it stretches to more complex event tracking, catering to diverse needs across various industries. Users can customize their data collection according to what matters most for their business, making this software particularly adaptable and user-friendly. The architecture facilitates not just capturing data but also processing it in an efficient manner, allowing insights to flow seamlessly.
Key Features
- Real-Time Data Processing: Snowplow provides capabilities for real-time analytics, which means businesses can act on insights as they emerge, rather than relying on lagging reports.
- Custom Event Tracking: It allows for tailored event data collection, accommodating unique business models and customer interactions.
- Integration Capabilities: Snowplow plays well with a multitude of data ecosystems, including data warehouses such as Amazon Redshift and Google BigQuery.
- Strong Data Governance: The platform emphasizes data quality and governance, ensuring that organizations can trust the insights derived from their data.
- Open-Source Flexibility: Being open-source, it grants organizations the freedom to modify and optimize their analytics according to specific requirements.
Software Comparison
Comparison with Similar Software
When placed alongside traditional analytics platforms like Google Analytics and Adobe Analytics, Snowplow reveals both strengths and weaknesses. Traditional solutions often offer user-friendly interfaces, requiring less technical prowess, making them accessible for small businesses or users with limited technical knowledge. In contrast, Snowplow’s setup might seem daunting initially, but it rewards users with unparalleled flexibility and customization in their data collection strategies.
Advantages and Disadvantages
**"Understanding both sides of the coin is crucial for informed decisions."
Advantages:
- Greater Customization: Snowplow allows users to tailor their data collection to their specific needs, rather than adhering to a one-size-fits-all approach.
- Comprehensive Data Insight: The ability to capture a wide array of events can lead to richer insights about user behavior.
- Robust Integration: It integrates well with existing cloud infrastructures, thus enhancing its utility in data-heavy organizations.
Disadvantages:
- Steeper Learning Curve: New users may find it challenging to get accustomed to its setup and configuration.
- Requires Technical Expertise: Ongoing technical support may be required to maintain an efficient setup, which could strain smaller teams.
In summary, Snowplow Analytics emerges as a powerful tool for those willing to invest the time and resources to leverage its full potential. It stands as both a challenge and an opportunity for tech-savvy professionals seeking to push the boundaries of their analytics capabilities.
Understanding the Fundamentals of Snowplow Analytics
In the fast-paced world of data analytics, understanding the fundamentals of Snowplow Analytics is crucial for businesses seeking to harness the full power of their data. This section provides the foundational aspects that set Snowplow apart from traditional analytics schemes, emphasizing its adaptability, precision, and comprehensive tracking capability. The modern enterprise works with vast amounts of data generated from various user interactions, making the ability to analyze and derive insights more important than ever. Snowplow offers a unique approach to this need, giving organizations tools for more granular and actionable insights.
Origins and Evolution of Snowplow
Snowplow Analytics originated from the need to address the limitations of conventional analytics tools, which often operate on a one-size-fits-all basis. The founders, having realized that standardized tracking methods were inadequate for diverse business models, set out to create an adaptable system. Initially designed to serve specific analytics needs for e-commerce, it quickly evolved into a more versatile framework capable of handling a wide array of use cases.
As industries evolved and digital interactions became more complex, so did Snowplow. Over the years, it has adapted to incorporate various data gathering methodologies, keeping up with technological advancements. This evolution allowed Snowplow to remain relevant as more businesses sought customized solutions, leading to its adoption across multiple sectors beyond just retail.
Core Principles of Analytics Tracking
The core principles that guide Snowplow's approach are vital for appreciating its strengths. Here are some key elements:
- Event-Level Tracking: Unlike many analytics tools that aggregate data at a high level, Snowplow allows for event-level monitoring. This means businesses can see every interaction, giving them detailed visibility into user behavior.
- Data Ownership: Snowplow promotes a strong sense of data ownership among clients. Users can control their data pipeline without being beholden to third-party tools, thus ensuring better compliance with data privacy regulations.
- Flexibility and Customization: Users can customize data collection, enabling tailored insights that align with their specific needs. This flexibility is particularly beneficial for businesses with unique operational workflows and customer interactions.
Through these principles, Snowplow Analytics empowers organizations not just to gather data, but to own it, understand it deeply, and draw actionable insights tailored to their strategic objectives.
"Data is not just a resource; it's an opportunity to create a competitive advantage."
In summary, understanding the fundamentals of Snowplow Analytics lays a strong groundwork for leveraging its full potential. With a focus on evolution, detailed tracking, and user empowerment, the platform emerges as a forward-thinking solution for contemporary analytics challenges.
The Architecture of Snowplow
The architecture of Snowplow is the backbone of its functionality, making it pivotal in the world of data analytics. Understanding how it all pieces together not only helps in appreciating its purpose but also highlights the advantages it brings to businesses seeking to leverage data. With an open-source model and a focus on expandable frameworks, Snowplow provides a robust architecture designed to capture, process, and analyze data seamlessly.
