Understanding Signals Analytics for Better Decisions
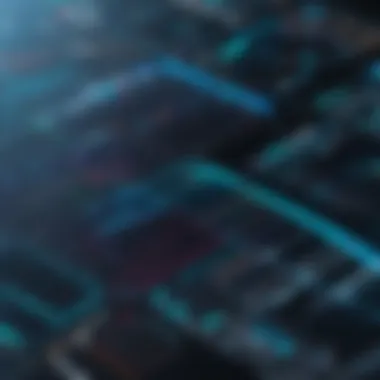
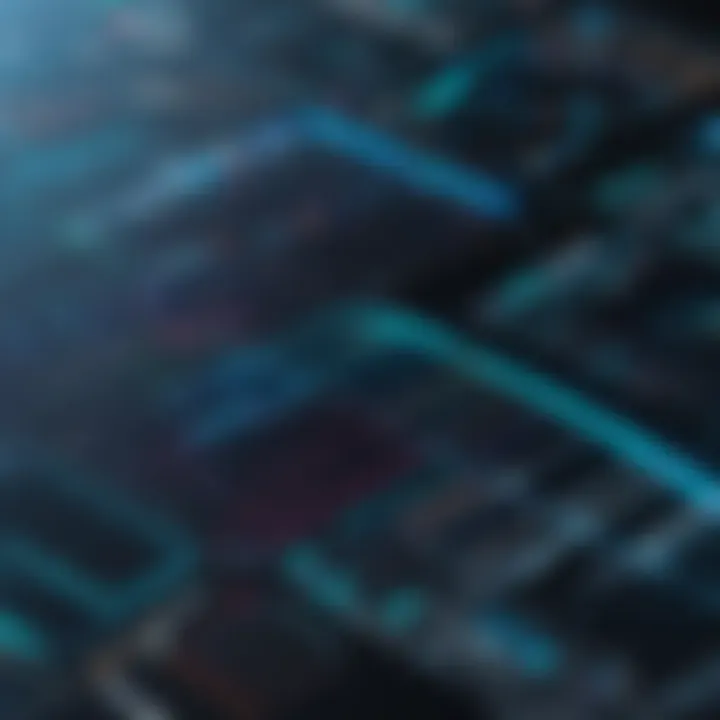
Intro
In today's fast-paced world, organizations are swimming in a sea of data, ranging from customer interactions to market trends. Sifting through this data to find useful information can be overwhelming. This is where signals analytics makes its grand entrance. By examining patterns and extracting meaningful insights from various data streams, signals analytics transforms raw data into a treasure trove of knowledge. This guide will unravel the intricacies of signals analytics, catering to IT professionals, software experts, and businesses of all sizes.
Overview of Software
To understand signals analytics deeply, one must first take a look at how the software operates. While there are numerous platforms out there, a few stand out due to their cutting-edge methodologies and efficiency.
Description of Software
Signals analytics software is designed to harness the power of data. It enables organizations to track signals or indicators that illustrate patterns in data across diverse domains. Whether itโs social media sentiment or purchase behaviors, this software allows for real-time analysis, ensuring businesses can adapt promptly to market changes.
Key Features
- Real-time Data Processing: This enables organizations to access and analyze data instantly, making responses timely and informed.
- Data Visualization: Through charts and dashboards, users can quickly understand trends and insights, helping in decision-making.
- Predictive Analytics: Anticipate future trends based on historical data patterns, giving businesses an edge over competitors.
- User-friendly Interface: The software is designed for users of varying expertise, ensuring seamless accessibility.
Software Comparison
In an ever-evolving tech landscape, comparing various signals analytics tools is essential to stumbling upon the best fit for your organization or business needs. While there are many players in this field, comparing functionalities can guide you toward making an informed choice.
Comparison with Similar Software
Some notable software tools in the domain include Tableau, QlikSense, and Google Data Studio. Tableau excels in data visualization but might require a steeper learning curve. QlikSense offers great associative data modeling capabilities but may not be as intuitive for beginners. In contrast, Google Data Studio provides an easy entry point but might fall short on advanced functionalities.
Advantages and Disadvantages
- Tableau
- QlikSense
- Google Data Studio
- Advantages: Exceptional visualization tools.
- Disadvantages: Higher costs and a need for stronger technical skills.
