Top Minitab Alternatives: Features and Benefits
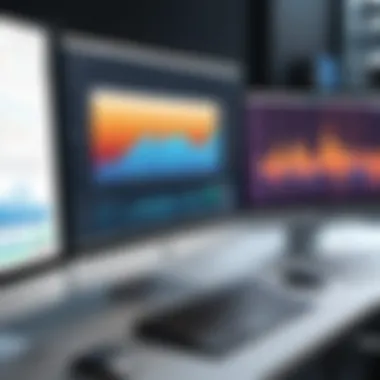
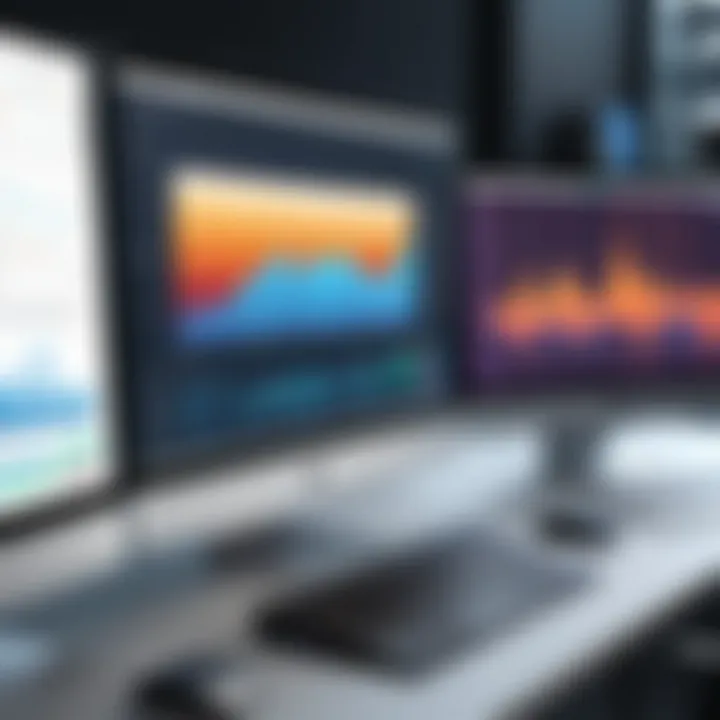
Intro
In today's data-driven world, the need for comprehensive data analysis tools has surged. While Minitab has established its significance in this domain, analytically-minded professionals often crave alternatives that may better suit their specific needs.
This article dissects various statistical software options that stand as viable competitors to Minitab, each offering unique features and capabilities. We aim to cater to a diverse audience, spanning IT professionals, academic researchers, and small to large business entities, ensuring that every reader walks away with insightful information to assist their decision-making processes.
Statistical analysis is a craft that requires both precision and a deep understanding of the tools available. By exploring alternatives, we do not only highlight comparable software but also deepen our understanding of how these tools address specific analytical challenges.
As we proceed, we will critically assess these options, shedding light on their functionalities, strengths, and weaknesses. This analytical journey lets stakeholders evaluate the landscape of data analysis tools with an informed lens.
Let’s dive into the details to discover the most fitting alternatives to Minitab.
Understanding Minitab and Its Popularity
Minitab has carved out a significant place in the landscape of data analysis. It's favored for several reasons, all intertwined with its ability to make complex statistics accessible to a broad audience—from seasoned statisticians to those who are just starting. At its heart lies a user-friendly design.
When people think of crunching numbers, they often think of Minitab first. This isn’t merely because it’s been around for a while; its reputation stems from reliable performance in statistical analysis, especially in quality improvement processes. Many industries, particularly manufacturing and education, have woven Minitab into their daily operations, thanks to its robust suite of tools.
The Role of Minitab in Statistical Analysis
Minitab serves as a cornerstone in the statistical analysis domain. It allows users to perform a wide range of statistical tests, from descriptive statistics to advanced regression analysis. This flexibility makes it an appealing choice for professionals aiming to make data-driven decisions. Moreover, the software supports Six Sigma methodologies, making it indispensable for quality assurance experts.
This accessibility encourages a hands-on approach to learning statistics. It also empowers professionals to translate their data into actionable insights—a necessity in today’s data-driven world. In fact, many educational institutions use Minitab in their curriculums. This exposure further fuels its popularity, as graduates often seek it in the workplace when looking for statistical tools they already know how to use.