Components of the Snowplow Ecosystem
Snowplow operates through an intricate ecosystem that consists of several key components, each playing a crucial role in its architecture. The following elements stand out:
- Snowplow trackers: The initial point where user interactions occur. They capture events from websites or mobile applications and send them to the data collector.
- Data Collector: This component is responsible for receiving the event data from the trackers. It readily logs incoming data in bulk, minimizing the chances of data loss.
- Enrich: Next up is the enrichment process, which involves augmenting the raw data with contextual details, such as user attributes, geolocation, and more. This step is essential for meaningful analysis.
- Data Storage: Once enriched, the data can be stored in various databases, including Amazon S3 or Google BigQuery, making it readily available for future access.
- Data Models: After storage, data is molded into analytical structures that are suitable for querying and reporting, allowing businesses to extract insights efficiently.
Each of these components is modular, enabling users to customize their analytics solutions according to specific business needs.
Snowplow's Data Pipeline Structure
The data pipeline structure of Snowplow is engineered to uphold efficiency and reliability, preventing any bottleneck in data handling. Here’s how it flows:
- Event Tracking: User interactions trigger events intended for tracking.
- Data Ingestion: The event data is ingested into Snowplow servers efficiently, maintaining integrity.
- Data Enrichment: This step enriches data by adding vital information that transforms raw data into something valuable.
- Data Storage: The enriched data is stored in various formats, ensuring accessibility for further processing.
- Analysis and Reporting: Finally, the data is made available for analytical queries and visualization.
This streamlined pipeline structure supports both real-time and batch processing, accommodating the dynamic demands of contemporary businesses.
Payload and Event Tracking
In the context of Snowplow, payload and event tracking are critical aspects that enable detailed data collection. Each event can carry a payload that could include a multitude of elements, depending on what the business needs to measure. The structured nature of payloads allows for varied event types—ranging from page views to custom user interactions.
A well-structured payload leads to better data quality and more precise analytics. It's like putting your best foot forward each time you step into a conversation.
Moreover, event tracking in Snowplow is highly flexible. Businesses can define custom events tailored to their specific requirements. This flexibility enhances the granularity of data captured, offering more profound insights into user behavior and interactions. The combination of well-defined payloads along with diverse event tracking empowers organizations to make data-driven decisions with confidence.
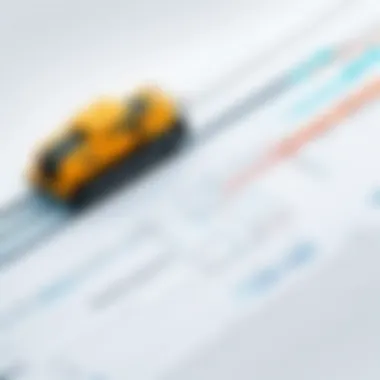
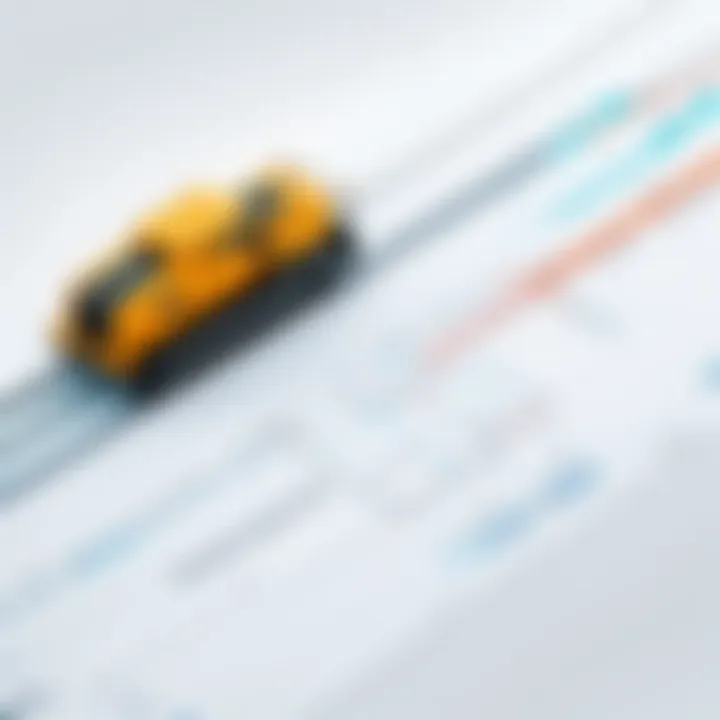
Clearly, the architecture of Snowplow represents a cohesive system that not only captures and processes data but does so in a way that supports advanced analytics and ultimately drives business growth.
Data Collection Mechanisms
Understanding Data Collection Mechanisms is essential for grasping how Snowplow Analytics operates. The way data is collected forms the backbone of analytics; without robust data collection, any analysis is just smoke and mirrors. Businesses today rely on data not just for numbers, but for actionable insights that can drive strategic decisions. That’s where effective data collection comes in—ensuring that raw data is captured accurately, consistently, and in a timely manner.