- Advantages: Powerful data integration.
- Disadvantages: Complexity can be daunting for new users.
- Advantages: Free and easy to use.
- Disadvantages: Limited advanced analytics features.
In summary, each software tool has its own set of strengths and weaknesses. Businesses must consider their specific needs, budget, and the technological expertise of their teams when making a choice.
"The beauty of signals analytics lies in its ability to turn complexity into clarity, offering businesses a roadmap where previously there was just noise."
By understanding these software dynamics, organizations can better navigate their choices to leverage signals analytics to its full potential.
Prelims to Signals Analytics
Signals analytics has emerged as a cornerstone in making well-informed decisions across various sectors. Its significance lies in the ability to sift through vast swathes of data to extract actionable insights. In todayโs fast-paced landscape, organizations that can effectively harness signals analytics often outpace their competitors by making data-driven strategies rather than relying solely on gut feelings. This capability enables firms to respond swiftly to market changes, customer behaviors, and operational challenges.
Defining Signals Analytics
Signals analytics can be understood as the systematic process of interpreting data signals, which often stem from quantitative and qualitative sources. This field emphasizes not just the collection of data but also the discerning interpretation of that data to understand underlying patterns and trends. Think of it as tuning into a radio frequency; the clearer the signal, the better the reception.
To get into the nuts and bolts, signals analytics encompasses several techniques and tools that facilitate this process, ranging from simple descriptive statistics to more complex predictive models that foretell future trends. Organizations apply these methods to figure out everything from consumer preferences to operational efficiencies. For instance, a retail chain might use signals analytics to analyze foot traffic data to gain insights into sales patterns, helping them position products more strategically in stores.
The Importance of Signals in Data Analysis
Understanding the role of signals in data analysis cannot be overstated. Signals serve as a bridge, connecting raw data to meaningful insights, enabling organizations to see the forest for the trees. By identifying which data points are significant, organizations can prioritize what truly matters, thus leading to more timely and effective decision-making.
The implications of this process are vast. For one, signals can forecast market trends, providing companies with a competitive edge. Additionally, they can enhance customer experiences by tailoring services and products based on real-time insights.
Moreover, as data privacy regulations tighten, relying on well-defined signals rather than raw and possibly sensitive data can mitigate risks associated with compliance. Effective signals analytics is not just about crunching numbers but about the stories those numbers tell.
"In data, the signal is the insight, while the noise is everything else. A clear signal can guide decision-making like a lighthouse guiding ships to safety."
Thus, organizations equipped with signals analytics capabilities can maneuver through unpredictability with a clearer sense of direction. They are better positioned to identify risks and seize opportunities, paving the way for sustainable growth and innovation.
Core Components of Signals Analytics
In today's fast-paced world, understanding signals analytics is essential for businesses and organizations aiming to thrive. The core components of signals analytics are vital in shaping the way data is gathered, processed, and leveraged for informed decision-making. Each aspect plays a critical role in how signals analytics is conducted, ensuring that decisions are not made in a vacuum, but rather based on concrete insights derived from solid methodologies.
In this section, we will delve into the fundamental data sources involved in gathering information and the tools and technologies that facilitate effective analysis of that data. Understanding these elements can significantly enhance an organizationโs ability to discern patterns and forecast outcomes, making the implementation of signals analytics both a strategic advantage and a necessity.
Data Sources and Information Gathering
Data sources are the backbone of signals analytics. They encompass a wide range of information that feeds into analytical models, allowing organizations to derive meaningful insights.