Industry Applications of Minitab
Minitab finds significant traction across various industries:
- Manufacturing: Here, it plays a pivotal role in quality control, using tools like Control Charts and Process Capability Analysis to maintain production standards.
- Healthcare: Hospitals and clinics utilize Minitab to track patient outcomes and improve service quality through statistical process control.
- Education: Many colleges teach students statistical methods via Minitab, allowing them to apply theoretical knowledge in practical scenarios.
These applications underscore Minitab's adaptability and efficacy, making it a go-to choice across different fields.
Limitations of Minitab
Despite its strengths, Minitab isn't without its drawbacks. A few limitations make some professionals seek alternatives. First, the cost can be a significant barrier. While it might be affordable for larger organizations with a hefty budget, small businesses or individual users might find the expense steep compared to free or open-source alternatives.
Additionally, some users express frustration with Minitab's limited capabilities in certain advanced analyses, especially in machine learning applications. Also, as more data scientists shift toward programming languages like R or Python, Minitab's market share may dwindle among more technically inclined users. It’s crucial to weigh these aspects against its benefits when considering Minitab for data analysis tasks.
"While Minitab is a powerhouse for statistical analysis, the cost and certain limitations may drive users to explore a broader toolbox of alternatives."
Navigating the intricacies of data analysis involves understanding both Minitab's strengths and weaknesses. Recognizing this balance is essential for any professional looking to make informed choices in statistical software.
Criteria for Evaluating Minitab Alternatives
When considering alternatives to Minitab for data analysis, it’s crucial to evaluate several key criteria. These factors significantly influence both the practical use and overall efficiency of the alternative software. By assessing options through these lenses, users can better align their tools to their specific needs.
Usability and User Experience
Usability refers to how user-friendly the software is. A product could be feature-rich, but if it takes a PhD to figure it out, it won't be helpful in real-world applications. Professionals often have tight deadlines, so a tool that is straightforward and intuitive can save precious hours. Look for features like drag-and-drop functionality, interactive dashboards, and clear navigation. The learning curve can vary notably across different platforms.
User experience often ties back to the interface. Is it cluttered or clean? Captivating layouts can enhance productivity while overwhelming ones can frustrate even the most seasoned analyst. User reviews can serve as valuable resources to gauge overall satisfaction; they often reveal issues not found in promotional materials.
Cost Considerations
Cost is a major decision-making factor when selecting an alternative to Minitab. Some options are free, while others can escalate into significant investments. It’s important to consider not just the initial software cost, but also ongoing expenses like maintenance, updates, and additional modules.
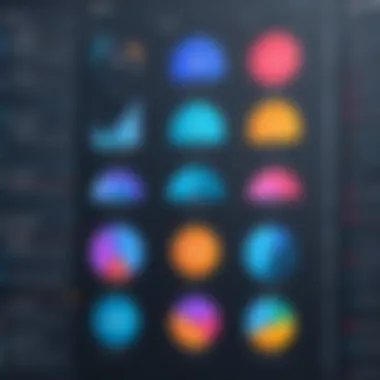
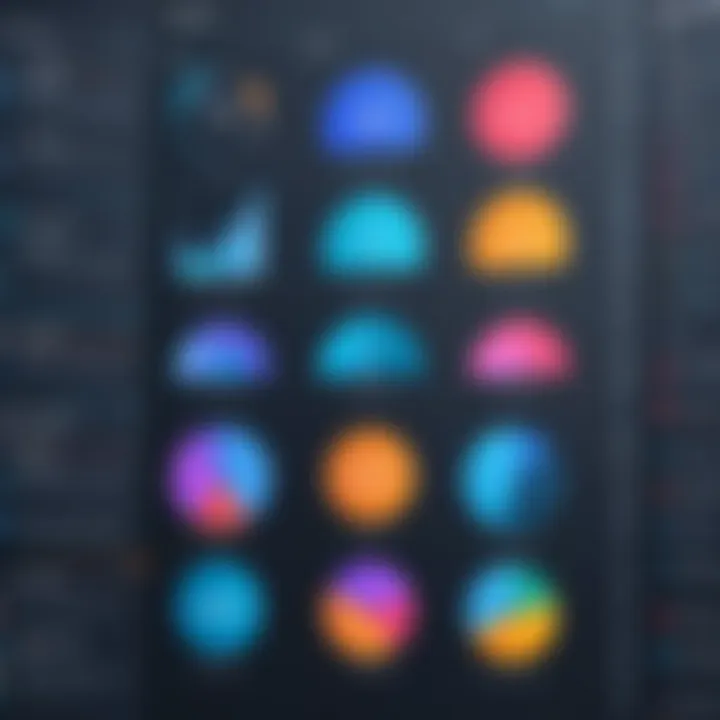
- Free Alternatives: Open-source tools like R come at no cost, enabling startups and smaller businesses to access sophisticated analysis without breaking the bank.