Client-Side vs Server-Side Tracking
When discussing client-side and server-side tracking, it’s crucial to comprehend the distinct implications each approach has on data integrity and performance. Client-side tracking captures data directly from the user’s browser or app. This method ensures that user interactions are recorded in real-time, providing immediate insights. However, it also carries the risk of data loss due to ad blockers or other browser settings that users might have in place.
On the other hand, server-side tracking processes data on the server, which can effectively bypass many of the limitations of client-side methods. This generally results in more controlled and reliable data collection as it is not subject to client-side conditions. However, it can introduce latency in data delivery since actions performed by users are not immediately dashed to the server. The choice between these two methods should be influenced by specific business needs:
- Client-Side Tracking:
- Server-Side Tracking:
- Pros: Instant data capture, detailed user behavior insights.
- Cons: Vulnerable to ad blockers, less control over data integrity.
- Pros: More reliable data capture, immunity to client-side blockers.
- Cons: Potential delay in data processing.
Ultimately, organizations might find a hybrid approach suitable, depending on their operational goals and the user experience they aim to provide.
Integrating Snowplow with Web and Mobile Apps
Integrating Snowplow with web and mobile applications is not just about connecting systems; it's about creating a cohesive ecosystem for data management. A well-crafted integration allows businesses to amass a wealth of data across different platforms, enriching their insights.
The integration process involves a few steps:
- Implementation of Tracking Code: Custom Snowplow trackers must be embedded within web and mobile apps. This code is vital as it allows data to be sent to the Snowplow data pipeline.
- Custom Event Definitions: Businesses should define metrics that are essential to their experience and objectives. Whether it’s user engagement or sales conversions, identifying what to track is critical.
- Testing and Validation: It's essential to conduct rigorous testing to ensure data is collected correctly. This involves checking that event data is flowing seamlessly into the Snowplow pipeline.
Some key considerations during integration:
- Cross-device Consistency: Ensuring user identity is maintained across devices can lead to a holistic view of user behavior.
- Data Privacy Regulations: Compliance with laws such as GDPR must be factored in during data collection and processing.
- Performance Impact: Integrating any tracking system should not adversely affect application performance; hence, optimizing the implementation is critical.
Effective integration of Snowplow with your platforms not only enhances analytical capabilities but also translates into more informed business strategies and decisions.
By establishing strong data collection mechanisms, businesses can set the stage for profound analytics, which can be transformative for their strategic initiatives. A leap towards data-driven growth is but an effective collection method away.
Processing and Enriching Data
Processing and enriching data is a pivotal step in the analytics pipeline, especially within the realm of Snowplow Analytics. By transforming raw data into insightful information, businesses can drive decisions that set them apart in a competitive market. This section examines how Snowplow manages its data processing and enrichment techniques to maximize the value of data collected from diverse sources.
Data Enrichment Techniques
Data enrichment is the process of enhancing collected data by integrating additional information from external sources. This is crucial because raw data often lacks context or actionable insights. Snowplow employs various enhancement techniques, allowing organizations to gain deeper perspectives on user behavior and interactions.
One common method is contextual enrichment, where additional user details, such as demographic information or geographic location, are appended to the original events. For example, if a user clicks on an advertisement, Snowplow can provide insights into where that user is located, their age, or even their favorite products. This enables businesses to create targeted marketing strategies based on real and relevant data.
Another technique is combining multiple data sources through a method known as join enrichment. Imagine a scenario where a website's shopping data can be matched with CRM data. Here, insights regarding customer preferences and purchase histories become clearer, providing a holistic view of customer engagement.
Benefits of Data Enrichment:
- Deeper Insights: It allows companies to understand not just what people do but why they do it.
- Improved Segmentation: Enhanced data leads to better target audience segmentation, thus refining marketing campaigns.
- Predictive Analytics: Combining enriched data with analytical tools increases the potential for predictive modeling, paving the way for smarter decisions.
Real-time Data Processing Capabilities
In today's fast-paced business environment, the ability to process data in real-time is indispensable. Snowplow provides a robust architecture that supports real-time data processing, allowing organizations to act on data as it is generated. This capability is crucial for businesses looking to maintain a competitive edge in their respective markets.
The real-time processing feature of Snowplow is facilitated by its data pipeline, which captures events and processes them immediately. That means when a user interacts with a web page, the data can be analyzed right away to reflect that interaction. Picture this: a potential customer browses a website, adds items to their cart, but does not complete the purchase. Real-time data processing enables the application to trigger immediate follow-up actions, such as sending a reminder email or displaying targeted ads, preventing potential revenue loss.
Moreover, the architecture supports low-latency processing, ensuring businesses can receive insights almost instantaneously.
“Real-time data processing with Snowplow allows merchants to react swiftly to customer behaviors, enhancing user experience and driving sales.”
Considerations for Real-time Processing:
- Infrastructure Requirements: Organizations need to ensure that their infrastructure can handle streams of incoming data without bottlenecks.