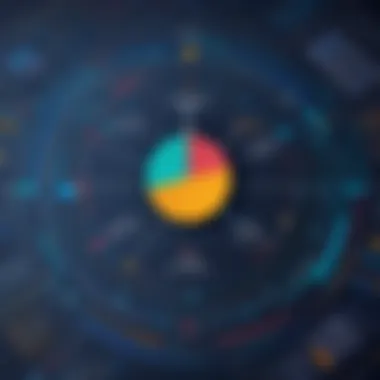
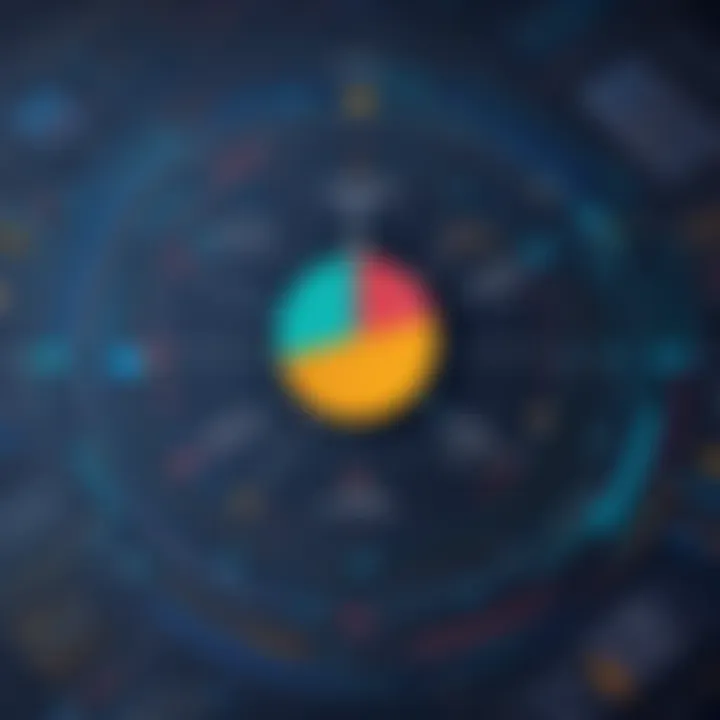
Quantitative Data
Focusing on numbers, quantitative data is all about hard facts and figures. This type of data is primarily statistical and can be analyzed using various mathematical methods. In the context of signals analytics, quantitative data is invaluable because it provides a clear, measurable foundation for analysis. It allows analysts to identify trends, compute averages, and forecast potential outcomes with a degree of accuracy thatโs hard to achieve with other forms of data.
- Key Characteristic: Itโs numeric, objective, and often analyzed through statistical software.
- Why Beneficial: This type of data helps in establishing concrete metrics.
- Unique Feature: Amplifies findings through rigorous testing and verification. However, it might miss the nuances behind the numbers that qualitative data can provide.
Qualitative Data
On the flip side, qualitative data brings forth the story behind the numbers. It delves into perceptions, motivations, and feelings, forming a narrative that's often indispensable in understanding customer behaviors or market trends. For an organization, integrating qualitative data adds depth to the analytics process, allowing for decisions based on comprehensive insights rather than sheer numbers alone.
- Key Characteristic: Subjective and often gathered through interviews, surveys, or observations.
- Why Beneficial: Rich in context, it sheds light on why certain trends appear in quantitative data.
- Unique Feature: Captures complex emotions and thoughts but lacks the straightforwardness of numbers.
Third-Party Integrations
Leveraging third-party integrations is another core aspect of signals analytics. By connecting various data sources, organizations can create a more holistic view of their datasets. This component is crucial for businesses that operate in a data-rich environment, allowing them to unite disparate streams of information into a coherent analytic framework.
- Key Characteristic: It combines data from several external systems, enriching the dataset.
- Why Beneficial: Provides diverse insights and promotes a more comprehensive analysis.
- Unique Feature: Enables organizations to capitalize on existing technologies and datasets without reinventing the wheel, although it can introduce complexities regarding compatibility and data management.
Tools and Technologies Involved
The right tools and technologies play a fundamental role in the effective application of signals analytics. They enable the seamless processing and interpretation of data, essentially translating numbers into actionable insights.
Data Processing Tools
Data processing tools are specialized software that help in cleaning, organizing, and analyzing data. In relation to signals analytics, these tools are essential because they streamline workflows and ensure that data insights are relevant and accurate.
- Key Characteristic: Automates tedious tasks related to data entry and processing.
- Why Beneficial: Enhances accuracy and efficiency, allowing analysts to focus on interpretation rather than data cleaning.
- Unique Feature: Real-time processing capabilities can facilitate immediate decision-making, yet they might require initial setup costs and training.
Visualization Techniques
Visualization techniques translate complex datasets into intuitive graphics, making it easier for stakeholders to grasp insights at a glance. In the realm of signals analytics, this is particularly important as it fosters a more engaging presentation of data findings.
- Key Characteristic: Converts data points into visual formats such as graphs, charts, and dashboards.