- Subscription Models: Software such as SPSS may offer a monthly subscription, allowing flexibility but could add up significantly over time.
- Total Cost of Ownership: Don’t forget to factor in training costs, potential hiring of external consultants, and time investments for onboarding.
Weighing these financial implications against the functionality and benefits the software provides is key to finding a cost-effective solution.
Functionality and Features
The heart of any statistical software lies in its functionality. This aspect includes not just the ability to perform basic statistical analyses but also advanced functionalities such as machine learning capabilities, regression analysis, and predictive modeling. Are there built-in templates for common analysis tasks? Can it handle large datasets seamlessly?
Moreover, consider whether the software integrates well with other tools in your tech stack. Compatibility with programming languages like Python or R may enhance overall capabilities, allowing for extended custom analysis. Also, don’t overlook the importance of visualization features, which can turn complex data into easily digestible formats.
Support and Community Resources
Having solid support and community resources can make all the difference when struggling with software. Comprehensive documentation, tutorials, and active user communities can ease the transition to a new tool. Within the realms of data analysis, community forums like Reddit allow users to share insights, troubleshoot, and exchange strategies. Moreover, a responsive customer support team can quickly resolve issues that might hamper workflow.
Good alternatives often have a blend of official training resources and user-generated content available online. This is particularly noticeable with popular tools like Python’s libraries—where communities thrive on sharing tips, example projects, and troubleshooting.
In essence, it is vital to select software not just based on features, but also based on available support and educational resources to nurture an effective user experience.
Key Alternatives to Minitab
In the quest for effective data analysis, exploring alternatives to Minitab becomes not just beneficial but essential. Each software alternative brings its own set of strengths and features that may align better with specific project needs, budget constraints, or user preferences. Below, we dig into several noteworthy alternatives that can cater to a variety of analytical requirements while keeping the intrinsic needs of IT and software professionals in mind.
R: An Open-Source Paradigm
R has garnered considerable attention as a leading open-source option for statistical computing and graphics. One of its main strengths lies in its flexibility. Users can create custom statistical models tailored to their specific project requirements. A vast ecosystem of packages, like ggplot2 for data visualization and dplyr for data manipulation, allows users to extend functionality easily. The community around R thrives, with forums and resources readily available to assist users. However, the steep learning curve associated with R might deter the faint-hearted, as it typically requires a certain level of programming knowledge to harness its full potential.
Python with Pandas and SciPy
Python stands tall, especially in the realm of data science, thanks to libraries like Pandas and SciPy. Pandas is perfect for handling data in a tabular format, supporting a plethora of data formats and offering tools for easy manipulation. When combined with SciPy, users gain access to powerful tools for numerical integration, optimization, and statistical analysis. Python's general-purpose nature further empowers it, making it suitable for both data analysis and other programming tasks. That said, its broad applicability means users might need additional time to set up the environment and understand its libraries fully.
SPSS: A Classic Choice
SPSS remains a solid contender in the lineup of statistical software, particularly favored in fields like social sciences. Its user-friendly interface makes tasks straightforward, allowing users to focus on analysis without wrestling with complex coding. SPSS is tailored for a range of statistical manipulations, from simple descriptive statistics to complex multivariate analysis. Despite its numerous advantages, prospective users should note that licensing fees can escalate considerably, which may not be suitable for all budgets.
SAS: Enterprise-Level Functionality
SAS is often synonymous with enterprise-grade statistical analysis. With its extensive capabilities in advanced analytics, business intelligence, and data management, it’s a go-to for larger organizations aiming for robust data solutions. SAS excels in handling large datasets and performing predictive analytics, but its cost can be prohibitive for smaller outfits. The software’s sophisticated features necessitate significant training, so companies should be prepared to invest in user education.