- Data Privacy: Real-time processing must be aligned with data governance policies to maintain user trust and comply with regulations.
- Cost Implications: While instant insights are invaluable, the associated costs of real-time systems should be weighed against potential ROI.
In summary, both processing and enriching data are essential elements of Snowplow Analytics. With effective enhancement techniques and real-time processing capabilities, businesses can unlock the full potential of their data, making informed decisions that lead to growth and improved user engagement.
Technical Implementations
In the realm of Snowplow Analytics, the aspect of technical implementations can’t be stressed enough. It serves as the backbone supporting the collection, processing, and analysis of massive troves of data. This section aims to shed light on the critical elements involved in deploying and scaling Snowplow solutions, emphasizing their significance in harnessing analytics capabilities.
Deploying Snowplow on Cloud Platforms
When it comes to deploying Snowplow, cloud platforms like Amazon Web Services (AWS), Google Cloud Platform (GCP), or Microsoft Azure play a pivotal role. They provide the necessary infrastructure, allowing businesses to set up a scalable and flexible Snowplow environment.
- Benefits of Cloud Deployment
- Scalability: It allows organizations to rapidly adjust resources in accordance with evolving data needs. If your user interactions spike suddenly, your cloud resources can expand in tandem.
- Cost-Effectiveness: Pay-as-you-go pricing models mean companies only pay for what they use. This is especially beneficial for startups and small businesses that might not have a hefty budget.
- Accessibility: Cloud solutions enhance collaborative efforts. Teams across different geographical locations can connect to a centralized analytics platform seamlessly.
To implement Snowplow effectively in cloud environments, one must undertake several steps:
- Setting Up the Infrastructure: Initiate services within your chosen cloud platform to create storage resources, compute instances, and necessary networking configurations.
- Configuring Components: Properly set up Snowplow components like collectors and enrichers. These components must communicate effectively within the cloud infrastructure to ensure data streams without interruption.
- Establishing Security Protocols: Secure your analytics pipeline through data encryption and following best practices for cloud security.

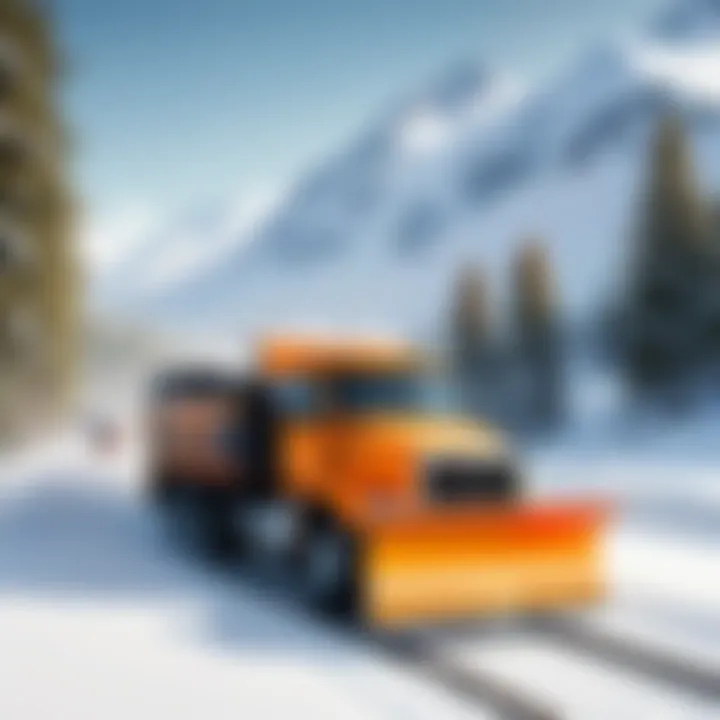
The deployment on cloud platforms can seem daunting but adapting to the unique requirements of an organization can lead to effective implementations that propel data analytics forward.
Scaling Snowplow Solutions
Once Snowplow is up and running, the next logical step is scaling its solutions. Growing businesses often experience dynamic changes in data volume, requiring an analytics framework that keeps pace.
Scaling is vital for several reasons:
- Handling Increased Data Loads: Traffic spikes, seasonal fluctuations, or marketing campaigns can cause dramatic surges in data that must be captured and processed efficiently.
- Enhancing Data Processing Speed: A scaled solution improves the speed of data collection and reporting, something critical for businesses seeking rapid insights.
- Flexibility Across Applications: As organizations integrate more systems, scaling ensures that Snowplow can gather data from various sources without breaking a sweat.
To effectively scale Snowplow, consider the following strategies:
- Load Balancing: Distributing incoming data loads across multiple instances can prevent bottlenecks and ensure stable performance.
- Microservices Architecture: Implementing Snowplow in a microservices setup allows for individual components of the analytics pipeline to scale independently, ensuring that each part meets demand without impacting the others.
- Optimizing Storage Solutions: As data grows, consider employing data lakes or optimized database solutions that better handle large datasets compared to traditional databases.
Scaling is not merely about adding resources; it’s about intelligently allocating them and ensuring your Snowplow instance is ready for the future.