- Why Beneficial: Supports better understanding through visual storytelling and clearer communication of data trends.
- Unique Feature: Can uncover patterns that may go unnoticed in raw data. Nonetheless, care must be taken to ensure visuals are not misleading.
Machine Learning Applications
At the forefront of innovation in signals analytics are machine learning applications. These advanced technologies can analyze vast quantities of data, discerning patterns and making predictions with a degree of sophistication that manual analysis lacks.
- Key Characteristic: Utilizes algorithms that learn from data inputs.
- Why Beneficial: Automates predictive analytics, enhancing decision-making ability.
- Unique Feature: Adaptability to new data makes it a powerful forecasting tool; however, reliance on them can sometimes lead to opaqueness in decision processes due to complexity.
As organizations harness signals analytics, understanding the core componentsโfrom data sources to the technologies involvedโbecomes crucial for fostering informed decision making across all levels.
Techniques in Signals Analytics
In the realm of signals analytics, the techniques employed serve as the backbone for extracting meaningful insights from data. Understanding the various methods not only enhances analytical capabilities but also equips organizations to make informed, data-driven decisions. By breaking down these vital techniques, businesses can tailor their approaches to suit specific needs and contexts, leveraging data to its utmost potential.
Descriptive Analysis
Descriptive analysis is like peering into a mirror; it reflects what has happened by summarizing past data. This technique helps businesses identify trends, patterns, and anomalies within existing data sets. For instance, a retail company could utilize this method to analyze sales data over the last year, highlighting peak seasons and identifying top-selling products.
Focusing on metrics such as
- Mean,
- Median, and
- Frequency distributions
allows firms to craft a clear picture of whatโs going on within their operations.
Moreover, visuals like bar charts or pie graphs can reinforce these insights. Itโs one thing to say that sales have increased, and another to visually represent that growth to stakeholders during presentations. This can be accompanied by insights on customer behavior, such as frequent purchases or feedback patterns, providing a solid foundation for deeper analysis.
Predictive Analytics
Now, if descriptive analysis is telling the story of the past, predictive analytics takes the bold step of forecasting future outcomes. Relying heavily on historical data, machine learning algorithms, and statistical methods, predictive analytics allows organizations to anticipate trends before they even hit.
Consider a financial institution using predictive models to assess credit risk. By analyzing past borrower behaviors and current economic conditions, they can identify potential risks and adjust their lending strategies accordingly. Similarly, in healthcare, predictive analytics can model patient readmission rates, allowing doctors to intervene proactively.
Some common techniques used include:
- Regression Analysis - Checks relationships between variables.
- Time-Series Forecasting - Analyzes data points collected at intervals over time.
- Classification Algorithms - Predicts category membership based on input data.
This proactive approach can protect against pitfalls and capitalize on opportunities, paving the way for sustainable growth.
Prescriptive Analytics
Where predictive analytics stops at forecasting, prescriptive analytics goes one step further by recommending actions based on those forecasts. Itโs about not just knowing what might happen, but guiding organizations on what to do about it.
For instance, in a factory setting, prescriptive analytics can assess the impact of maintenance schedules on production efficiency. Using decision models, it can suggest the optimal timing for equipment checks to minimize downtime while maximizing output. This method employs techniques from operations research, simulations, and optimization studies to guide critical decisions.