Statgraphics: Comprehensive Toolset
Statgraphics offers a rich set of tools for both graphical and statistical analysis. Its strength lies in combining ease of use with powerful statistical capabilities, appealing to both novice and experienced users. One remarkable feature is its ability to create a wide variety of plots and graphs, which enhances the visual presentation of analyzed data. That said, Statgraphics might feel less intuitive than some competitors, requiring a learning phase to navigate its options and menus effectively.
JMP: Dynamic Data Visualization
JMP, a product of SAS, prioritizes interactive visualization, making it easier for users to understand their data on a deeper level. Users can explore data relationships dynamically, shifting their focus as new variables are introduced. This unique ability allows analysts to interpret data findings quickly, promoting efficient decision-making. Still, like SAS, JMP comes with a price tag that may limit its use among smaller companies or individual analysts.
Tableau: Beyond Statistical Analysis
Tableau, while primarily a data visualization tool, ensures its place in the arena of data analysis alternatives. It’s acclaimed for transforming complex datasets into intuitive and interactive dashboards. The drag-and-drop interface means even users with minimal technical skills can create insightful representations of their data. However, the tool focuses more on visualization than traditional statistical functions, which may limit its usage for in-depth data analysis compared to others on this list.
"Exploring alternatives to Minitab opens up a world of possibilities, each with unique strengths suitable for different data analysis needs."
Each of these alternatives carries distinct merits that cater to the diverse workflow and financial constraints faced by individuals and organizations alike. From R’s flexibility to Tableau’s focus on visualization, there’s a fit for every data analysis requirement.
Comparative Analysis of Alternatives
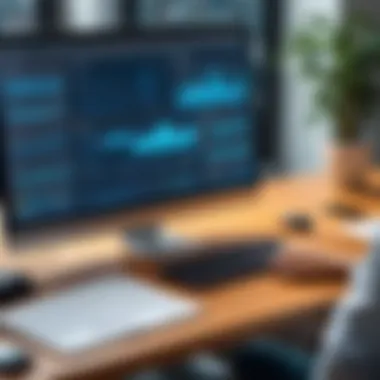
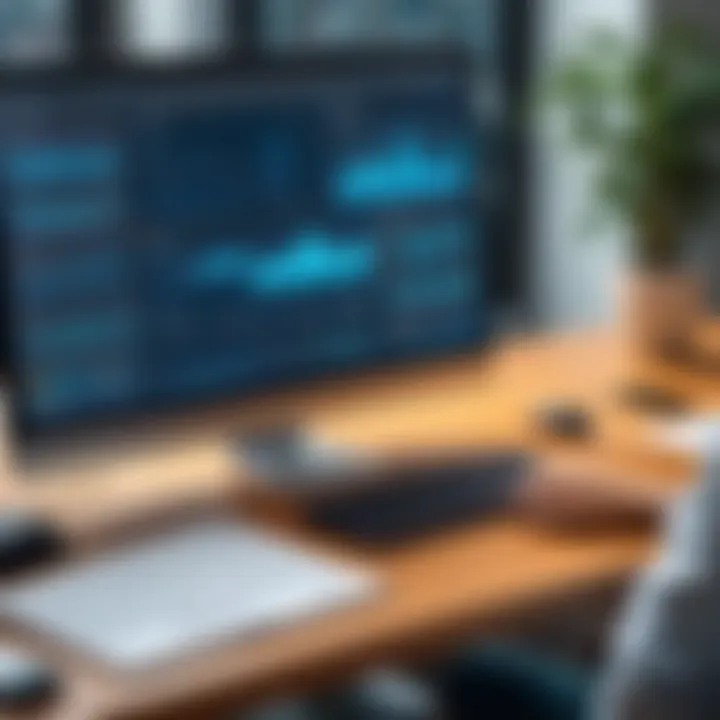
Comparative analysis stands as a cornerstone in evaluating potential replacements for Minitab in statistical software solutions. In the realm of data analysis, simply knowing a tool exists is not enough. You need to truly grasp how it stacks up against competitors in a multitude of aspects. Why? Deciding to transition from a familiar software like Minitab involves weighing benefits against drawbacks, assessing compatibility with existing workflows, and determining the future trajectory of your analytical capabilities.