Integration with Other Data Tools
Connecting Snowplow Analytics with other data tools is a critical aspect for organizations looking to leverage their data more effectively. The ability to integrate with a variety of data ecosystems can substantially enhance how insights are drawn and utilized. This integration not only allows for a more comprehensive view of customer interactions but also fosters operational efficiency and decision-making agility. Companies can harness the full power of their data, marrying it with tools that offer advanced analytical capabilities, reporting, and visualizations.
Connecting Snowplow with Data Warehouses
Data warehousing is a crucial component in the realm of analytics. Integrating Snowplow with data warehouses like Amazon Redshift, Google BigQuery, or Snowflake empowers businesses to store vast amounts of data efficiently while maintaining quick access for queries. This relationship allows for seamless transitions from raw data to processed insights, enabling sophisticated analysis and reporting.
By establishing a conduit between Snowplow's rich dataset and a data warehouse, organizations can perform tasks such as:
- High-Performance Queries: Employ powerful querying capabilities that can process large datasets without suffocating performance.
- Aggregated Reporting: Generate reports that combine user interaction data with sales, inventory, or other relevant metrics.
- Historical Analysis: Store and access long-term data trends, assisting in forecasting and strategic planning.
This integration also encompasses considerations such as data schema design and ETL (Extract, Transform, Load) processes. Ensuring that data flows efficiently without bottlenecks is vital for maintaining accuracy and reliability. So, companies looking to take this route must embrace a structured approach to implementing connections, addressing any potential friction points.
Leveraging BI Tools with Snowplow Data
Business Intelligence (BI) tools have gained momentum as indispensable resources for data-driven organizations. Tools such as Tableau, Power BI, and Looker enable users to draw insights from Snowplow data without necessitating deep technical expertise. By connecting Snowplow's analytics data with these BI platforms, businesses can create visualizations and dashboards that transform raw data into digestible insights.
Here are some solid benefits of leveraging BI tools with Snowplow data:
- Visual Data Representation: BI tools can turn complex datasets into intuitive visuals, making it much easier for stakeholders to understand analytics at a glance.
- Customization Options: These tools offer extensive customization for users to tailor reports and dashboards that reflect specific business needs and KPIs.
- Per-User Access Control: Organizations can easily manage who sees what data, enhancing security and ensuring team members have access to the information most relevant to them.
Moreover, integrating Snowplow with BI tools can lead to a more collaborative analysis process where teams can share insights and visual representations effectively. Often, the workflow is enhanced, making it easier to engage with data.
It's worth mentioning that while the integration process is often seamless, it is crucial to ensure data formatting aligns, and the data being pushed to BI tools is clean and enriched to maximize its value.
“Connecting Snowplow to your data ecosystem is not just an add-on; it's about forging deeper insights from your analytics, empowering better decisions.”
Advantages of Using Snowplow Analytics
Snowplow Analytics stands out in the crowded field of data collection and processing platforms. Its numerous advantages over traditional analytics solutions make it a compelling choice for businesses looking to supercharge their data strategies. Understanding these benefits is crucial not just to make an informed decision, but to leverage Snowplow’s capabilities for maximum impact. Two of the most significant advantages include the platform's customization and flexibility, along with improved data ownership and control.
Customization and Flexibility
One of the standout features of Snowplow is its ability to be tailored to meet the specific needs of a business. Unlike more rigid analytics solutions, Snowplow offers a customizable framework that allows organizations to define their tracking parameters and data models. This is important because different businesses have diverse requirements.
- Adaptability: Companies can track specific events relevant to their operations, from user interactions on their webpages to transactions in their apps. This adaptability ensures that the data collected is not only plentiful but also pertinent.
- Schema Evolution: Snowplow supports schema evolution, which enables businesses to adjust their data structures over time without the worry of breaking existing setups. This is particularly useful as businesses grow or pivot their strategies.
- Custom Events: Users define custom events, meaning a business can capture niche interactions that standard solutions may overlook. For instance, a gaming company can track unique player actions or, in an e-commerce context, a business can monitor the behaviors of customers during checkout.
The flexibility Snowplow offers results in richer data that aligns closely with a company’s specific analytical goals.
Improved Data Ownership and Control
Data ownership is becoming increasingly important in a world where privacy regulations are tightening, and businesses are held accountable for the data they collect. Snowplow provides significant advantages in this regard:
- Full Control Over Data: Unlike many cookie-cutter solutions, Snowplow allows businesses to own and control their data entirely. This means organizations can guarantee compliance with data privacy laws, such as GDPR, which may not be possible with more traditional platforms.
- Data Pipeline Transparency: Snowplow's architecture allows for complete transparency in how data flows through the system. Organizations can monitor and audit their data collection processes, ensuring that everything is operating as intended.
- Self-Reliance: Businesses reduce their dependency on third-party analytics vendors, which can lead to unexpected fee increases or changes in service. With Snowplow, they own the infrastructure, giving them the freedom to adjust and expand without external limitations.