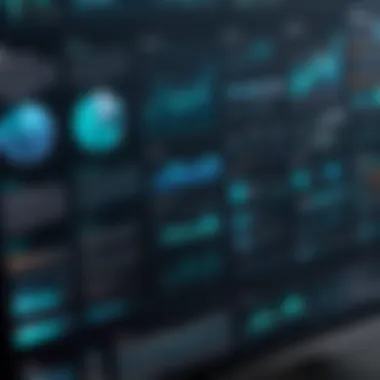
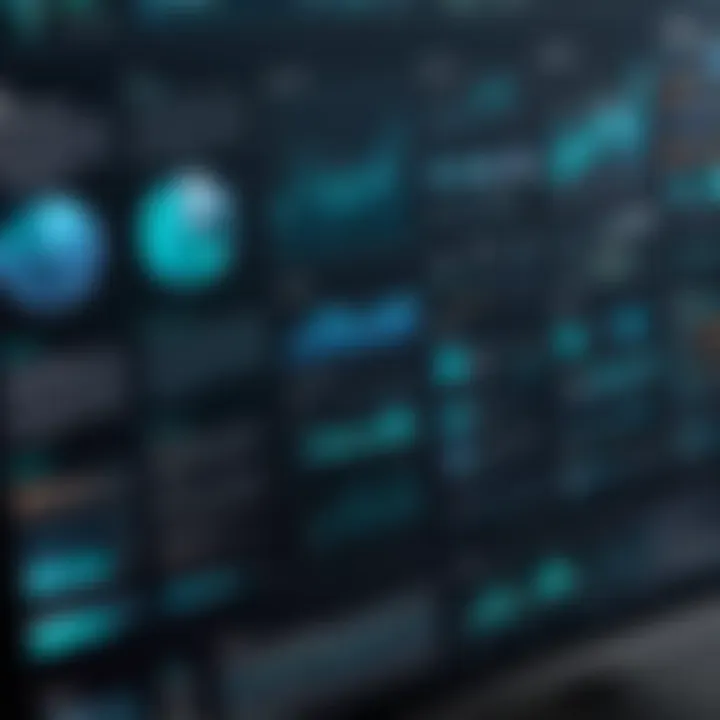
Advantages of prescriptive analytics include:
- Actionable Recommendations: Rather than guesses, businesses get specific strategies.
- Resource Optimization: Helps utilize resources more efficiently, reducing waste.
- Risk Management: Aids in evaluating the consequences of various choices, allowing for informed risk-taking.
With these techniques, organizations can not only adapt to new challenges but also thrive, fostering a culture of proactive decision-making that bases its choices on solid data.
"Data is like a precious artifact; it only holds value when it's thoughtfully analyzed and applied across decision-making processes."
Each technique in signals analytics is crucial to building a comprehensive framework that enhances data-informed decision-making. By weaving descriptive, predictive, and prescriptive analytics into their strategies, organizations can navigate the complexities of modern business environments with confidence and acumen.
Applications of Signals Analytics
Signals analytics serves as a lens guiding organizations through complex data landscapes. By translating noise into actionable insights, it empowers decision-makers across various sectors. Its implications extend well beyond theoretical applications, offering tangible benefits like enhanced operational strategies, improved customer experiences, and optimized resource allocation. As markets become more competitive, employing signals analytics enables businesses to maintain an edge by discerning patterns and trends that would otherwise go unnoticed.
Business Decision-Making
In the sphere of business decision-making, signals analytics becomes an invaluable ally. It processes vast amounts of data to unearth insights that can inform strategy and operational adjustments.
Market Analysis
Market analysis encapsulates the investigation of multiple market indicators such as trends, pricing, and competitor behavior. This specific aspect of signals analytics enables businesses to determine where they stand in the landscape and where they need to pivot. By understanding customer preferences and market dynamics through data, organizations can make informed decisions related to product development or promotional tactics. Its characteristic is the ability to provide real-time data, which many firms find beneficial for timely responses. A distinctive feature is the incorporation of competitor intelligence; it can highlight unexpected trends or shifts in consumer behavior before they become mainstream, giving firms a head start. However, it does have its drawbacks, such as the potential for data overload, which may lead to paralysis by analysis if not managed well.
Customer Insights
Customer insights represent the understanding derived from analyzing customer behavior, preferences, and feedback. In shaping product lines and developing marketing strategies, this aspect plays a critical role. Capturing specific characteristics of buying patterns can reveal hidden opportunities for expansion or identify gaps in customer satisfaction. Its popularity stems from its focus on the voice of the customer, enabling businesses to tailor offerings effectively. Moreover, unique analytics can capture sentiment analysis, providing a quantitative measure of customer satisfaction. Yet, risks include overreliance on data patterns without acknowledging qualitative factors or sudden market shifts that don't fit historical trends, which may mislead decision-making.
Operational Efficiency
Operational efficiency focuses on optimizing internal processes through data-driven insights. By analyzing workflow and resource usage, organizations can identify bottlenecks or redundancies that hinder productivity. This focus aids in the continuous improvement of operations, making it a popular choice in today's fast-paced environment. Its key trait is the capacity to automate routine tasks, freeing up human resources for more complex responsibilities. On the flip side, reliance on technology may inadvertently lead to neglecting human oversight, potentially causing oversights in nuanced scenarios that require a human touch.