A well-rounded comparative analysis enables users to scrutinize crucial elements such as feature availability, overall cost effectiveness, and the robustness of community support networks. This critical approach fosters informed decision-making—a necessity not only to optimize costs but also to enhance productivity. As such, understanding these nuances can make the difference between a smooth transition and a tumultuous one.
Feature Comparison
When comparing features among Minitab alternatives, it’s essential to dive beyond surface-level attributes. You might ask: What unique functionalities does each alternative offer? For instance, while R presents extensive libraries for advanced statistical modeling, SPSS excels in user-friendly data manipulation through powerful graphical interfaces.
- R fosters a versatile environment for statistical analysis and data visualization, boasting comprehensive packages for complex algorithms.
- Python, paired with libraries like Pandas and SciPy, allows for custom data manipulation and integration with web applications.
- JMP takes pride in its dynamic data visualization, making it easier to interpret complex datasets at a glance.
Ultimately, evaluating features should also encompass the ease with which users can access and utilize them. Users must determine their own needs—whether advanced modeling is required, or perhaps simpler, exploratory analysis suffices.
Cost Effectiveness Comparison
Cost plays a pivotal role in decision-making, especially for small to mid-sized businesses that need to manage budgets effectively. Minitab is known for being on the pricier side, which leads many to seek out economical alternatives. Here, the cost-effectiveness of software becomes paramount.
- Open-source options, like R and Python, can provide tremendous value, as they eliminate the upfront costs associated with licenses.
- SPSS, while potentially more affordable than Minitab, still requires licensing fees, but may offer tiered pricing that benefits startups and educational institutions.
- SAS is often considered valuable for enterprise-level clients due to its extensive analytical capabilities but comes with a hefty price tag.
Scrutinizing total ownership costs—including maintenance, training, and support—offers a clearer picture of long-term affordability.
User Access and Community Support
In an age where collaborations and shared knowledge are invaluable, the strength of a community becomes an essential factor in the selection of a data analysis tool. With Minitab, you might not have encountered broad user forums or a plethora of online resources. However, alternatives like R and Python thrive on community contributions.
- R hosts an active community eager to share insights via forums, GitHub projects, and even social media platforms like Reddit.
- Tableau, widely used in data visualization, benefits from a vibrant online community that engages in sharing best practices and troubleshooting issues.
- Conversely, some proprietary software like SAS might not have the same openness in community support, leading to a reliance on official resources.
Discovering a platform that not only caters to your immediate needs but also fosters community interaction can significantly enhance learning and support during the transition.
"Choosing the right alternative isn't merely about features or cost; it's about finding a software ecosystem that grows along with your technical prowess."
Best Practices for Transitioning to an Alternative
Transitioning from a well-known software like Minitab to other platforms can be daunting. Understanding best practices for making this switch is essential. Companies and individuals alike must ensure that the alternative chosen aligns perfectly with their specific needs. This section sheds light on crucial practices that can smoothen the journey towards adopting a new data analysis tool.
Assessing Your Needs
Before embarking on the transition, it's key to assess what your actual needs are. Organizations must identify the types of analysis they frequently conduct. Is it basic descriptive statistics, advanced multivariate analyses, or perhaps real-time data visualizations?
Some questions to consider include:
- What are the primary statistical methods used?
- Is collaboration with team members a significant aspect?
- How much training is required before staff can use the new tool effectively?
Understanding this will help narrow down suitable alternatives. For instance, if collaboration is a priority, opting for cloud-based tools may be more beneficial than traditional desktop software. Always remember, tailoring the choice to fit specific requirements can improve productivity and team efficiency in the long-run.
Training and Resources
Once your needs are clear, the next step involves ensuring adequate training for your team. Transitioning only works if users are comfortable with the new system.
Consider utilizing these resources during the transition:
- Online Tutorials: Many platforms offer free resources and video tutorials. Explore sites like YouTube for user-generated content.
- Workshops and Webinars: Attend software-specific training sessions that provide hands-on experience. This can be contacted through vendor websites or online learning platforms.
- Community Forums: Websites like Reddit host discussions where users exchange tips and solutions. Utilizing such communities can be invaluable, especially for troubleshooting.
Organizing regular check-ins to discuss progress and issues may further accelerate the training phase.