"In today's data-driven world, having control over your data is equivalent to having power in your business strategy. Snowplow not only provides that power but also allows you to mold it to your advantage."
Common Use Cases
Common use cases of Snowplow Analytics highlight its remarkable adaptability across different sectors. Understanding these applications can shine a light on the benefits and considerations of integrating this powerful data tool into various business strategies. By recognizing how others have leveraged Snowplow, organizations can form clearer expectations and better-informed decisions, particularly around analytics goals and technical implementations.
E-commerce and Retail Analytics
The e-commerce sector stands to gain immensely from Snowplow's rich data tracking capabilities. In today’s market, where every click counts, businesses need insights that drive customer engagement and conversion rates. With Snowplow, companies can track user behavior on their websites—from the moment a potential customer lands on the homepage to the final point of purchase.
Here's how Snowplow empowers e-commerce analytics:
- Granular Data Capture: Snowplow allows businesses to capture detailed event data. This includes page views, clicks, add-to-cart actions, and checkout completions. This granularity helps retailers understand the customer journey in-depth.
- Customer Segmentation: Tailoring offerings to meet specific customer demands becomes more manageable. By analyzing behavior patterns, businesses can identify different customer segments and target them effectively.
- Enhanced Personalization: Harnessing data from Snowplow, e-commerce businesses can present personalized recommendations to users. This can significantly increase overall sales and customer satisfaction.
- Optimized Marketing Strategies: Analyzing conversion paths enables businesses to determine the most effective channels for marketing efforts, leading to better ROI.
Utilizing Snowplow in this context equips e-commerce players with the tools necessary to navigate customer preferences dynamically.
Marketing Performance Analysis
In the fast-paced world of marketing, being able to rapidly assess campaign effectiveness can make the difference between success and shortcomings. Snowplow's versatility extends into this arena, making it a tool of choice for marketers looking to evaluate and iterate on their strategies.
Here are key advantages:
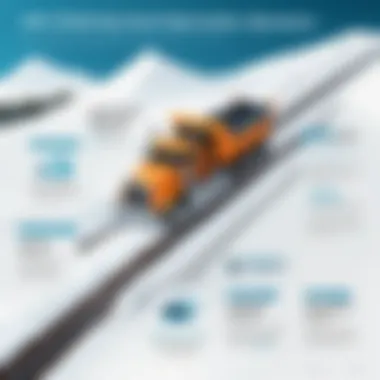
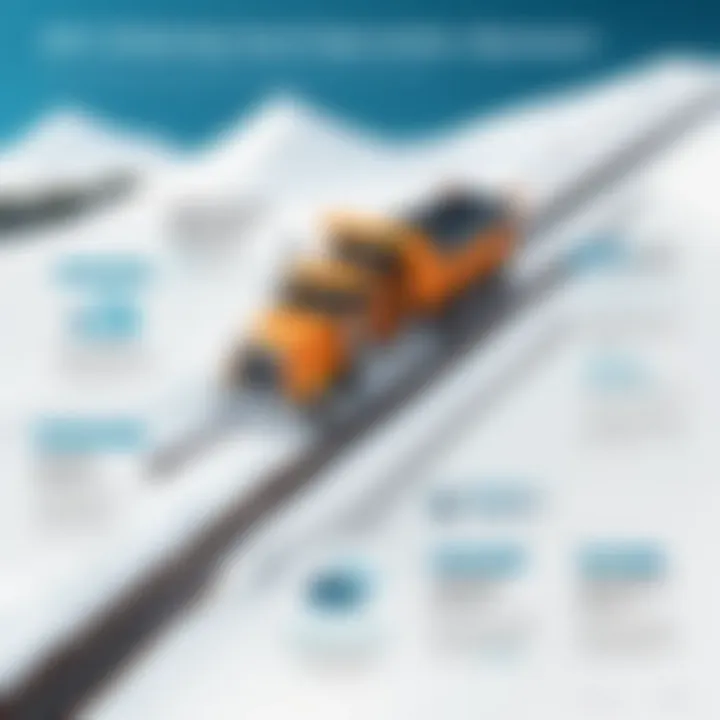
- Real-Time Tracking: With Snowplow, marketers can monitor campaign performance as it happens. This immediate feedback loop sharpens their ability to adjust campaigns on the fly, whether that involves altering messaging or reallocating budget.
- Holistic Insights: By integrating Snowplow with marketing tools, organizations can fuse CRM data with user behavior insights. This creates a comprehensive view of how marketing initiatives influence customer interactions.
- Attributing Success Accurately: Snowplow facilitates detailed attribution modeling. By tracking multiple touchpoints, businesses can identify which campaigns are driving desired actions most effectively.
- Test and Learn Approach: Organizations can conduct A/B testing seamlessly, ensuring they can identify which elements of a campaign resonate best with customers.
"The best marketing doesn't feel like marketing." - Tom Fishburne
Thus, for marketers dissecting the impact of their strategies, Snowplow provides the depth of analysis required to continuously improve performance while staying connected to business objectives.