Healthcare Analytics
In healthcare, signals analytics has emerged as a game-changer. The ability to optimize patient care, resource allocation, and overall healthcare strategies through data analysis makes it indispensable in this field.
Patient Monitoring
Patient monitoring employs real-time data from various sources, such as wearable devices and EMRs, to track patient health and predict complications. This real-time characteristic is essential for timely interventions that can change outcomes dramatically. The continual stream of data presents unique advantages, allowing for quick responses to declining health indicators. However, the potential disadvantage lies in privacy concerns; managing vast amounts of sensitive patient data highlights the need for stringent data protection measures to safeguard personal health information.
Resource Management
Resource management within healthcare analyzes data related to staffing, equipment availability, and facility use. The essential aspect of this analysis is its contribution to cost reduction and improved service delivery. By understanding workflow patterns, healthcare providers can allocate resources more effectively, leading to enhanced patient satisfaction. The key characteristic here is its adaptabilityโanalyzing fluctuating demand or unexpected surges in patient load can streamline operations efficiently. A notable caution, however, includes the unpredictability of healthcare demands, which may render historical data less reliable during crisis situations, such as pandemics.
Predictive Health Models
Predictive health models leverage historical data to forecast future health trends and patient outcomes. This aspect of signals analytics plays a vital role in preventive care, enabling early intervention for at-risk patients. The key characteristic is its forward-looking nature, which allows healthcare providers to make data-driven decisions around resource allocation and patient care strategies. This unique predictive analytics capability enhances workflow and care practices but may lead to dependency on predictive metrics, sometimes overshadowing the immediate and changing needs of individual patients.
Financial Forecasting
Ultimately, signals analytics holds significant promise in financial forecasting, informing investment strategies, risk management, and fraud detection. Its application here is crucial, particularly in an environment increasingly shaped by volatility and rapid change.
Investment Strategies
Investment strategies in financial forecasting hinge on analyzing market signals to guide investment decisions. Its specific aspect involves interpreting fluctuations in stock prices, interest rates, and economic indicators. The characteristic of timely data processing heightens its value in making decisions that align with market conditions. A key feature is portfolio optimization, balancing risks and returns based on predictive insights. Yet, the inherent volatility of markets can pose challenges; substantial reliance on analytics might lead individuals to neglect critical qualitative elements that affect market behavior.
Risk Management
Risk management entails identifying potential risks that could adversely affect an organization. This specific aspect is fundamental, especially in a landscape fraught with economic uncertainties. By analyzing historical data and modeling potential risks, organizations can strategize better to mitigate negative impacts. Its key characteristic lies in developing a proactive rather than reactive approach to risk. A unique feature is the use of scenario analysis, which helps uncover potential vulnerabilities for informed decision-making. One challenge, however, is maintaining accuracy in predictive models amidst changing regulatory or market conditions, which can skew forecasts.
Fraud Detection
Fraud detection serves as a critical function within financial forecasting, utilizing signals analytics to identify irregularities or patterns indicative of fraudulent behavior. Its importance lies in safeguarding assets and maintaining trust in financial transactions. The primary characteristic is real-time monitoring, enabling swift responses to suspicious activities. A unique feature is the integration of machine learning algorithms, escalating detection rates by continuously learning from new data patterns. Yet, it may also present challenges, including potential false positives that could harm legitimate customers and lead to reputational damage if not managed carefully.
Challenges in Signals Analytics
In the realm of signals analytics, challenges can arise that may impede the effective utilization of data for informed decision-making. These hurdles are not merely obstacles but essential factors to understand for anyone looking to successfully navigate the complex landscape of data analytics. The nature of data is ever-changing, and as more signals are produced, organizations must address these challenges to maintain accuracy and reliability. Remarkably, overcoming these challenges not only enhances data utilization but also contributes to a more transparent and secure process.
Data Privacy and Security
Data privacy and security are pivotal elements when analyzing signals. With the exponential growth of data collection, organizations find themselves juggling a minefield of regulations and best practices. The need to ensure personal data is handled with care cannot be overstated. There's no point in having cutting-edge analytics capabilities if the data at your disposal is unsecured.
"Data is the new oil, but if not managed properly, it can leak like a rusty faucet."