Implementation Strategies
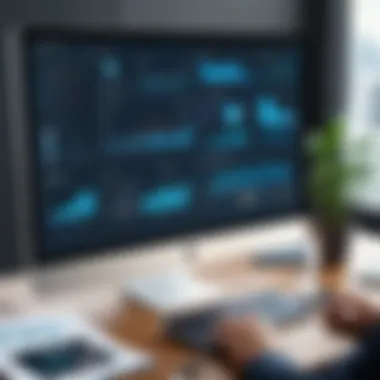
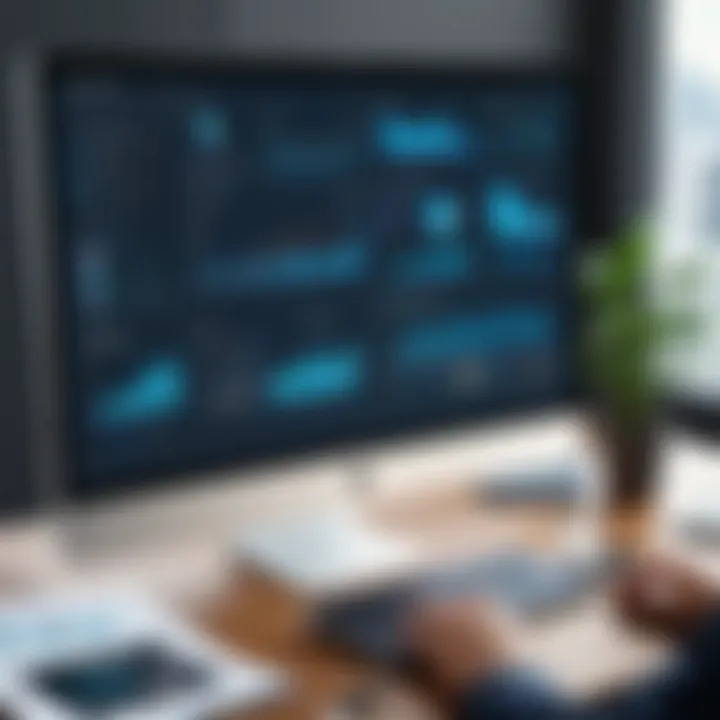
Having a robust strategy for implementation is vital to ensure that the adoption process is smooth and less disruptive.
- Set Clear Goals: Clearly outline what the organization aims to achieve after the switch. For example, a goal might be to reduce data processing time by 20%.
- Phased Rollout: Instead of transitioning all at once, consider a phased approach. Start with a smaller team or department before full-scale implementation.
- Feedback Mechanisms: Create a platform where users can share feedback and experiences. This ongoing dialogue can lead to quick adjustments and improvements, refining how the new tool is used.
- Monitoring Progress: Keep track of performance metrics to gauge the impact of the new tool compared to Minitab. This not only validates the choice made but also highlights areas requiring adjustments.
These considerations will not only maximize the chances of a successful transition but will also foster a culture of adaptability within the organization. Making the leap from Minitab or any other platform is not just about switching software; it's about enhancing overall data analysis capabilities.
Real-World Case Studies
Real-world case studies play a crucial role in guiding professionals to understand how various data analysis tools function in practical environments. They shed light on the pros and cons of different software options, drawing from the experiences of actual users. This can be particularly beneficial for those considering a shift from Minitab to other statistical solutions.
When exploring alternatives, it's essential to see how they perform in real-world situations—this helps professionals determine which tools align with their specific needs and outcomes. These studies provide evidence of functionality, usability, and overall satisfaction, factors that are often overlooked in sales pitches or technical specifications. Moreover, they highlight potential challenges one might face during a transition, offering invaluable insights into not only the success stories but also the hurdles that come with adopting new software.
The following sections delve into specific case studies that illustrate the process of transitioning from Minitab to other powerful data analysis tools.
Transitioning from Minitab to R
Transitioning from Minitab to R can feel like jumping into the deep end for some users, but it often proves to be worthwhile. For example, a mid-sized manufacturing firm previously relied on Minitab for quality control processes. The management decided to make the switch to R, primarily due to its vast range of packages tailored for specific statistical tasks and its open-source nature.
Initially, the learning curve was steep, as R does not have the same user-friendly interface as Minitab. This shift required training sessions for their staff. However, as users became comfortable with R's syntax and the rich ecosystem of libraries it offers, they noticed greater flexibility in their analyses. The firm could easily perform advanced techniques like time series forecasting and machine learning, which were either cumbersome or impossible to implement in Minitab.