These common use cases—e-commerce optimization and marketing intelligence—provide a solid foundation for understanding how Snowplow Analytics can be leveraged effectively. As companies in these fields recognize the potential, they are better equipped to navigate an increasingly complex data landscape and craft strategies that resonate with their audiences.
Challenges and Limitations
In the context of deploying Snowplow Analytics, understanding its challenges and limitations is crucial for IT and software professionals as well as businesses seeking data-driven solutions. While Snowplow offers a robust platform for tracking user interactions and processing data, navigating through its complexities can be a tall order. Identifying these challenges does not only prepare organizations but also helps to set realistic expectations when integrating this analytics solution.
Implementation Complexity
Getting Snowplow off the ground requires a fair bit of technical know-how. Implementing Snowplow’s architecture demands familiarity with various components such as trackers, enrichers, and storage solutions. Without adequate technical expertise, businesses can easily feel like they’re trying to climb a mountain without the right gear. The installation often involves:
- Design of a proper data model that suits specific business needs
- Configuration of multiple integrations, be it with cloud storage like Amazon S3 or processing platforms like Apache Kafka
- Setting up various tracking mechanisms including custom events, page views, and user identification
This complexity can lead to longer timelines for actual implementation. Organizations must have not just the right tools but also skilled personnel available. If you are a small business owner, diving into Snowplow might feel like stepping into a lion's den without proper shielding. As daunting as it may sound, developing a well-planned roadmap can significantly ease this process.
Data Governance and Compliance Issues
In today's data landscape, understanding data governance is like having a road map when you are lost. Snowplow can handle vast amounts of data seamlessly, but this capability comes with the caveat of ensuring compliance with regulations such as GDPR or CCPA. Failure to comply can lead to hefty fines and reputational damage. Key considerations include:
- User consent: With strict regulations on user data collection, ensuring that consent mechanisms are in place is paramount.
- Data retention policies: Organizations must carefully manage how long they keep their data and under what circumstances it can be deleted.
- Data security: Businesses need to implement security measures to protect sensitive information obtained through Snowplow from breaches.
As data becomes more central to operations, navigating the intricacies of data governance is not just a technical obstacle but a business imperative. Organizations that get a handle on these complexities will better position themselves to harness the full potential of Snowplow Analytics while staying compliant.
"In a world inundated with data, mastering governance is as important as leveraging the insights gleaned from it."
Tackling these challenges head-on requires sound strategies, expert knowledge, and a commitment to maintaining a compliant data ecosystem. Snowplow Analytics, with its capability for custom tracking and processing, can indeed throw businesses a lifeline toward actionable insights. However, understanding these challenges is what empowers them to navigate the waters effectively.
Market Position and Competitive Landscape
In the current digital landscape, understanding the market position of Snowplow Analytics is pivotal. The platform occupies a unique niche in the analytics ecosystem, providing businesses with the tools to control their data collection and analysis in a manner that many traditional analytics platforms cannot. Its flexibility allows organizations to adapt their tracking according to evolving business needs.
Snowplow's architecture supports distinct advantages over competitors. For instance, the open-source nature of its platform permits users to customize and extend its capabilities, a feature that means a lot in dynamic business environments. Moreover, Snowplow promotes data ownership; unlike certain analytics solutions, users maintain full control over how data is processed and utilized. This aspect is becoming increasingly essential in light of rising privacy concerns and regulations like GDPR.
Comparative Analysis with Other Analytics Platforms
When pitting Snowplow against established players like Google Analytics or Adobe Analytics, it quickly becomes apparent that there is a stark contrast in how data is approached and leveraged.
- Customization: Snowplow allows extensive customization of event tracking, enabling businesses to track specific user interactions tailored to their objectives. Options are near endless here compared to the more rigid frameworks of other platforms.
- Data Processing: Snowplow processes data in real-time, unlike many competitors which process data in batches. This immediacy opens up opportunities for timely decision-making.
- Cost Efficiency: Many traditional analytics platforms can require hefty licenses or usage fees. Snowplow's architecture can be deployed on cost-effective infrastructure, potentially resulting in lower total costs for businesses.
"Snowplow Analytics not only offers a comprehensive set of capabilities but also empowers users through ownership and flexibility, setting it apart from conventional options in analytics."
Beyond features, businesses must also consider the learning curve. Some platforms, while feature-rich, can alienate users unfamiliar with their technicalities. Snowplow, while powerful, may necessitate a bit more technical know-how during setup. However, once established, users can harness its full potential without the weight of limitations.
Emerging Trends in Analytics Solutions
Looking forward, the analytics landscape is on the cusp of change, influenced by innovative technologies and methodologies. Snowplow is well-positioned to capitalize on these trends.
- Integration with Machine Learning: The incorporation of artificial intelligence into analytics tools is not just a trend; it’s fast becoming the norm. Snowplow can seamlessly integrate with machine learning algorithms, allowing for complex predictive analytics models that can forecast user behavior based on historical data.