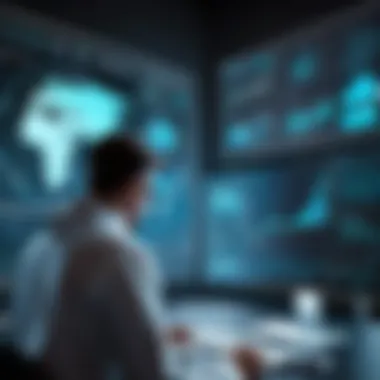
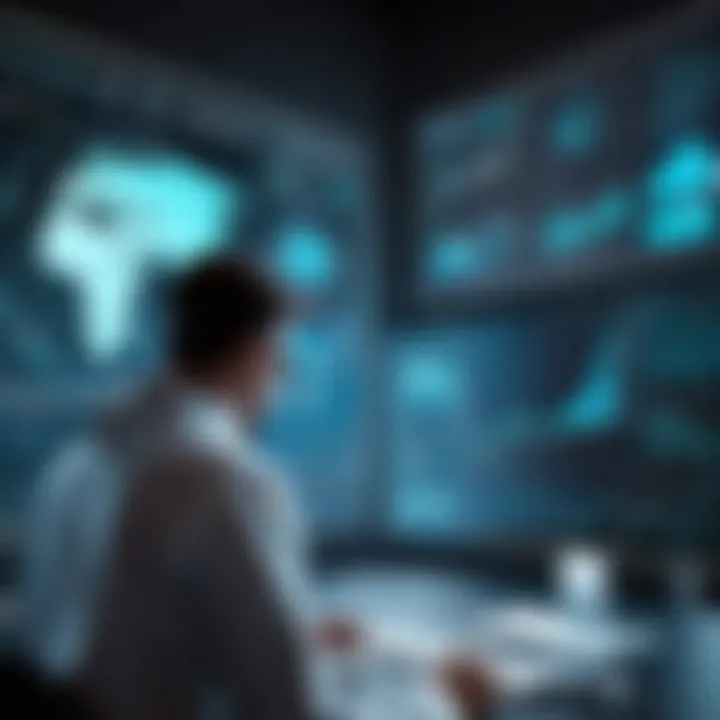
Here are some key considerations regarding data privacy and security:
- GDPR Compliance: The General Data Protection Regulation has raised the bar for organizations handling EU residents' data. Ensure that youโre compliant, or risk hefty fines.
- Data Encryption: This is not just good practice; it's essential. Encrypting data both at rest and in transit safeguards it against unauthorized access.
- Access Control: Limiting the number of individuals who have access to sensitive data is crucial. This creates a culture of accountability.
Organizations should also be prepared for potential breaches by implementing incident response plans that outline swift and effective actions.
Integration of Diverse Data Sources
The integration of diverse data sources presents both opportunities and challenges in signals analytics. In todayโs world, organizations collect data from a plethora of channels, including social media, IoT devices, and customer databases. However, bringing such a diverse set of data together can be a daunting task.
Complications can arise from differences in format, quality, and structure of the data. When these data strands are woven together effectively, they can form a rich tapestry of insight. But without proper integration, organizations may miss out on pivotal information.
Some hurdles organizations face:
- Data Silos: These occur when different departments do not share their data. This can lead to discrepancies in understanding customer needs.
- Quality of Data: Merging high-quality data with outdated or inaccurate data can poison the well, muddying insights.
- Technical Complexity: Establishing systems that effectively integrate various data sources requires significant technical investment.
To tackle these challenges, organizations might explore Data Integration Platforms that specialize in combining heterogeneous data sources into a unified framework.
Algorithm Bias and Transparency
Another significant challenge in signals analytics is algorithm bias and the need for transparency. Algorithms can inadvertently reinforce existing biases present in training data, leading to skewed outcomes. This matter resonates deeply in sectors like recruitment, credit assessment, and even healthcare.
- Recognizing Bias: First and foremost, organizations must learn to recognize and understand the biases that may be inherent in their data.
- Transparency in Algorithms: Stakeholders should have access to how algorithms make decisions. This transparency fosters trust and allows for scrutiny where needed.
- Regular Audits: Conducting periodic audits of algorithms can help identify and mitigate biases proactively.