In essence, the real benefit came from the ability to customize analyses and share scripts, fostering a culture of collaboration among data scientists and statisticians in the organization.
SPSS Success in Market Research
A notable success story concerning SPSS emerged from a large market research firm that used Minitab for years. When the firm encountered limitations in handling larger datasets, they debated options and gravitated towards SPSS, known for its robust capabilities in handling surveys and sophisticated statistical modeling.
The transition was formidable yet rewarding. The company organized workshops to bring team members up to speed on SPSS's interface and functionalities. They saw immediate improvements in analyzing consumer behavior patterns and preferences, allowing the firm to better tailor marketing strategies for their clients.
What set SPSS apart is its dedicated focus on statistical analysis for social sciences, making it an ideal candidate for this market research firm. Consequently, SPSS became their tool of choice, enabling quicker and more accurate insights into market dynamics.
Using Python for Data Science Projects
In the tech realm, a startup focused on leveraging Python for its data-centric projects found great success after moving away from Minitab. Initially using Minitab for statistical analysis, the team soon recognized Python's potential due to its versatility and extensive libraries dedicated to data manipulation and visualization, like Pandas and Matplotlib.
The startup embarked on a transformation journey that included internal training on Python. This exchange of knowledge fostered a collaborative environment, allowing team members to rapidly test solutions and generate insights from their data. In time, Python not only met their statistical analysis needs but became the backbone for developing machine learning algorithms that propelled their product forward.
By integrating Python into their data workflows, the startup successfully optimized their resources and maximized their analytical capabilities, yielding better predictions and customer insights.
"The shift from Minitab to more versatile tools like R and Python wasn’t just a change of software; it was a transformation in how we envisioned data analysis in our organization."
Through these real-world case studies, one can appreciate the nuanced complexities involved in transitioning away from Minitab, as well as the potential rewards that come with embracing alternatives tailored to specific analytical needs.
Epilogue
In dissecting the landscape of ever-evolving data analysis methods, the importance of selecting the right statistical software cannot be overstated. This article has drawn attention to a range of alternatives to Minitab, each carrying its own strengths and nuances. Understanding these alternatives holds significance for those who find themselves at a crossroads, contemplating the decision to transition away from Minitab due to its limitations or cost implications.
Summarizing the Alternatives
The alternatives explored range from open-source giants like R to enterprise-level solutions such as SAS. Each software package brings something unique to the table. R, for instance, is an open-source powerhouse offering an extensive array of packages for statistical tests and graphical representation, making it particularly appealing for educational and research-oriented applications. On the flip side, SPSS provides a more guided and user-friendly interface, which can be beneficial for professionals who prioritize accessibility over code complexity.
Furthermore, tools like Python with its powerful libraries – Pandas for data manipulation and SciPy for scientific computing – stand out for users keen on employing programming skills in their statistical work. Meanwhile, Tableau transitions beyond traditional statistical analysis, emphasizing interactive data visualization, which can facilitate decision-making in business environments.
Here’s a brief summary of key characteristics of each discussed alternative:
- R: Open-source, vast feature set, strong community support.
- Python with Pandas/SciPy: Versatile, programming-centric, excellent for large datasets.
- SPSS: User-friendly, comprehensive statistical capabilities, common in social sciences.
- SAS: Enterprise-level analytics, strong data management.
- Statgraphics: Extensive statistical options, easy to interpret results.
- JMP: Dynamic and interactive visualizations, great for exploratory data analysis.
- Tableau: Focus on visual analytics, useful for presentations and storytelling with data.
Final Thoughts on Selection Criteria
As IT professionals and businesses sift through these options, several criteria should be at the forefront of their decision-making processes. Usability, for instance, is paramount; the software should not just be powerful but should also accommodate the skill levels of the intended user. Cost is another critical element—ensuring that the software aligns not only with the budget constraints but also provides value proportional to its investment.
The functionality of the software, including features like automation, reporting capabilities, and compatibility with existing data infrastructures, should also guide the selection. Finally, a supportive community can serve as a lifeline when encountering challenges, so evaluating available resources for training and troubleshooting is essential.
Ultimately, transitioning to a new data analysis solution is not merely about selecting an alternative; it is a pathway towards enhancing reliability and optimizing performance in statistical analysis. The right choice paves the way for improved analytics, which can lead to more informed decisions and, consequently, better outcomes for businesses and research alike.