- Rise of Decentralized Data Policies: As organizations face increasing scrutiny regarding data privacy, decentralized data handling will become paramount. Snowplow’s architecture aligns nicely with this trend, allowing businesses to manage their data more securely and transparently.
- Event-Driven Analytics: As businesses adopt a more user-centric approach, the demand for event-driven insights is mushrooming. Snowplow’s data collection methods are geared towards capturing detailed user interactions, thus empowering organizations to derive meaningful conclusions from even the minutiae of user engagement.
As these trends evolve, Snowplow's foundation will allow it to adjust and rise to meet changing demands, offering unique solutions where other platforms may falter.
Future Directions
As we navigate the rapidly changing landscape of data analytics, understanding the future directions of Snowplow Analytics becomes crucial for businesses wanting to remain ahead of the curve. The significance of this topic lies not just in adopting new technologies but in recognizing how these advancements can profoundly impact decision-making, operational efficiency, and strategic planning.
The Role of AI in Analytics
Artificial Intelligence (AI) is swiftly becoming a game-changer in the world of data analytics. Organizations are harnessing machine learning algorithms and advanced analytical frameworks to automate processes, identify trends, and unearth insights faster than ever. For Snowplow, integrating AI capabilities could mean:
- Enhanced Predictive Analytics: Businesses could leverage AI models to forecast user behavior and trends based on historical data, allowing for more informed decision-making.
- Automated Data Enrichment: AI can take on the burden of enriching raw user data, providing deeper insights with minimal human intervention.
- Anomaly Detection: With powerful algorithms, Snowplow could help identify irregular patterns in data that might otherwise go unnoticed, saving companies from potential errors.
In essence, the marriage of AI and analytics is a significant evolution. It offers not only efficiency but also the potential for profound insights, transforming how organizations understand their data.
Predictions for the Evolution of Snowplow
The evolution of Snowplow Analytics is likely to follow a few key trajectories. While it's hard to predict the future with certainty, certain trends stand out based on emerging technologies and changing market demands:
- Broader Integrations: Snowplow will likely enhance its compatibility with various third-party tools and services, allowing seamless data flow across platforms, thus creating a more holistic view of user engagement.
- Greater Focus on Privacy: As regulations like GDPR become more prevalent, Snowplow may adapt by building in more robust privacy-focused features, allowing businesses to better manage user data while complying with legal requirements.
- Expansion into Real-Time Analytics: Businesses increasingly rely on immediate data for decision-making. Snowplow could expand its capabilities in real-time analytics, providing users with instant reporting and actionable insights.
- Community-Driven Enhancements: With an active open-source community, the Snowplow ecosystem might see innovations generated not just by developers but by a broad range of contributors, making the platform continuously adaptive to user needs.
These predictions underscore the importance of remaining vigilant and adaptive in a world where data and technology are constantly in flux. Companies that choose to invest in these future directions will likely find themselves at the forefront of effective analytics capabilities that can drive significant business growth.
End
In wrapping up this exploration of Snowplow Analytics, it’s vital to underscore the enormous significance of leveraging this platform in today’s data-driven landscape. Snowplow is more than just an analytics tool; it’s a comprehensive ecosystem that facilitates deeper understanding and control over data collection and processing. For IT professionals and businesses, the importance of this kind of robust analytics cannot be overstated.
Key Takeaways from Snowplow Analytics
- Customization and Flexibility: Unlike many off-the-shelf analytics solutions, Snowplow allows for tailored tracking setups. Businesses can define their own data points and attributes which makes it very flexible. This ensures that the exact metrics which matter to an organization are captured.
- Real-time Processing: The capability for real-time data processing means that decision-makers are never out of touch with current user interactions. This immediacy allows businesses to react swiftly to changes in user behavior, exemplifying the phrase "time is money."
- Data Ownership: Organizations that utilize Snowplow benefit from complete data ownership. Control over data means that businesses are not at the mercy of third-party platforms for insights, promoting a sense of independence and security on their journey towards data empowerment.
- Integration Capability: Snowplow's architectural framework allows seamless integration with popular tools like BigQuery or Snowflake. This integration fosters a deeper analysis, enabling teams to pull in diverse datasets and draw more nuanced insights from their analytics efforts.
Things to Consider
- Implementation Complexity: While the advantages are clear, the learning curve can be steep. Organizations must have the right resources and expertise to fully harness Snowplow, making initial setup a potential hurdle.
- Data Governance: As businesses funnel more data through Snowplow, maintaining compliance with data governance and regulatory requirements becomes crucial. Companies must adopt best practices to ensure they’re protecting user information while leveraging analytics.
In summary, the conclusion of this article highlights that adopting Snowplow Analytics presents businesses with a golden opportunity to refine their data strategies. The combination of flexibility, control, and real-time insight positions organizations to thrive in competitive markets. For further reading, consider exploring resources on Wikipedia or discussions on Reddit.
"Data may be the new oil, but not all oil wells yield the same output. With Snowplow, you’re digging deeper into the right wells."