In summary, while the challenges of signals analytics can seem formidable, they are not insurmountable. Addressing these issues head-on can lead to a more robust and effective analytics framework that not only promotes informed decision-making but also fosters trust and integrity in data handling.
Future Trends in Signals Analytics
Understanding future trends in signals analytics is pivotal for organizations seeking to remain competitive in an ever-evolving landscape. As technology progresses, the ways in which data can be leveraged will change, presenting both opportunities and challenges. Recognizing these emerging patterns helps businesses make informed decisions and proactively adapt their strategies.
Advancements in Artificial Intelligence
The advancements in artificial intelligence are set to transform signals analytics significantly. AI tools and algorithms can sift through large volumes of data swiftly, unearthing patterns that human analysts might overlook. This ability not only enhances efficiency but also increases the accuracy of insights.
With natural language processing, for instance, organizations can analyze customer feedback in real-time. AI can swiftly categorize sentiments, highlighting potential customer dissatisfaction or emerging trends in preferences. Furthermore, machine learning models are increasingly employed to refine predictions based on historical data, allowing businesses to adjust their strategies dynamically.
The prospect of AI-driven analytics is exciting but also warrants caution. Companies must prioritize transparency and ensure their AI models are free from bias and inaccuracies.
The Role of Big Data
In the context of big data, signals analytics finds its true form. The sheer volume of data generated daily, be it from social media, IoT devices, or transactional systems, offers a goldmine of insights. However, not all data is created equal. Discerning the signals from the noise is where signals analytics shines.
Advantages of harnessing big data include:
- Enhanced personalization in services and products
- Improved operational efficiencies
- Proactive identification of emerging market trends
Organizations that can adeptly analyze and interpret vast data sets will likely gain a competitive edge. Yet, this demands robust infrastructure and skilled professionals who can navigate and exploit these complex data landscapes effectively.
Evolving Analytical Frameworks
The evolving analytical frameworks are crucial for adapting to the changing data environment. Traditional methods may not suffice when addressing the multifaceted nature of current data streams. Modern frameworks emphasize agility and adaptability, incorporating real-time processing, predictive modeling, and advanced visualizations to support decision-making.
Key considerations for organizations include:
- Integration: Ensuring seamless collaboration between different analytics tools and platforms.
- User-friendly Interfaces: Facilitating easier access and interpretation of data for non-technical users.
- Continuous Learning: Encouraging systems that update themselves based on new data.
These evolving frameworks provide companies with robust capabilities to analyze signals efficiently, leading to more informed decision-making.
"The ability to adapt to changing data patterns distinguishes successful organizations in today's digital landscape."
With each of these trends, organizations can better position themselves not only to harness insights but also to forecast and prepare for future challenges. Signals analytics is becoming an integral tool for those aiming to thrive in this data-driven world.
Culmination
Wrapping our discussion around signals analytics, itโs clear that this field holds immense potential for informed decision-making. Throughout this article, we have peeled back the layers of what signals analytics entails, from foundational definitions to its multifaceted applications in various sectors.
Summary of Key Insights
In essence, signals analytics is more than just a collection of data points. Itโs about establishing a cohesive narrative out of raw data, a story that organizations can leverage to navigate market dynamics and operational challenges. Here are some prominent takeaways:
- Integration of Diverse Data: Signals analytics thrives on data from various sourcesโquantitative measurements combined with qualitative insights create a richer context for decision-making.
- Significant Applications Across Sectors: Whether in business, healthcare, or finance, the tools derived from signals analytics convert numbers into actionable strategies. For instance, predictive analytics aids in anticipating market trends, while descriptive analytics illuminates past performances.
- Challenges Must Be Addressed: Issues surrounding data privacy, algorithm bias, and the integration of diverse data sources present hurdles that must be tackled head-on. Acknowledging these challenges is as crucial as leveraging the data.
"Data is a precious thing and will last longer than the systems themselves." โ Tim Berners-Lee
Final Thoughts on Signals Analytics
Looking toward the future, signals analytics represents a vital cog in the wheel of organizational success. As technology evolves and the landscape of big data expands, so too will the mechanisms for filtering and analyzing signals.
Focusing on clear metrics, ethical considerations, and innovative tools will allow organizations to refine their strategies continually. Keeping an ear to the ground for emerging trends and adapting will empower firms, regardless of size, to stay ahead in an increasingly competitive environment.